Determining prescriptions in electronic health care (EHR) data: methods for development of standardised, reproducible drug codelists
medrxiv(2023)
摘要
Objective Epidemiological research using electronic healthcare records(EHR) uses combinations of codes to define diseases and prescriptions (or phenotypes), requiring transparency and reproducibility. Yet methodology to generate codelists varies, manifesting in misclassification bias. Therefore we designed methodology enabling codelist reproducibility and generalisability across study contexts.
Materials and Methods We developed a process to generate drug codelists, testing this using the Clinical Practice Research Datalink (CPRD) Aurum database, accounting for missing data in ‘attribute’ variables searched to identify codes. We generated a 1) cardiovascular codelist and 2) codelist for inhaled Chronic Obstructive Pulmonary Disease (COPD) therapies, applying them to a sample cohort of 335,931 COPD patients. We compared searching on all search variables (A, “gold standard”) to B) chemical and C) ontological information only.
Results In our full search (A), within follow-up, we determined 165,150 patients (49.2% of cohort) prescribed drugs from the cardiovascular codelist. For the COPD inhalers codelist, we determined 317,963 patients (94.7% of cohort) prescribed. Considering output within each individual value set, Search C missed substantial prescriptions, including vasodilator anti-hypertensives (19,696 prescriptions for A and B; 1,145 for C), and for SAMA (35,310 for A and B; 564 for C).
Discussion Regardless of database and study context, we recommend the full method (A) for comprehensiveness. Despite database used, there are special considerations when generating adaptable drug codelists, including fluctuating status, cohort-specific drug indications, underlying hierarchical ontology, and collinearity in covariate analyses.
Conclusions Generating drug codelists must use standardisable and reproducible methodology based on underlying ontology, with end-to-end clinical input.
### Competing Interest Statement
JQ has received grants from MRC, HDR UK, GSK, BI, asthma+lung UK, and AZ and personal fees for advisory board participation, consultancy or speaking fees from GlaxoSmithKline, Evidera, AstraZeneca, Insmed. NP has received funding from Imperial Health Charity, SD is supported by the BHF Data Science Centre led by HDR UK (grant SP/19/3/34678), BigData@Heart Consortium, funded by the Innovative Medicines Initiative-2 Joint Undertaking under grant agreement 116074, the NIHR Biomedical Research Centre at University College London Hospital NHS Trust (UCLH BRC), a BHF Accelerator Award (AA/18/6/24223), E) the CVD-COVID-UK/COVID-IMPACT consortium and the Multimorbidity Mechanism and Therapeutic Research Collaborative (MMTRC, grant number MR/V033867/1). PS reports grants from asthma+lung UK and Gilead. EG, GM, AA, and SH have nothing to disclose.
### Funding Statement
No funding is reported for this study. This research was supported by the NIHR Imperial Biomedical Research Centre (BRC).
### Author Declarations
I confirm all relevant ethical guidelines have been followed, and any necessary IRB and/or ethics committee approvals have been obtained.
Yes
The details of the IRB/oversight body that provided approval or exemption for the research described are given below:
Clinical Practice Research Datalink (CPRD) has NHS Health Research Authority (HRA) Research Ethics Committee (REC) approval to allow the collection and release of anonymised primary care data for observational research [NHS HRA REC reference number 05/MRE04/87]. Each year CPRD obtains Section 251 regulatory support through the HRA Confidentiality Advisory Group (CAG), to enable patient identifiers, without accompanying clinical data, to flow from CPRD contributing GP practices in England to NHS Digital, for the purposes of data linkage [CAG reference number 21/CAG/0008]. The CPRD Research Data Governance (RDG) committee gave ethical approval for this work (protocol number 22_002515) and the approved protocol is available upon request. Linked pseudonymised data was provided for this study by CPRD. Data is linked by NHS Digital, the statutory trusted third party for linking data, using identifiable data held only by NHS Digital. Select general practices consent to this process at a practice level with individual patients having the right to opt-out.
I confirm that all necessary patient/participant consent has been obtained and the appropriate institutional forms have been archived, and that any patient/participant/sample identifiers included were not known to anyone (e.g., hospital staff, patients or participants themselves) outside the research group so cannot be used to identify individuals.
Yes
I understand that all clinical trials and any other prospective interventional studies must be registered with an ICMJE-approved registry, such as ClinicalTrials.gov. I confirm that any such study reported in the manuscript has been registered and the trial registration ID is provided (note: if posting a prospective study registered retrospectively, please provide a statement in the trial ID field explaining why the study was not registered in advance).
