Unsupervised Style-based Explicit 3D Face Reconstruction from Single Image
CoRR(2023)
摘要
Inferring 3D object structures from a single image is an ill-posed task due to depth ambiguity and occlusion. Typical resolutions in the literature include leveraging 2D or 3D ground truth for supervised learning, as well as imposing hand-crafted symmetry priors or using an implicit representation to hallucinate novel viewpoints for unsupervised methods. In this work, we propose a general adversarial learning framework for solving Unsupervised 2D to Explicit 3D Style Transfer (UE3DST). Specifically, we merge two architectures: the unsupervised explicit 3D reconstruction network of Wu et al. and the Generative Adversarial Network (GAN) named StarGAN-v2. We experiment across three facial datasets (Basel Face Model, 3DFAW and CelebA-HQ) and show that our solution is able to outperform well established solutions such as DepthNet in 3D reconstruction and Pix2NeRF in conditional style transfer, while we also justify the individual contributions of our model components via ablation. In contrast to the aforementioned baselines, our scheme produces features for explicit 3D rendering, which can be manipulated and utilized in downstream tasks.
更多查看译文
关键词
3D ground truth,Basel Face Model,conditional style transfer,depth ambiguity,Explicit 3D Face reconstruction,explicit 3D rendering,Explicit 3D Style Transfer,general adversarial learning framework,Generative Adversarial Network named StarGAN-v,hand-crafted symmetry priors,implicit representation,inferring 3D object structures,occlusion,Pix2NeRF,single image,supervised learning,typical resolutions,UE3DST,Unsupervised 2D,unsupervised explicit 3D reconstruction network,unsupervised methods
AI 理解论文
溯源树
样例
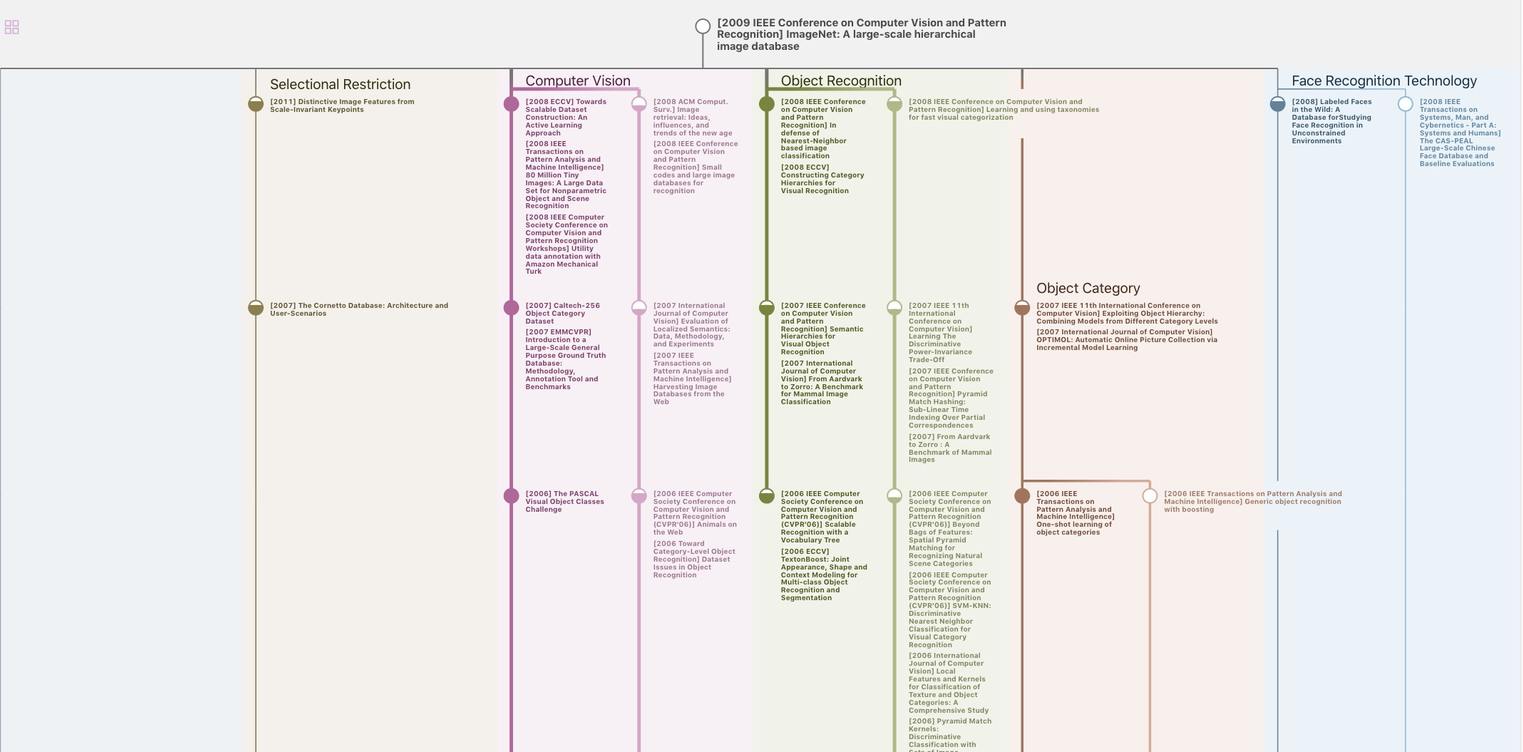
生成溯源树,研究论文发展脉络
Chat Paper
正在生成论文摘要