A Transformer-based Method to Reduce Cloud Shadow Interference in Automatic Lake Water Surface Extraction from Sentinel-2 Imagery
Journal of Hydrology(2023)
摘要
Lake extraction from remote sensing images is an important approach to monitoring water resources. It provides guidance for aiding in maintaining natural ecological balance through the artificial adjustment of lake water resources. Cloud shadows are common interference factors in lake extraction from optical images. In this paper, we proposed a deep learning network based on a novel Transformer architecture to extract lakes from Sentinel-2 imagery. To determine how training datasets affect misclassifications of cloud shadows, we produced five training datasets that contained different proportions of cloud shadows to train the network; the highest pro-portion of cloud shadows among them was 4%. We found that, when the training dataset contained 4% cloud shadows, the obtained model could effectively reduce the misclassification of cloud shadows and improve the accuracy of lake extraction, and with this model, the evaluation results over the validation dataset achieved an overall accuracy (OA) of 0.9954 and a Kappa of 0.9568. In addition, the Transformer-based network was applied in the endorheic basin of Tibetan Plateau to further evaluate the generalization ability of the network over a large area. The Global Surface Water (GSW) dataset from the European Commission Joint Research Centre was used as a reference to validate our results in this area. Our experiment and results demonstrated the underlying potential of the Transformer-based network for improving image classification of optical imagery by reducing cloud shadow inferences.
更多查看译文
关键词
cloud shadow interference,transformer-based
AI 理解论文
溯源树
样例
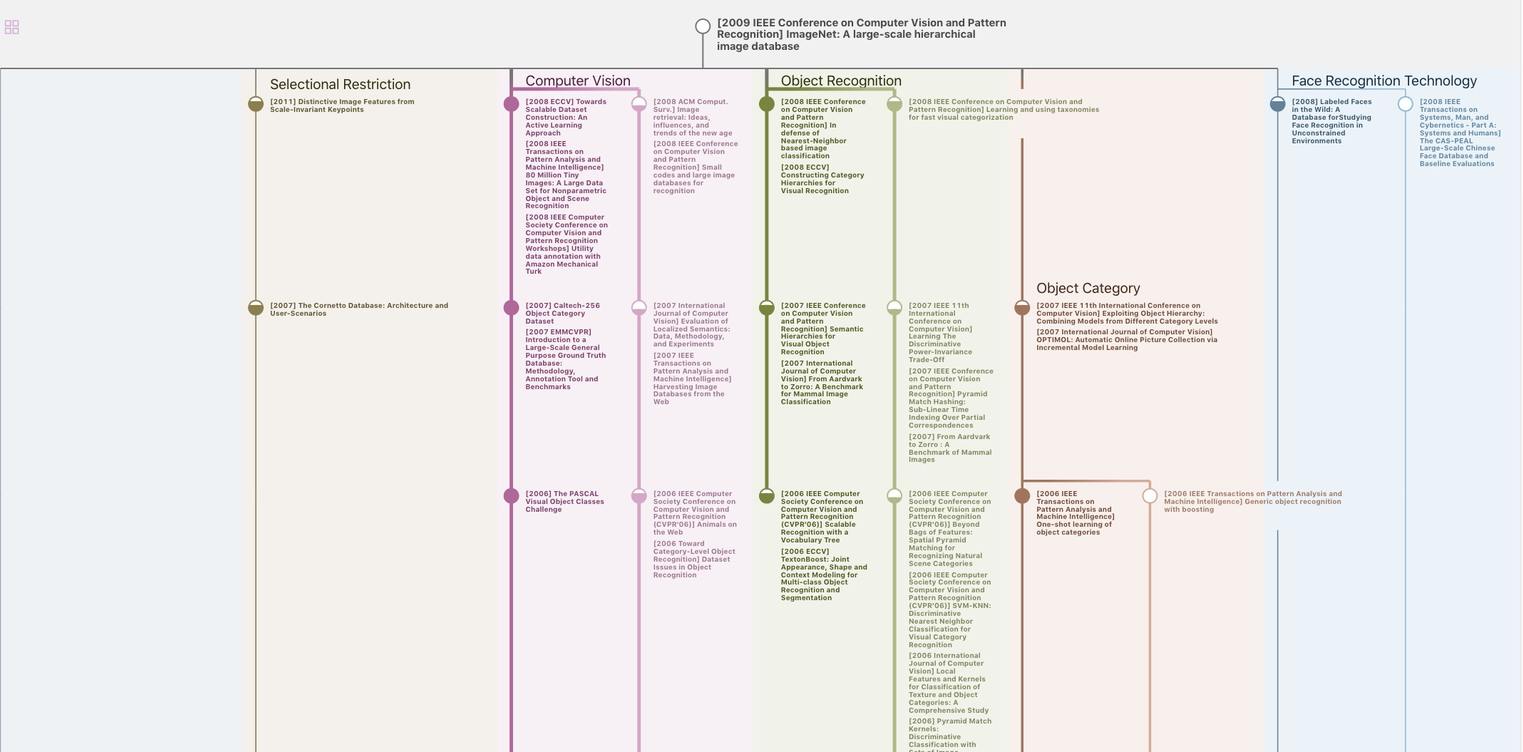
生成溯源树,研究论文发展脉络
Chat Paper
正在生成论文摘要