gSDF: Geometry-Driven Signed Distance Functions for 3D Hand-Object Reconstruction
CVPR 2023(2023)
摘要
Signed distance functions (SDFs) is an attractive framework that has recently shown promising results for 3D shape reconstruction from images. SDFs seamlessly generalize to different shape resolutions and topologies but lack explicit modelling of the underlying 3D geometry. In this work, we exploit the hand structure and use it as guidance for SDF-based shape reconstruction. In particular, we address reconstruction of hands and manipulated objects from monocular RGB images. To this end, we estimate poses of hands and objects and use them to guide 3D reconstruction. More specifically, we predict kinematic chains of pose transformations and align SDFs with highly-articulated hand poses. We improve the visual features of 3D points with geometry alignment and further leverage temporal information to enhance the robustness to occlusion and motion blurs. We conduct extensive experiments on the challenging ObMan and DexYCB benchmarks and demonstrate significant improvements of the proposed method over the state of the art.
更多查看译文
关键词
signed distance functions,3d,geometry-driven,hand-object
AI 理解论文
溯源树
样例
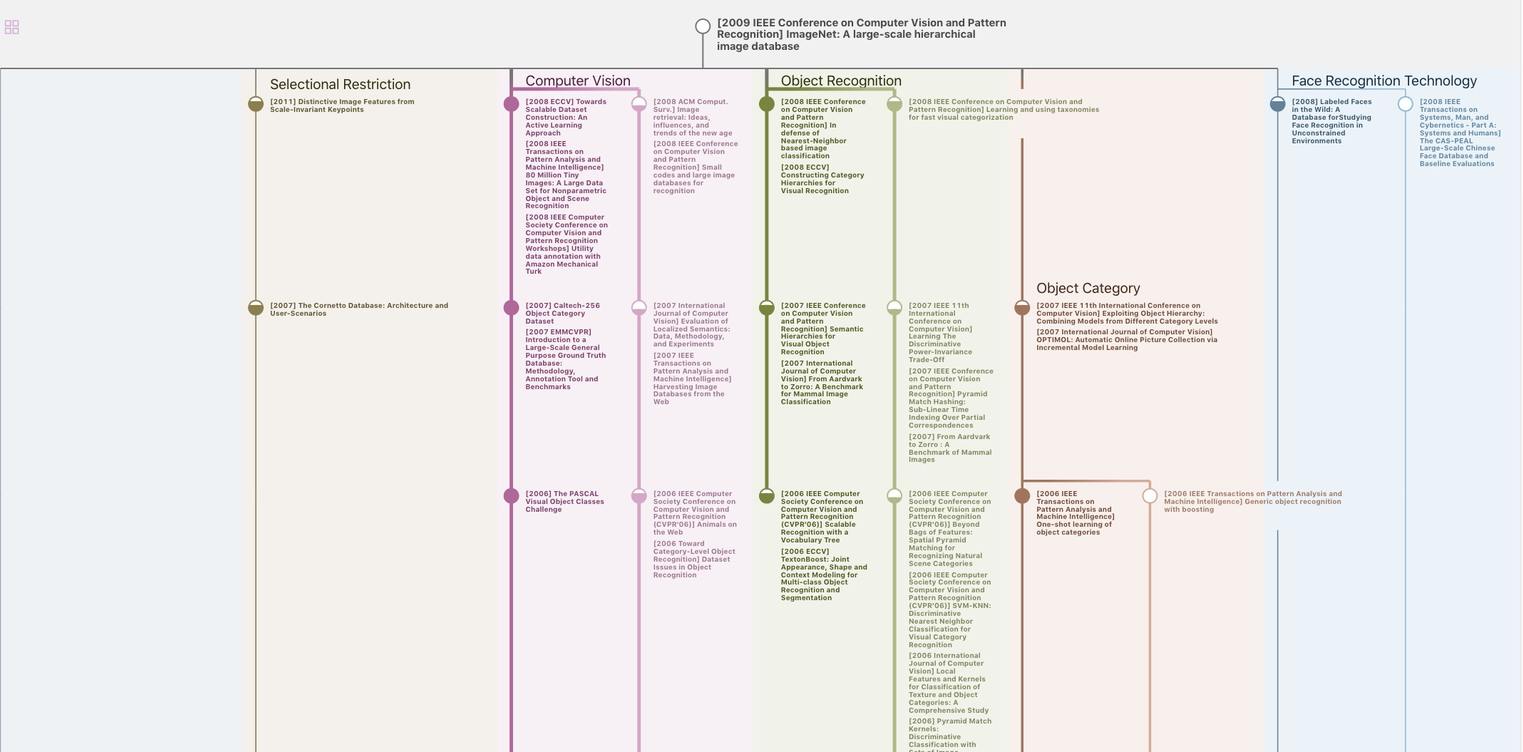
生成溯源树,研究论文发展脉络
Chat Paper
正在生成论文摘要