A Data Mining/ANFIS and Adaptive Control for Detection and Mitigation of Attacks on DC MGs
IEEE Transactions on Smart Grid(2023)
摘要
The integration of information and communication technologies (ICTs) has provided modern smart grids, such as direct current (DC) microgrids (MGs), with various advantages, e.g., the possibility of large-scale integration of renewable energy sources and complex control schemes. This extensive deployment of ICTs, however, makes DC MGs prone to a variety of cyber threats, e.g., false data injection (FDI) attacks. On this basis, this paper develops online detection and mitigation schemes to counter FDI attacks against DC MGs. Initially, in the offline training of the detection scheme, the density-based spatial clustering of applications with noise (DBSCAN) method is used for extracting prescriptive features of DC MG measurements and clustering them in healthy and under-attack operations under several uncertainties in DC MG operations. These features are used to train an adaptive network-based fuzzy inference system (ANFIS) architecture for the online identification of FDI attacks. Afterward, the trained ANFIS is used to detect FDI attacks and obtain the attack vectors benefiting from the real-time measurements estimated by an optimal state observer. Finally, an adaptive model predictive controller (AMPC) is developed to update the power-sharing commands—and mitigate the FDI attack—using the ANFIS’s obtained attack vectors. The effectiveness of the proposed framework is evaluated for several realistic FDI attack scenarios and different uncertainties. To show the scalability of the proposed detection and mitigation methods, a DC MG with eight converters is also studied in the presence of FDI attacks and uncertainties using real-time simulations.
更多查看译文
关键词
Direct current microgrid,data-mining clustering method,constant power loads,adaptive model predictive controller
AI 理解论文
溯源树
样例
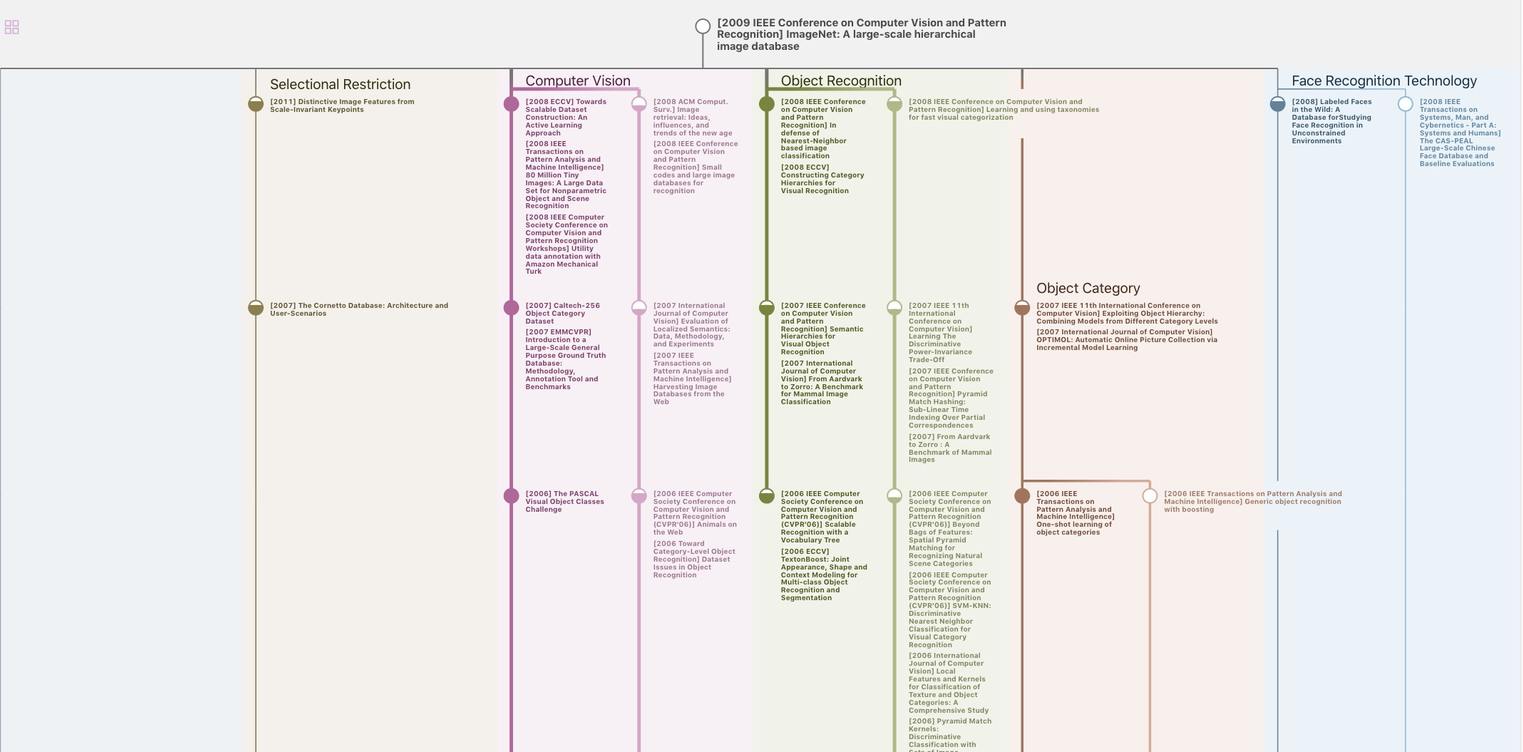
生成溯源树,研究论文发展脉络
Chat Paper
正在生成论文摘要