Validating a low-cost, open-source, locally manufactured workstation and computational pipeline for automated histopathology evaluation using deep learning
bioRxiv (Cold Spring Harbor Laboratory)(2023)
摘要
Deployment and access to state-of-the-art diagnostic technologies remains a fundamental challenge in providing equitable global cancer care to low-resource settings. The expansion of digital pathology in recent years and its interface with computational biomarkers provides an opportunity to democratize access to personalized medicine. Here we describe a low-cost platform for digital side capture and computational analysis composed of open-source components. The platform provides low-cost ($200) digital image capture from glass slides and is capable of real-time computational image analysis using an open-source deep learning (DL) algorithm and Raspberry Pi ($35) computer. We validate the performance of deep learning models' performance using images captured from the open-source workstation and show similar model performance when compared against significantly more expensive standard institutional hardware.
### Competing Interest Statement
A.T.P. reports advisory boards for Abbvie, Elevar, Privo, Ayala, and Prelude. A.T.P. reports research funding from Abbvie and Kura Oncology. J.N.K. provides consulting services for Owkin, Panakeia, and DoMore Diagnostics.
更多查看译文
关键词
histopathology evaluation,deep learning,computational pipeline,low-cost,open-source
AI 理解论文
溯源树
样例
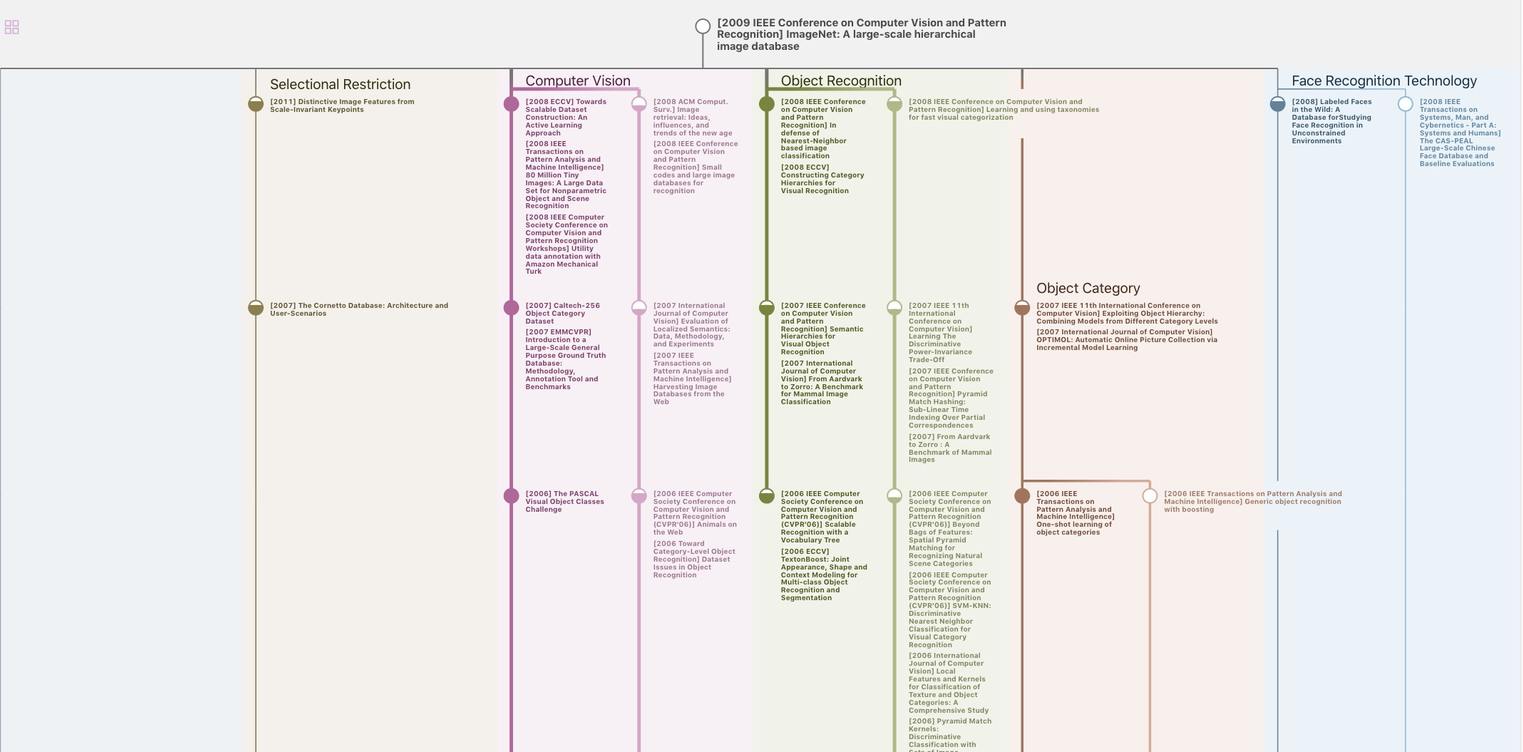
生成溯源树,研究论文发展脉络
Chat Paper
正在生成论文摘要