Adversarial Attack Mitigation Strategy for Machine Learning-Based Network Attack Detection Model in Power System
IEEE Transactions on Smart Grid(2023)
摘要
The network attack detection model based on machine learning (ML) has received extensive attention and research in PMU measurement data protection of power systems. However, well-trained ML-based detection models are vulnerable to adversarial attacks. By adding meticulously designed perturbations to the original data, the attacker can significantly decrease the accuracy and reliability of the model, causing the control center to receive unreliable PMU measurement data. This paper takes the network attack detection model in the power system as a case study to analyze the vulnerability of the ML-based detection model under adversarial attacks. And then, a mitigation strategy for adversarial attacks based on causal theory is proposed, which can enhance the robustness of the detection model under different adversarial attack scenarios. Unlike adversarial training, this mitigation strategy does not require adversarial samples to train models, saving computing resources. Furthermore, the strategy only needs a small amount of detection model information and can be migrated to various models. Simulation experiments on the IEEE node systems verify the threat of adversarial attacks against different ML-based detection models and the effectiveness of the proposed mitigation strategy.
更多查看译文
关键词
Adversarial attack,mitigation strategy,machine learning,network attack detection,vulnerability analysis,power system
AI 理解论文
溯源树
样例
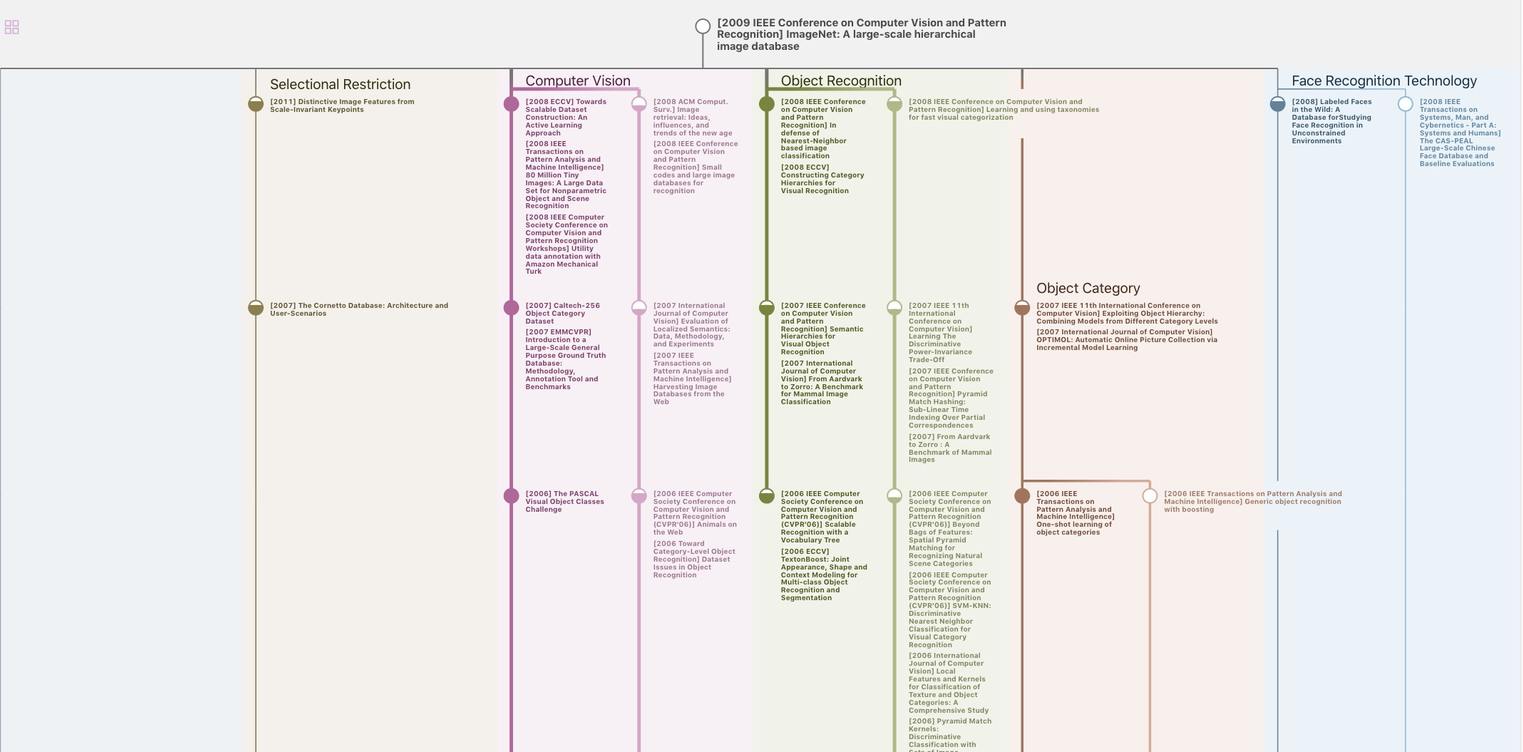
生成溯源树,研究论文发展脉络
Chat Paper
正在生成论文摘要