Permeability prediction and uncertainty quantification base on Bayesian neural network and data distribution domain transformation
Chinese Journal of Geophysics(2023)
摘要
Permeability is a key parameter for reservoir evaluation and reservoir development. The permeability estimated by traditional logging methods and conventional machine learning methods is a fixed value. However, due to the noise in the logging data itself, the prediction results of permeability may be affected by the noise, resulting in random measurement errors(ie, arbitrary uncertainty). At the same time, when there are differences between test data and training data, the machine learning model may have the uncertainty of model parameters(i.e. cognitive uncertainty) in predicting permeability. In order to realize the accurate prediction of permeability and quantify the impact of two uncertainties on the results, this paper proposes to realize the prediction of permeability and the estimation of its uncertainty simultaneously based on data distribution domain transformation and Bayesian neural network. The proposed method mainly includes two parts: one is the mutual transformation of data distribution in different domains, and the other is the neural network permeability modeling, prediction and uncertainty estimation based on Bayesian theory. Due to the assumption of data distribution in Bayesian neural network, Bayesian neural network can better learn the relationship between data when the probability distribution of label is consistent with the distribution of network. Therefore, by looking for a function, the permeability label of an original domain is transformed into the permeability related variable of the target domain(we call it the permeability of the target domain), so that the variable conforms to the distribution assumption of Bayesian neural network. We use Bayesian neural network to predict target domain penetration, as well as arbitrary uncertainty and cognitive uncertainty. Then, based on the inverse transformation of distribution domain, we restore the permeability of target domain back to the permeability of original domain. This method is applied to the logging data of 18 wells in an oil field. The data of 16 wells are used for training and 2 wells are tested. The predicted permeability of the test well is basically consistent with the real permeability. At the same time, the prediction results with arbitrary uncertainty provide the location where the permeability prediction value is affected by the noise of logging data. The prediction results of cognitive uncertainty show that the location with less data has higher cognitive uncertainty. The proposed process not only shows great potential in reservoir characterization, but also reduces the risk of logging interpretation.
更多查看译文
关键词
Bayesian neural network,Permeability prediction,Data distribution domain transformation,Artificial intelligence,Uncertainty estimation
AI 理解论文
溯源树
样例
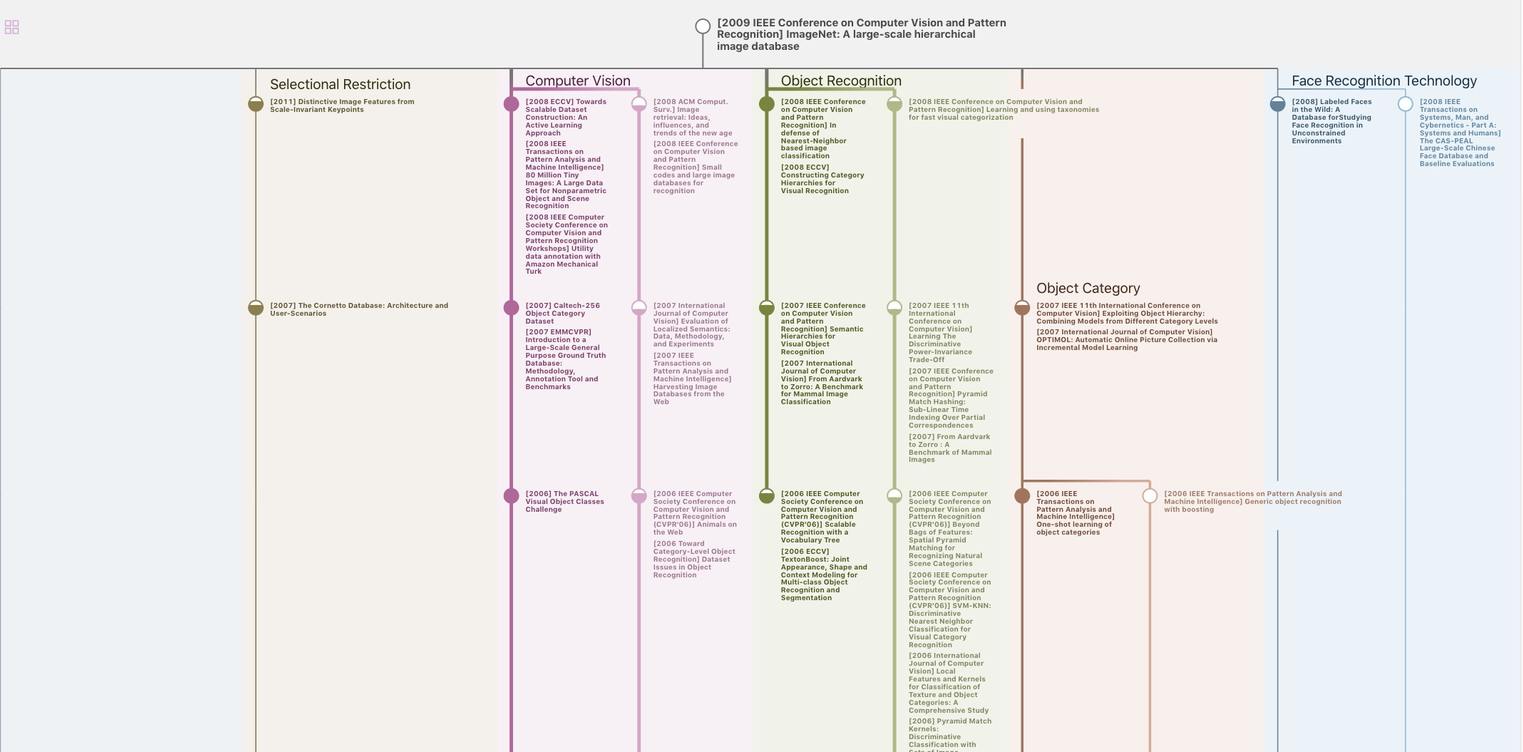
生成溯源树,研究论文发展脉络
Chat Paper
正在生成论文摘要