Optimization of chemical mixers design via tensor trains and quantum computing
arXiv (Cornell University)(2023)
摘要
Chemical component design is a computationally challenging procedure that often entails iterative numerical modeling and authentic experimental testing. We demonstrate a novel optimization method, Tensor train Optimization (TetraOpt), for the shape optimization of components focusing on a Y-shaped mixer of fluids. Due to its high parallelization and more extensive global search, TetraOpt outperforms commonly used Bayesian optimization techniques in accuracy and runtime. Besides, our approach can be used to solve general physical design problems and has linear complexity in the number of optimized parameters, which is highly relevant for complex chemical components. Furthermore, we discuss the extension of this approach to quantum computing, which potentially yields a more efficient approach.
更多查看译文
关键词
chemical mixers,tensor trains,quantum
AI 理解论文
溯源树
样例
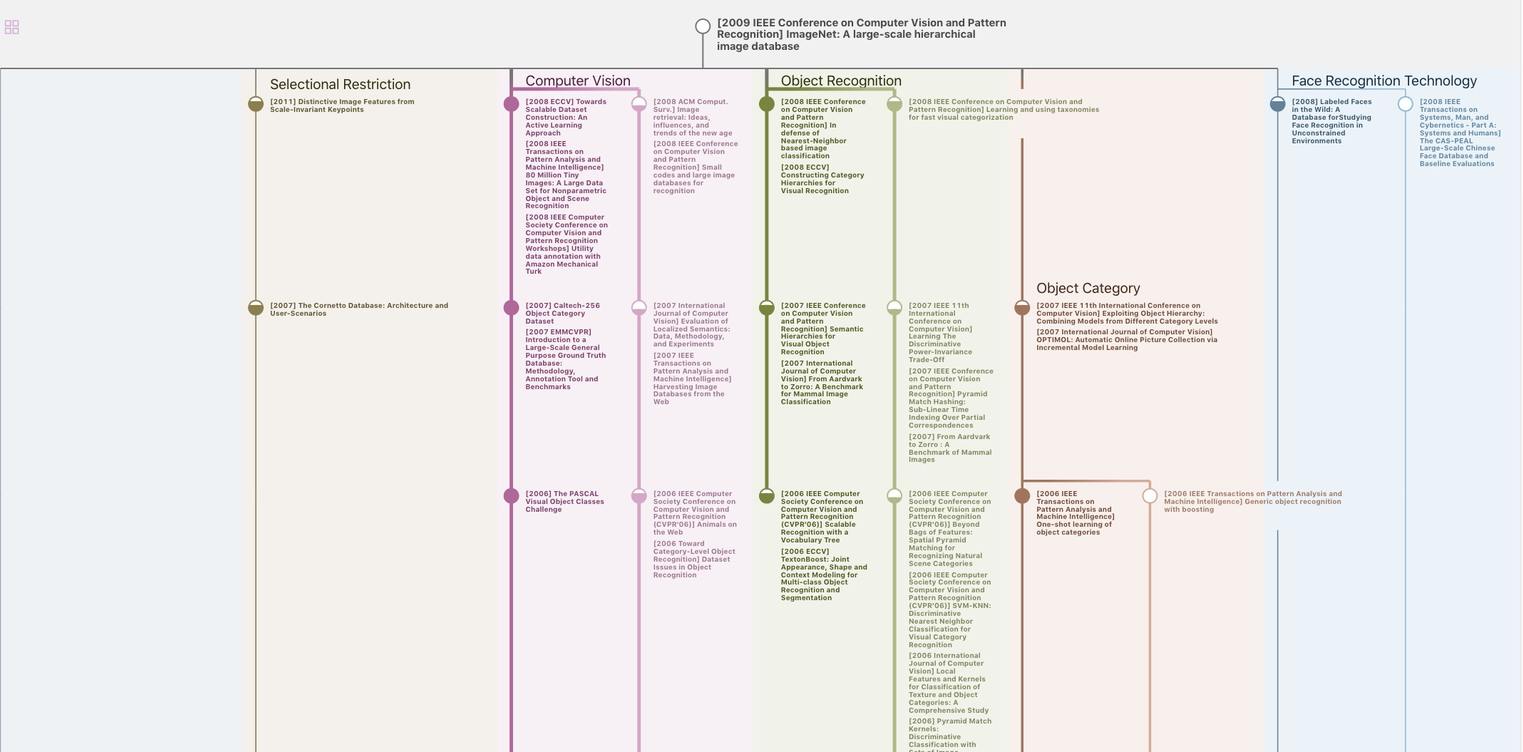
生成溯源树,研究论文发展脉络
Chat Paper
正在生成论文摘要