Kriging, Polynomial Chaos Expansion, and Low-Rank Approximations in Material Science and Big Data Analytics.
Big data(2023)
摘要
In material science and engineering, the estimation of material properties and their failure modes is associated with physical experiments followed by modeling and optimization. However, proper optimization is challenging and computationally expensive. The main reason is the highly nonlinear behavior of brittle materials such as concrete. In this study, the application of surrogate models to predict the mechanical characteristics of concrete is investigated. Specifically, meta-models such as polynomial chaos expansion, Kriging, and canonical low-rank approximation are used for predicting the compressive strength of two different types of concrete (collected from experimental data in the literature). Various assumptions in surrogate models are examined, and the accuracy of each one is evaluated for the problem at hand. Finally, the optimal solution is provided. This study paves the road for other applications of surrogate models in material science and engineering.
更多查看译文
关键词
concrete,polynomial chaos expansion,regression,surrogate model
AI 理解论文
溯源树
样例
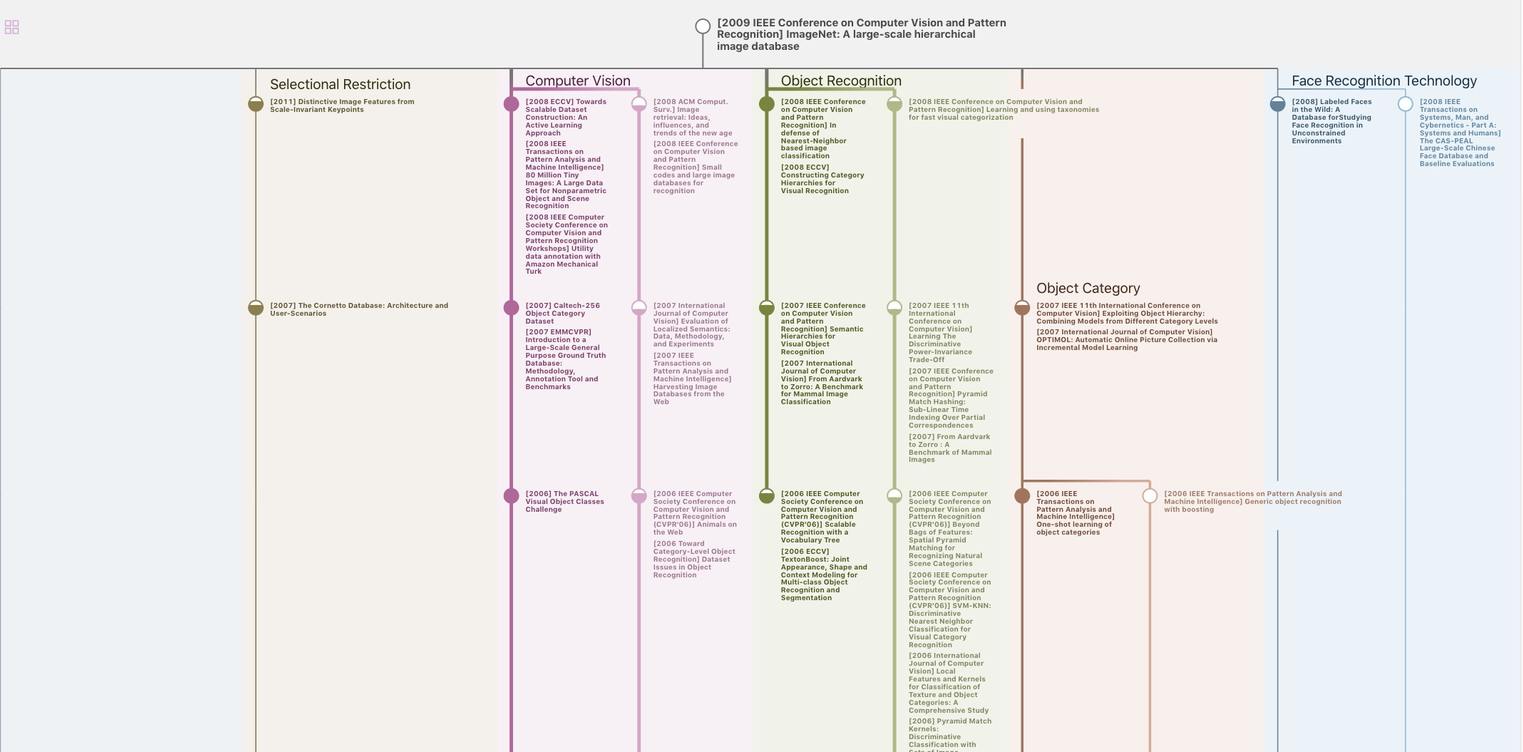
生成溯源树,研究论文发展脉络
Chat Paper
正在生成论文摘要