Modified you-only-look-once model for joint source detection and azimuth estimation in a multi-interfering underwater acoustic environment.
The Journal of the Acoustical Society of America(2023)
摘要
The you-only-look-once (YOLO) model identifies objects in complex images by framing detection as a regression problem with spatially separated boundaries and class probabilities. Object detection from complex images is somewhat similar to underwater source detection from acoustic data, e.g., time-frequency distributions. Herein, YOLO is modified for joint source detection and azimuth estimation in a multi-interfering underwater acoustic environment. The modified you-only-look-once (M-YOLO) input is a frequency-beam domain (FBD) sample containing the target and multi-interfering spectra at different azimuths, generated from the received data of a towed horizontal line array. M-YOLO processes the whole FBD sample using a single-regression neural network and directly outputs the target-existence probability and spectrum azimuth. Model performance is assessed on both simulated and at-sea data. Simulation results reveal the strong robustness of M-YOLO toward different signal-to-noise ratios and mismatched ocean environments. As tested on the data collected in an actual multi-interfering environment, M-YOLO achieved near-100% target detection and a root mean square error of 0.54° in azimuth estimation.
更多查看译文
关键词
underwater acoustic environment,joint source detection,azimuth estimation,you-only-look-once,multi-interfering
AI 理解论文
溯源树
样例
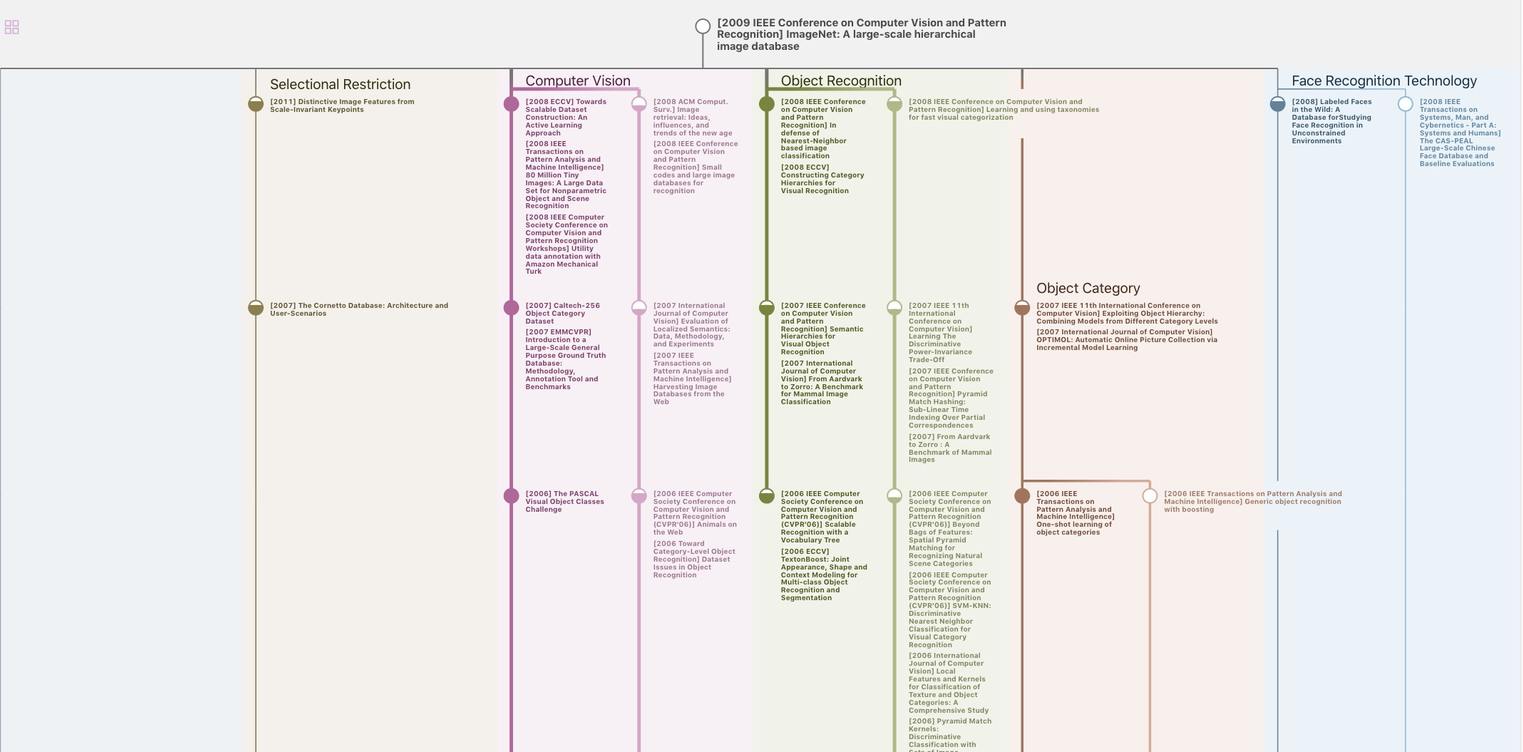
生成溯源树,研究论文发展脉络
Chat Paper
正在生成论文摘要