Developing a cost-effective emulator for groundwater flow modeling using deep neural operators
JOURNAL OF HYDROLOGY(2024)
摘要
Current groundwater models face significant challenges in their implementation due to heavy computational burdens. To overcome this, our work proposes a cost-effective emulator that efficiently and accurately forecasts the impact of abstraction in an aquifer. Our approach uses a deep neural operator (DeepONet) framework to learn operators that map between infinite -dimensional function spaces via deep neural networks. The goal is to infer the distribution of hydraulic heads in a confined aquifer in the presence of a pumping well. We successfully tested the DeepONet framework on multiple problems, including forward time -dependent problems, an inverse analysis, and a nonlinear system. Additionally, we propose a novel extension of the DeepONet-based architecture to generate accurate predictions for varied hydraulic conductivity fields and pumping well locations that are unseen during training. Our emulator's predictions match the target data with excellent performance, demonstrating that the proposed model can act as an efficient and fast tool to support a range of tasks that require repetitive forward numerical simulations or inverse simulations of groundwater flow problems. Overall, our work provides a promising avenue for developing cost-effective and accurate groundwater models.
更多查看译文
关键词
Deep neural operator,Groundwater flow,Surrogate modeling,Deep learning
AI 理解论文
溯源树
样例
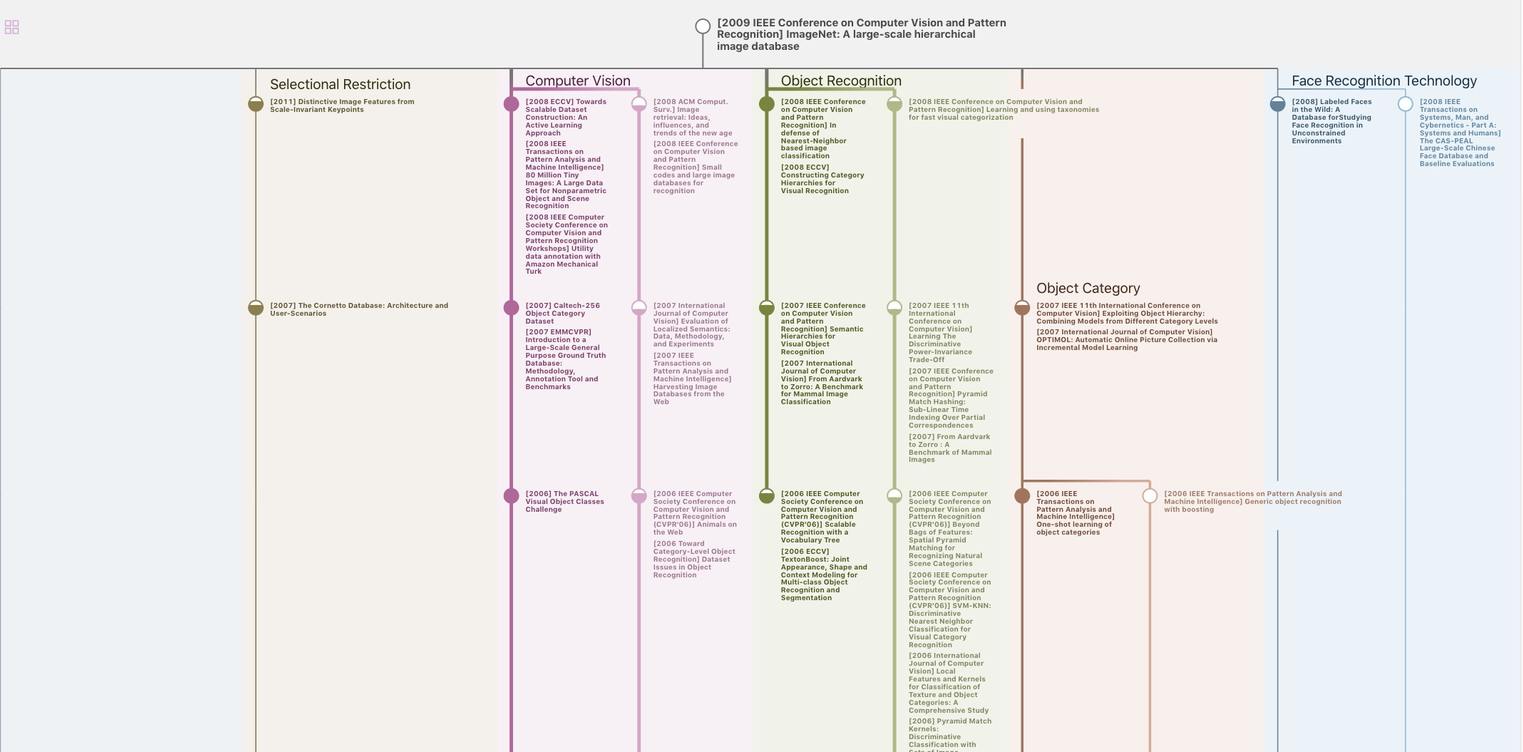
生成溯源树,研究论文发展脉络
Chat Paper
正在生成论文摘要