Towards Controllable Audio Texture Morphing
CoRR(2023)
摘要
In this paper, we propose a data-driven approach to train a Generative Adversarial Network (GAN) conditioned on "soft-labels" distilled from the penultimate layer of an audio classifier trained on a target set of audio texture classes. We demonstrate that interpolation between such conditions or control vectors provides smooth morphing between the generated audio textures, and shows similar or better audio texture morphing capability compared to the state-of-the-art methods. The proposed approach results in a well-organized latent space that generates novel audio outputs while remaining consistent with the semantics of the conditioning parameters. This is a step towards a general data-driven approach to designing generative audio models with customized controls capable of traversing out-of-distribution regions for novel sound synthesis.
更多查看译文
关键词
audio texture,morphing,audio classifier,GAN
AI 理解论文
溯源树
样例
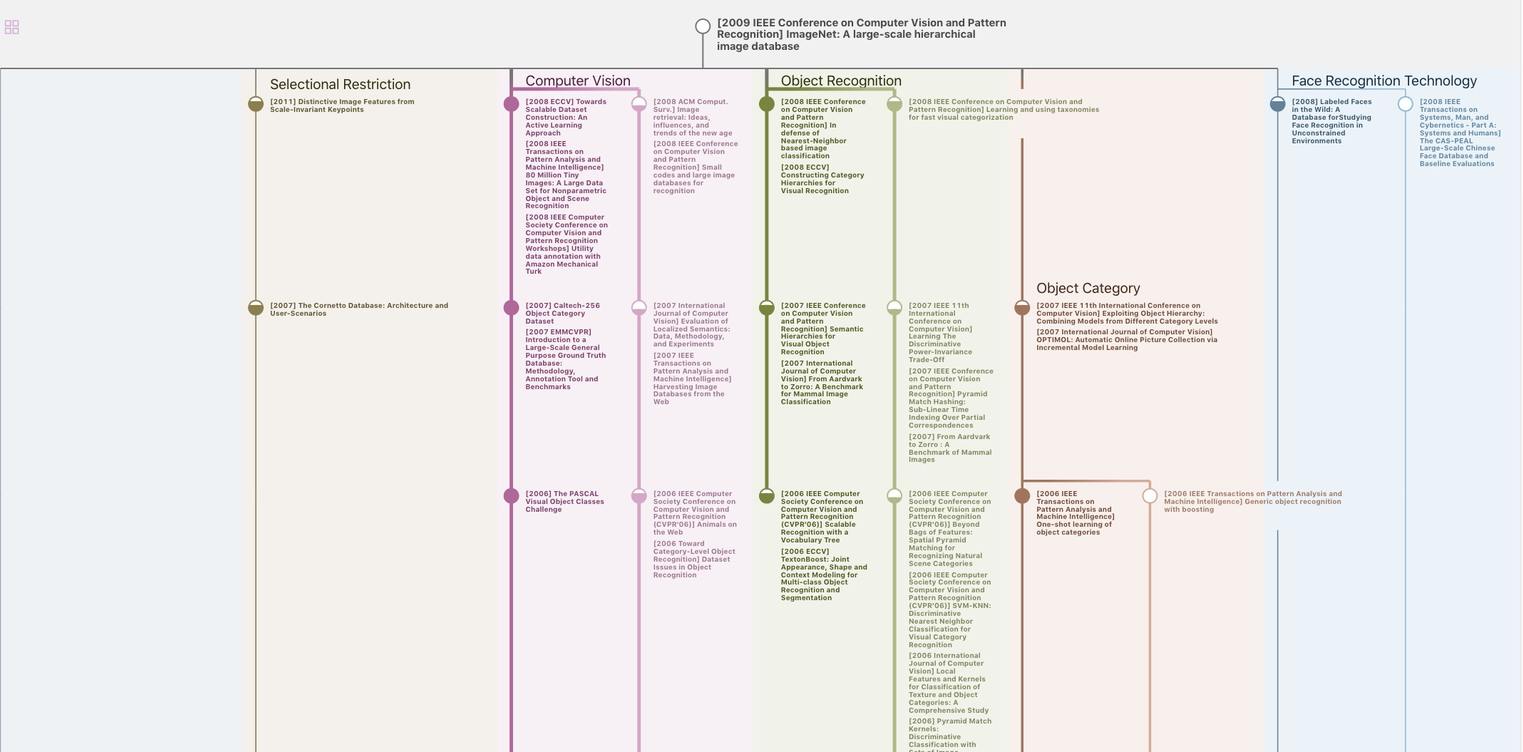
生成溯源树,研究论文发展脉络
Chat Paper
正在生成论文摘要