Instant-3D: Instant Neural Radiance Field Training Towards On-Device AR/VR 3D Reconstruction
CoRR(2023)
摘要
Neural Radiance Field (NeRF) based 3D reconstruction is highly desirable for immersive Augmented and Virtual Reality (AR/VR) applications, but achieving instant (i.e., < 5 seconds) on-device NeRF training remains a challenge. In this work, we first identify the inefficiency bottleneck: the need to interpolate NeRF embeddings up to 200,000 times from a 3D embedding grid during each training iteration. To alleviate this, we propose Instant-3D, an algorithm-hardware co-design acceleration framework that achieves instant on-device NeRF training. Our algorithm decomposes the embedding grid representation in terms of color and density, enabling computational redundancy to be squeezed out by adopting different (1) grid sizes and (2) update frequencies for the color and density branches. Our hardware accelerator further reduces the dominant memory accesses for embedding grid interpolation by (1) mapping multiple nearby points' memory read requests into one during the feed-forward process, (2) merging embedding grid updates from the same sliding time window during back-propagation, and (3) fusing different computation cores to support the different grid sizes needed by the color and density branches of Instant-3D algorithm. Extensive experiments validate the effectiveness of Instant3D, achieving a large training time reduction of 41x - 248x while maintaining the same reconstruction quality. Excitingly, Instant3D has enabled instant 3D reconstruction for AR/VR, requiring a reconstruction time of only 1.6 seconds per scene and meeting the AR/VR power consumption constraint of 1.9 W.
更多查看译文
关键词
Neural Radiance Field (NeRF),Hardware Accelerator
AI 理解论文
溯源树
样例
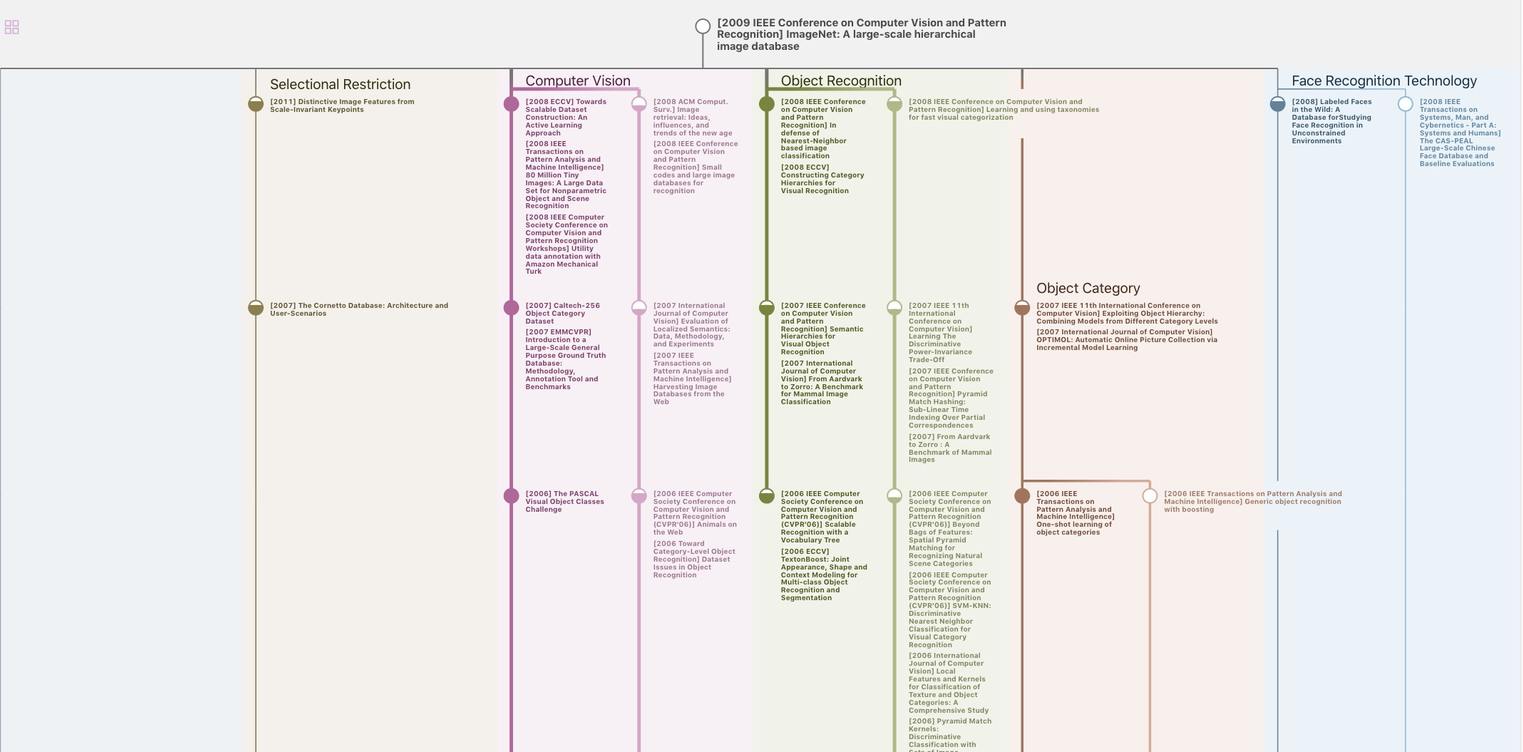
生成溯源树,研究论文发展脉络
Chat Paper
正在生成论文摘要