Knowledge distillation under ideal joint classifier assumption
NEURAL NETWORKS(2024)
摘要
Knowledge distillation constitutes a potent methodology for condensing substantial neural networks into more compact and efficient counterparts. Within this context, softmax regression representation learning serves as a widely embraced approach, leveraging a pre -established teacher network to guide the learning process of a diminutive student network. Notably, despite the extensive inquiry into the efficacy of softmax regression representation learning, the intricate underpinnings governing the knowledge transfer mechanism remain inadequately elucidated. This study introduces the 'Ideal Joint Classifier Knowledge Distillation' (IJCKD) framework, an overarching paradigm that not only furnishes a lucid and exhaustive comprehension of prevailing knowledge distillation techniques but also establishes a theoretical underpinning for prospective investigations. Employing mathematical methodologies derived from domain adaptation theory, this investigation conducts a comprehensive examination of the error boundary of the student network contingent upon the teacher network. Consequently, our framework facilitates efficient knowledge transference between teacher and student networks, thereby accommodating a diverse spectrum of applications.
更多查看译文
关键词
Knowledge distillation,Teacher-student learning,Deep learning
AI 理解论文
溯源树
样例
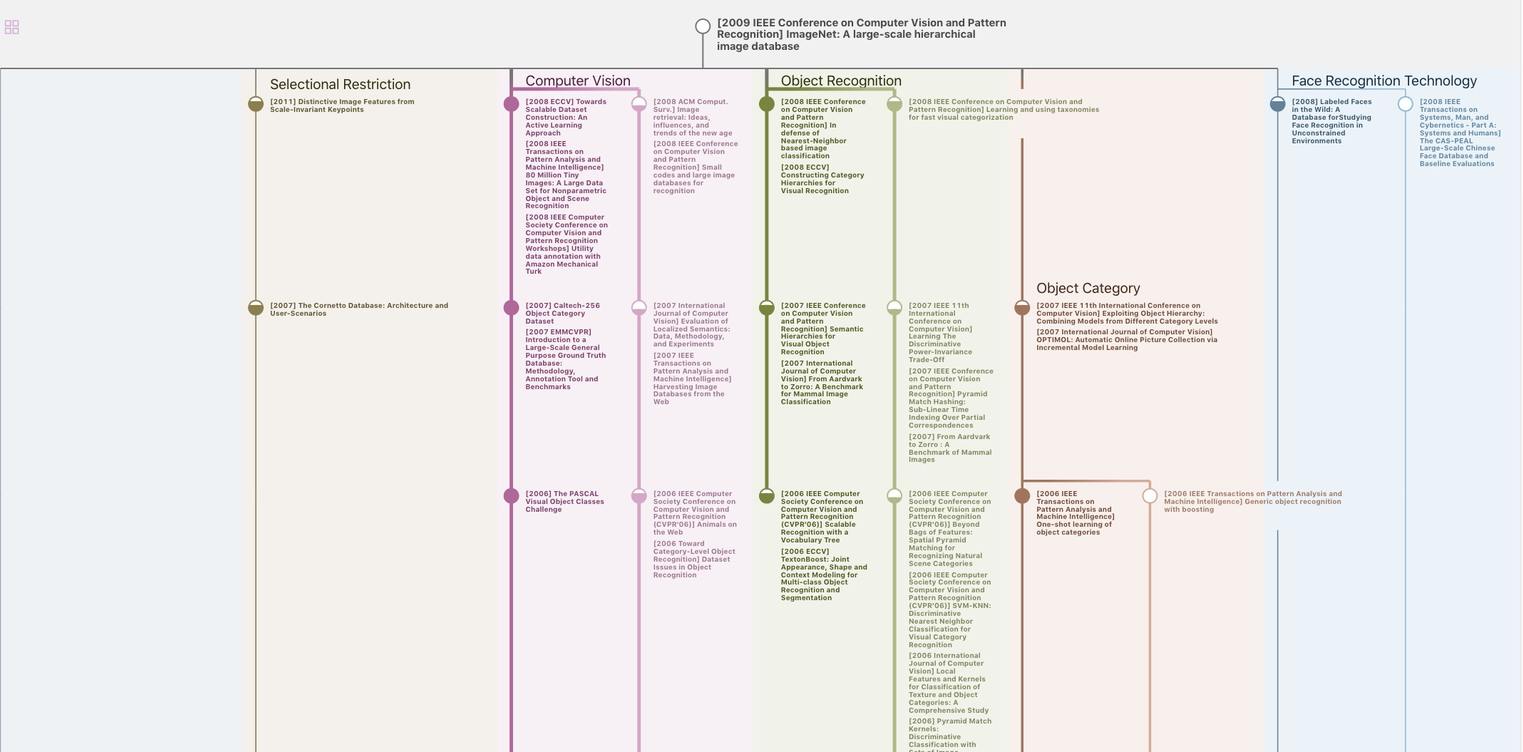
生成溯源树,研究论文发展脉络
Chat Paper
正在生成论文摘要