Subsampling based variable selection for generalized linear models
Computational statistics & data analysis(2023)
摘要
A novel variable selection method for low-dimensional generalized linear models is introduced. The new approach called AIC OPTimization via STABility Selection (OPT-STABS) repeatedly subsamples the data, minimizes Akaike's Information Criterion (AIC) over a sequence of nested models for each subsample, and includes in the final model those predictors selected in the minimum AIC model in a large fraction of the subsamples. New methods are also introduced to establish an optimal variable selection cutoff over repeated subsamples. An extensive simulation study examining a variety of proposed variable selection methods shows that, although no single method uniformly outperforms the others in all the scenarios considered, OPT-STABS is consistently among the best -performing methods in most settings while it performs competitively for the rest. This is in contrast to other candidate methods which either have poor performance across the board or exhibit good performance in some settings, but very poor in others. In addition, the asymptotic properties of the OPT-STABS estimator are derived, and its root-n consistency and asymptotic normality are proved. The methods are applied to two datasets involving logistic and Poisson regressions. (c) 2023 Elsevier B.V. All rights reserved.
更多查看译文
关键词
AIC,Screening threshold,Variable selection,Subsampling,Stability selection
AI 理解论文
溯源树
样例
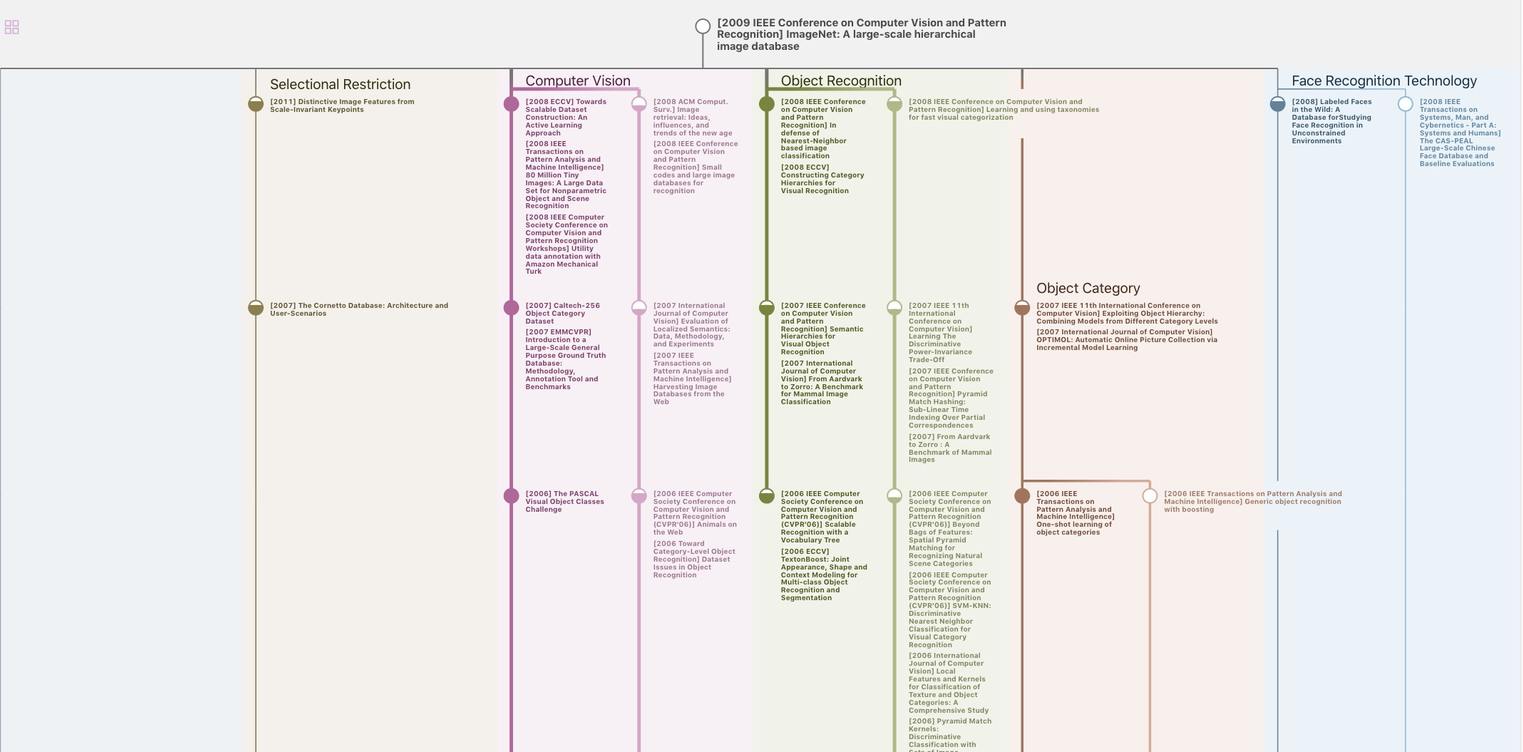
生成溯源树,研究论文发展脉络
Chat Paper
正在生成论文摘要