Persistently trained, diffusion-assisted energy-based models
STAT(2023)
摘要
Maximum likelihood (ML) learning for energy-based models (EBMs) is challenging, partly due to nonconvergence of Markov chain Monte Carlo. Several variations of ML learning have been proposed, but existing methods all fail to achieve both posttraining image generation and proper density estimation. We propose to introduce diffusion data and learn a joint EBM, called diffusion-assisted EBMs, through persistent training (i.e. using persistent contrastive divergence) with an enhanced sampling algorithm to properly sample from complex, multimodal distributions. We present results from a 2D illustrative experiment and image experiments and demonstrate that for the first time for image data, persistently trained EBMs can simultaneously achieve long-run stability, post-training image generation and superior out-of-distribution detection.
更多查看译文
关键词
energy-based models (EBMs),out-of-distribution detection,score-based diffusion models
AI 理解论文
溯源树
样例
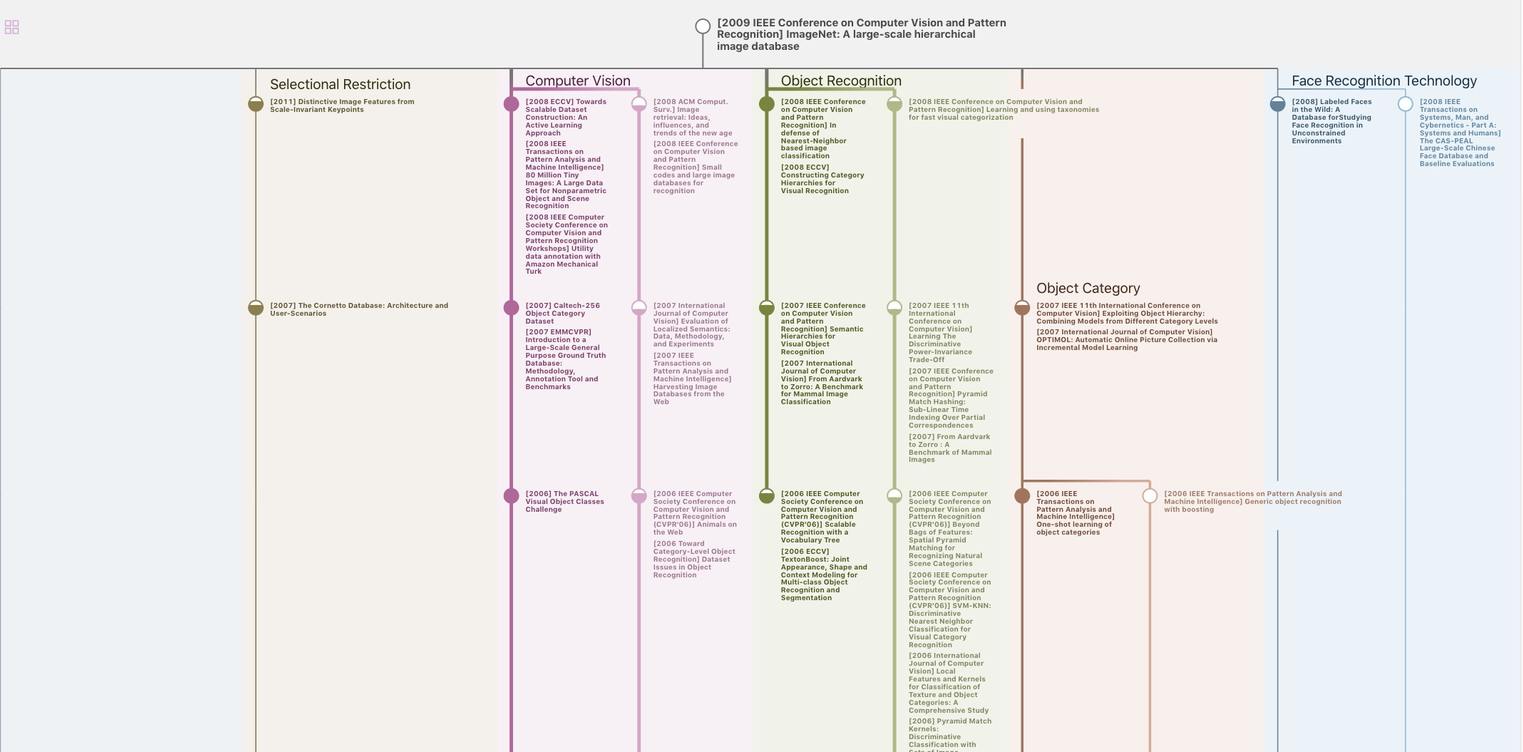
生成溯源树,研究论文发展脉络
Chat Paper
正在生成论文摘要