Can Decentralized Stochastic Minimax Optimization Algorithms Converge Linearly for Finite-Sum Nonconvex-Nonconcave Problems?
CoRR(2023)
摘要
Decentralized minimax optimization has been actively studied in the past few years due to its application in a wide range of machine learning models. However, the current theoretical understanding of its convergence rate is far from satisfactory since existing works only focus on the nonconvex-strongly-concave problem. This motivates us to study decentralized minimax optimization algorithms for the nonconvex-nonconcave problem. To this end, we develop two novel decentralized stochastic variance-reduced gradient descent ascent algorithms for the finite-sum nonconvex-nonconcave problem that satisfies the Polyak-{\L}ojasiewicz (PL) condition. In particular, our theoretical analyses demonstrate how to conduct local updates and perform communication to achieve the linear convergence rate. To the best of our knowledge, this is the first work achieving linear convergence rates for decentralized nonconvex-nonconcave problems. Finally, we verify the performance of our algorithms on both synthetic and real-world datasets. The experimental results confirm the efficacy of our algorithms.
更多查看译文
关键词
optimization,finite-sum,nonconvex-nonconcave
AI 理解论文
溯源树
样例
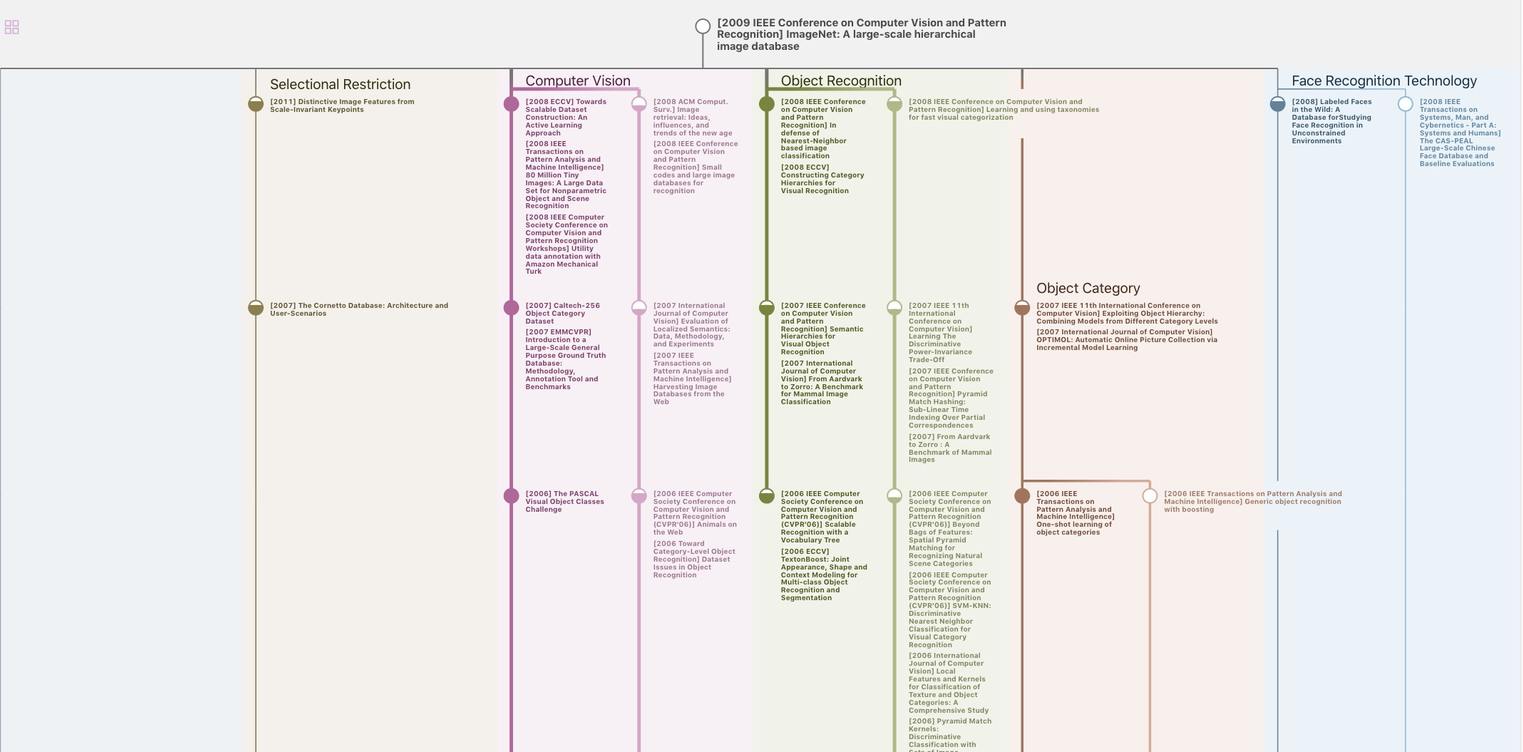
生成溯源树,研究论文发展脉络
Chat Paper
正在生成论文摘要