Yes
I have followed all appropriate research reporting guidelines, such as any relevant EQUATOR Network research reporting checklist(s) and other pertinent material, if applicable.
Yes
Data may be obtained from a third party and are not publicly available. All data are available on request from CPRD. CPRD data provision requires purchase of a license, and this license does not permit the authors to make them publicly available to all.
更多查看译文
关键词
ehr data,electronic healthcare record,prescriptions,drug
AI 理解论文
溯源树
样例
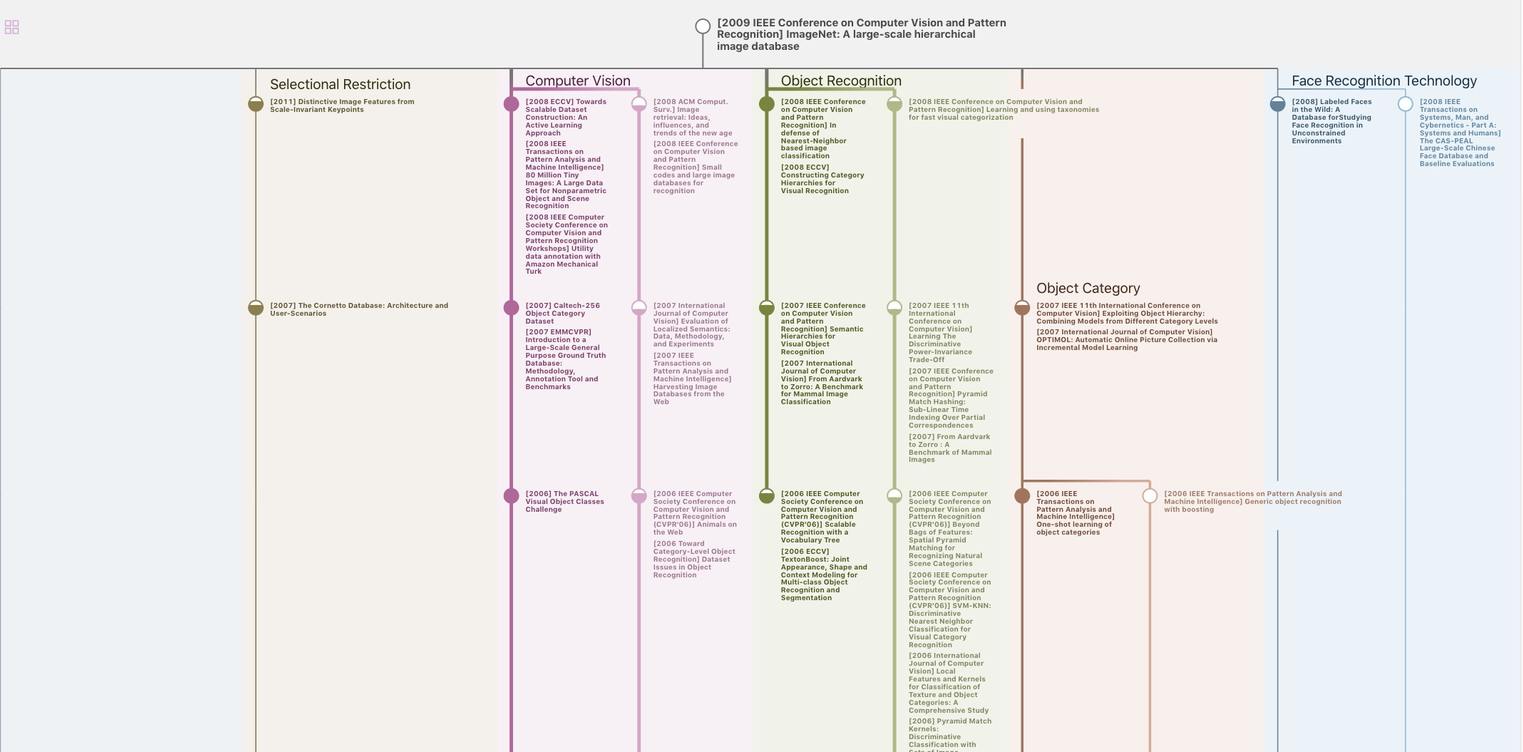
生成溯源树,研究论文发展脉络
Chat Paper
正在生成论文摘要