Hierarchical state abstraction based on structural information principles
IJCAI 2023(2023)
摘要
State abstraction optimizes decision-making by ignoring irrelevant environmental information in reinforcement learning with rich observations. Nevertheless, recent approaches focus on adequate representational capacities resulting in essential information loss, affecting their performances on challenging tasks. In this article, we propose a novel mathematical S tructural I nformation principle-sbased S tate A bstraction framework, namely SISA , from the information-theoretic perspective. Specifically, an unsupervised, adaptive hierarchical state clustering method without requiring manual assistance is presented, and meanwhile, an optimal encoding tree is generated. On each nonroot tree node, a new aggregation function and condition structural entropy are designed to achieve hierarchical state abstraction and compensate for sampling-induced essential information loss in state abstraction. Empirical evaluations on a visual gridworld domain and six continuous control benchmarks demonstrate that, compared with five SOTA state abstraction approaches, SISA significantly improves mean episode reward and sample efficiency up to 18.98 and 44.44%, respectively. Besides, we experimentally show that SISA is a general framework that can be flexibly integrated with different representation-learning objectives to improve their performances further.
更多查看译文
关键词
hierarchical state abstraction,structural information
AI 理解论文
溯源树
样例
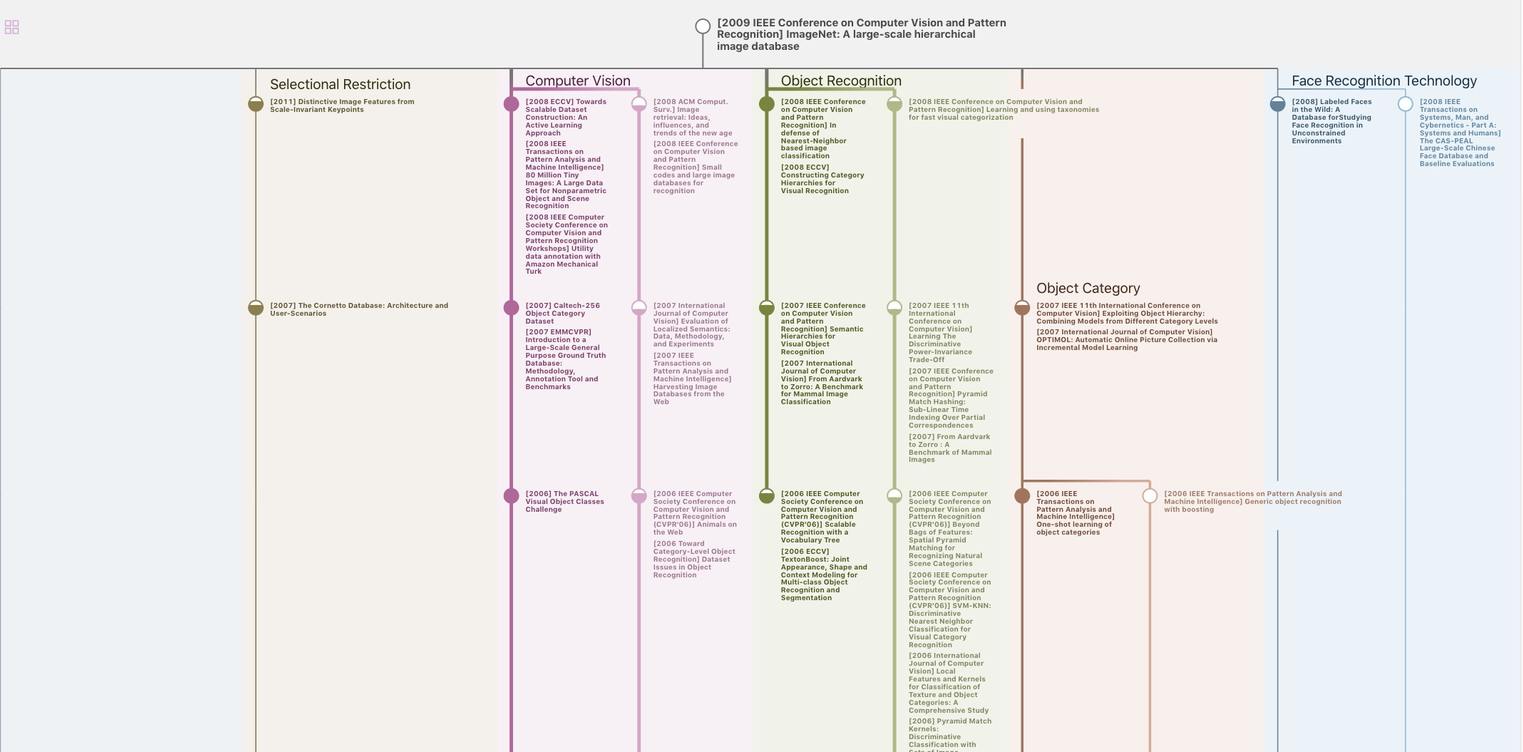
生成溯源树,研究论文发展脉络
Chat Paper
正在生成论文摘要