Active Learning Strategies Based on Text Informativeness
2022 IEEE/WIC/ACM International Joint Conference on Web Intelligence and Intelligent Agent Technology (WI-IAT)(2022)
摘要
In this paper, we propose strategies for selecting the next item to label in active learning for text data. Text data have several text-specific features, such as TF-IDF vectors and document embeddings. These features have correlation with the informativeness of the text data, so our methods select the next item to label by using these text-specific features. We evaluate the performance of our strategies in two problem settings: the standard active learning setting, where we focus on the improvement of the model accuracy, and the learning-to-enumerate setting, where we focus on the efficiency in enumerating all instances of a given target class. We also combine our strategies with two existing strategies: uncertainty sampling, a well-known strategy for active learning, and the exploitation-only strategy, a strategy used in learning-to-enumerate problems. Our experiment on two publicly available English text datasets show that our method outperforms the baseline methods in both problem settings.
更多查看译文
关键词
active learning,learning to enumerate,informativeness,TF-IDF,word embedding,uncertainty sampling
AI 理解论文
溯源树
样例
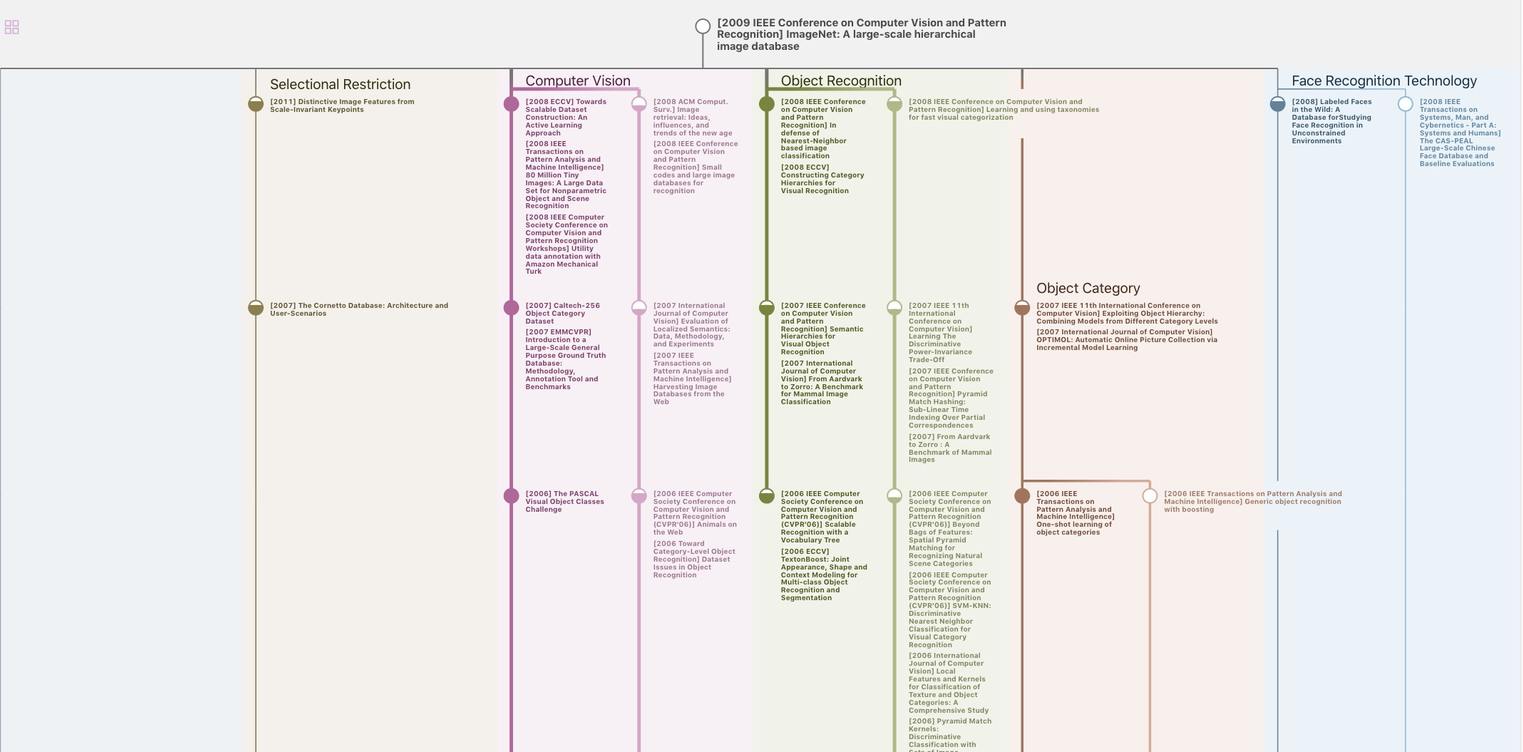
生成溯源树,研究论文发展脉络
Chat Paper
正在生成论文摘要