Benchmarking Uncertainty Quantification for Protein Engineering
bioRxiv (Cold Spring Harbor Laboratory)(2023)
摘要
Machine learning sequence-function models for proteins could enable significant ad vances in protein engineering, especially when paired with state-of-the-art methods to select new sequences for property optimization and/or model improvement. Such methods (Bayesian optimization and active learning) require calibrated estimations of model uncertainty. While studies have benchmarked a variety of deep learning uncertainty quantification (UQ) methods on standard and molecular machine-learning datasets, it is not clear if these results extend to protein datasets. In this work, we implemented a panel of deep learning UQ methods on regression tasks from the Fitness Landscape Inference for Proteins (FLIP) benchmark. We compared results across different degrees of distributional shift using metrics that assess each UQ method’s accuracy, calibration, coverage, width, and rank correlation. Additionally, we compared these metrics using one-hot encoding and pretrained language model representations, and we tested the UQ methods in a retrospective active learning setting. These benchmarks enable us to provide recommendations for more effective design of biological sequences using machine learning.
### Competing Interest Statement
The authors have declared no competing interest.
更多查看译文
关键词
uncertainty quantification,protein
AI 理解论文
溯源树
样例
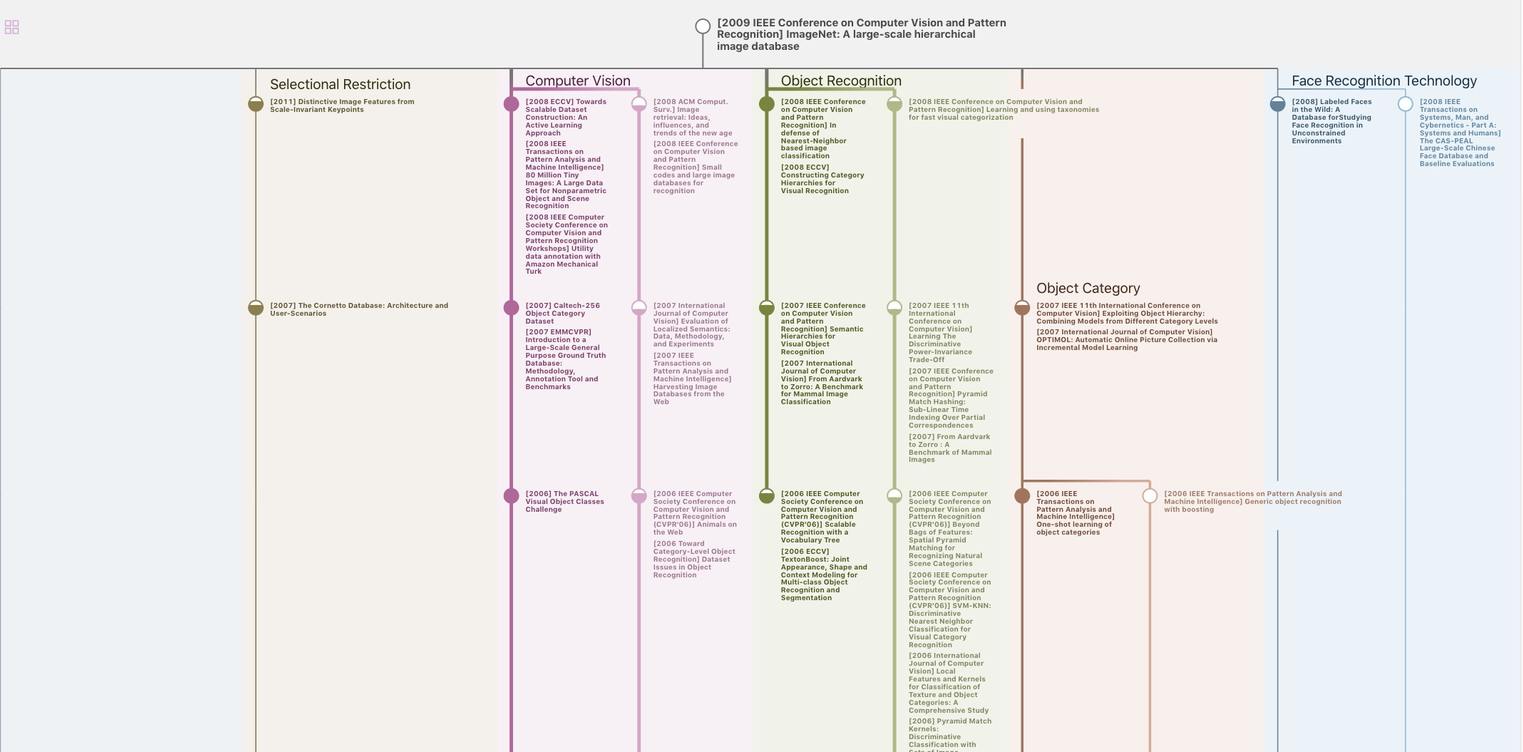
生成溯源树,研究论文发展脉络
Chat Paper
正在生成论文摘要