Reinforcement Learning with an Abrupt Model Change
2023 Winter Simulation Conference (WSC)(2023)
摘要
The problem of reinforcement learning is considered where the environment or the model undergoes a change. An algorithm is proposed that an agent can apply in such a problem to achieve the optimal long-time discounted reward. The algorithm is model-free and learns the optimal policy by interacting with the environment. It is shown that the proposed algorithm has strong optimality properties. The effectiveness of the algorithm is also demonstrated using simulation results. The proposed algorithm exploits a fundamental reward-detection trade-off present in these problems and uses a quickest change detection algorithm to detect the model change. Recommendations are provided for faster detection of model changes and for smart initialization strategies.
更多查看译文
关键词
Change Detection,Optimal Policy,Reinforcement Learning Problem,State Space,Change Model,Change Point,Self-driving,Markov Decision Process,Sequence Of States,Reinforcement Learning Algorithm,Inventory Control,External Sensors,Detection Delay,Average Reward,State-action Pair,Universal Policy,Non-parametric Algorithm,Q-learning Algorithm,Inventory Problem,State St,Change Detection Algorithm,Transition Kernel,Non-stationary Environments,Reward Function,Learning Rate,Transition Function,Stopping Rule,Reward Processing,Increase In Demand,False Alarm
AI 理解论文
溯源树
样例
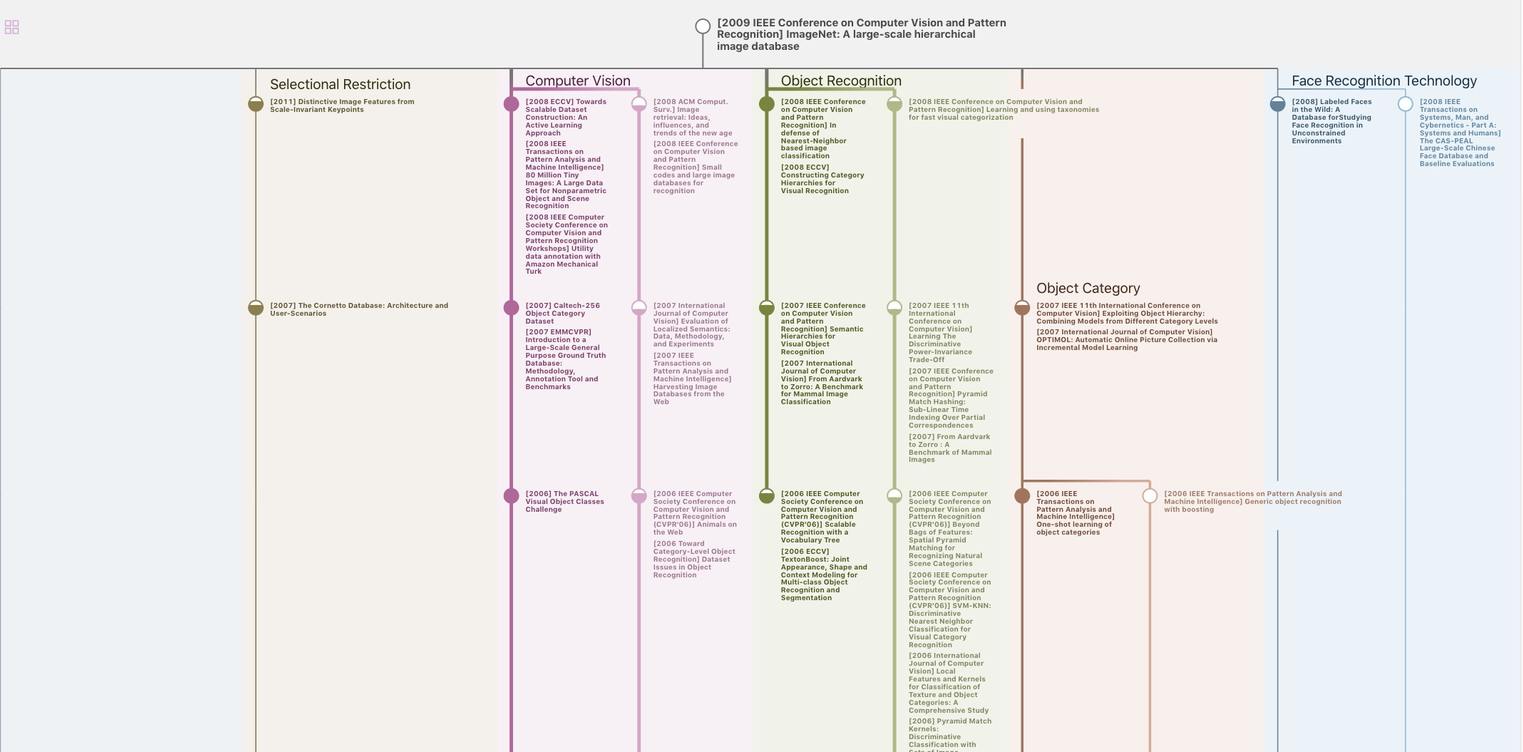
生成溯源树,研究论文发展脉络
Chat Paper
正在生成论文摘要