A revisit of the normalized eight-point algorithm and a self-supervised deep solution
CoRR(2024)
摘要
The normalized eight-point algorithm has been widely viewed as the cornerstone in two-view geometry computation, where the seminal Hartley’s normalization has greatly improved the performance of the direct linear transformation algorithm. A natural question is, whether there are and how to find other normalization methods that can further improve the performance for each input sample. In this paper, we provide a novel perspective and propose two contributions to this fundamental problem: (1) we revisit the normalized eight-point algorithm and make a theoretical contribution by presenting the existence of different and better normalization algorithms; (2) we introduce a deep convolutional neural network with a self-supervised learning strategy for normalization. Given eight pairs of correspondences, our network directly predicts the normalization matrices, thus learning to normalize each input sample. Our learning-based normalization module can be integrated with both traditional (e.g., RANSAC) and deep learning frameworks (affording good interpretability) with minimal effort. Extensive experiments on both synthetic and real images demonstrate the effectiveness of our proposed approach.
更多查看译文
关键词
Two-view geometry,Eight-point algorithm,Data normalization,Permutation invariance,Self-supervised
AI 理解论文
溯源树
样例
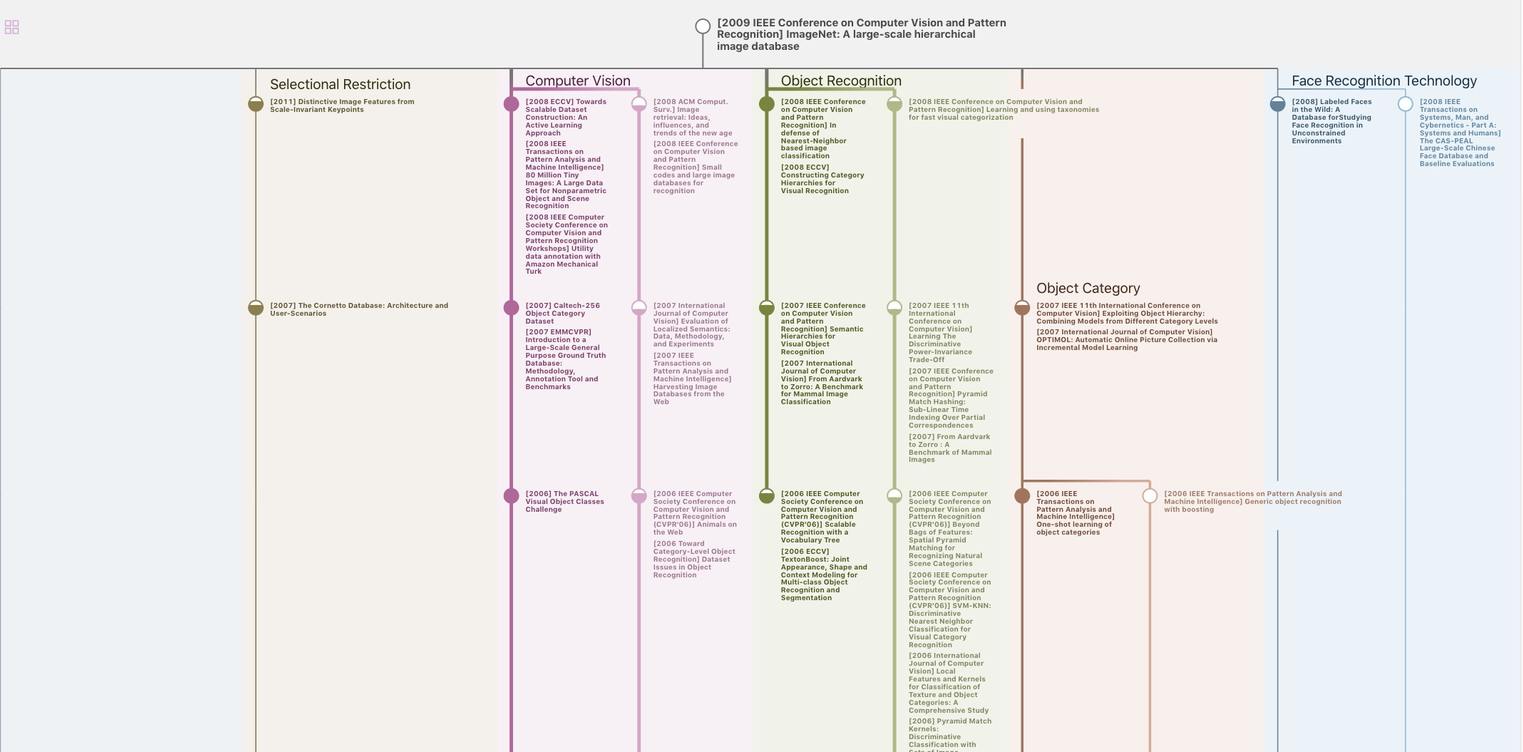
生成溯源树,研究论文发展脉络
Chat Paper
正在生成论文摘要