PowerGAN: A Machine Learning Approach for Power Side-Channel Attack on Compute-in-Memory Accelerators
CoRR(2023)
摘要
Analog compute-in-memory (CIM) accelerators are becoming increasingly popular for deep neural network (DNN) inference due to their energy efficiency and in-situ vector-matrix multiplication (VMM) capabilities. However, as the use of DNNs expands, protecting user input privacy has become increasingly important. In this paper, we identify a security vulnerability wherein an adversary can reconstruct the user's private input data from a power side-channel attack, under proper data acquisition and pre-processing, even without knowledge of the DNN model. We further demonstrate a machine learning-based attack approach using a generative adversarial network (GAN) to enhance the reconstruction. Our results show that the attack methodology is effective in reconstructing user inputs from analog CIM accelerator power leakage, even when at large noise levels and countermeasures are applied. Specifically, we demonstrate the efficacy of our approach on the U-Net for brain tumor detection in magnetic resonance imaging (MRI) medical images, with a noise-level of 20% standard deviation of the maximum power signal value. Our study highlights a significant security vulnerability in analog CIM accelerators and proposes an effective attack methodology using a GAN to breach user privacy.
更多查看译文
关键词
powergan,accelerators,side-channel,compute-in-memory
AI 理解论文
溯源树
样例
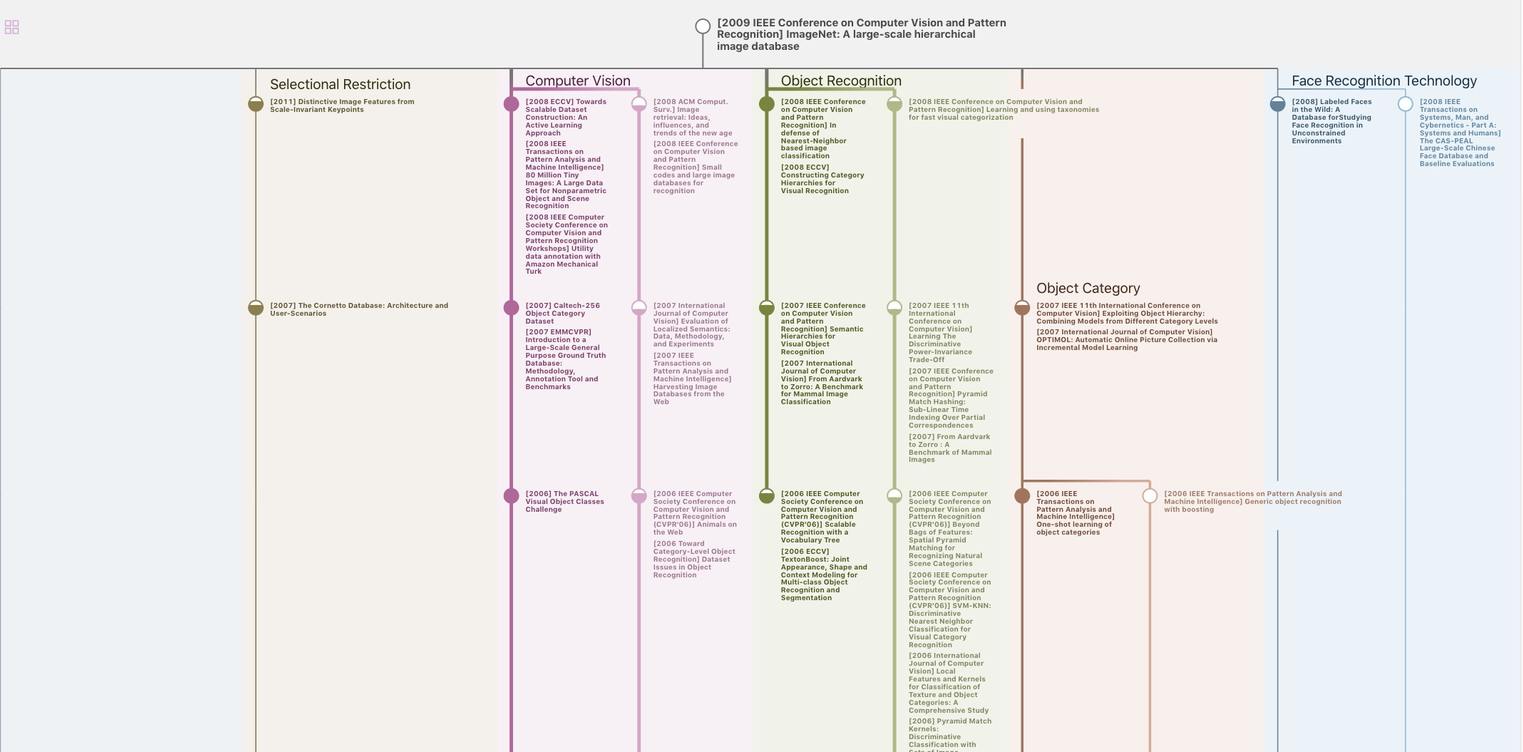
生成溯源树,研究论文发展脉络
Chat Paper
正在生成论文摘要