Novel Molecular Representations Using Neumann-Cayley Orthogonal Gated Recurrent Unit.
Journal of chemical information and modeling(2023)
摘要
Advances in deep neural networks (DNNs) have made a very powerful machine learning method available to researchers across many fields of study, including the biomedical and cheminformatics communities, where DNNs help to improve tasks such as protein performance, molecular design, drug discovery, etc. Many of those tasks rely on molecular descriptors for representing molecular characteristics in cheminformatics. Despite significant efforts and the introduction of numerous methods that derive molecular descriptors, the quantitative prediction of molecular properties remains challenging. One widely used method of encoding molecule features into bit strings is the molecular fingerprint. In this work, we propose using new Neumann-Cayley Gated Recurrent Units (NC-GRU) inside the Neural Nets encoder (AutoEncoder) to create neural molecular fingerprints (NC-GRU fingerprints). The NC-GRU AutoEncoder introduces orthogonal weights into widely used GRU architecture, resulting in faster, more stable training, and more reliable molecular fingerprints. Integrating novel NC-GRU fingerprints and Multi-Task DNN schematics improves the performance of various molecular-related tasks such as toxicity, partition coefficient, lipophilicity, and solvation-free energy, producing state-of-the-art results on several benchmarks.
更多查看译文
关键词
recurrent,neumann-cayley
AI 理解论文
溯源树
样例
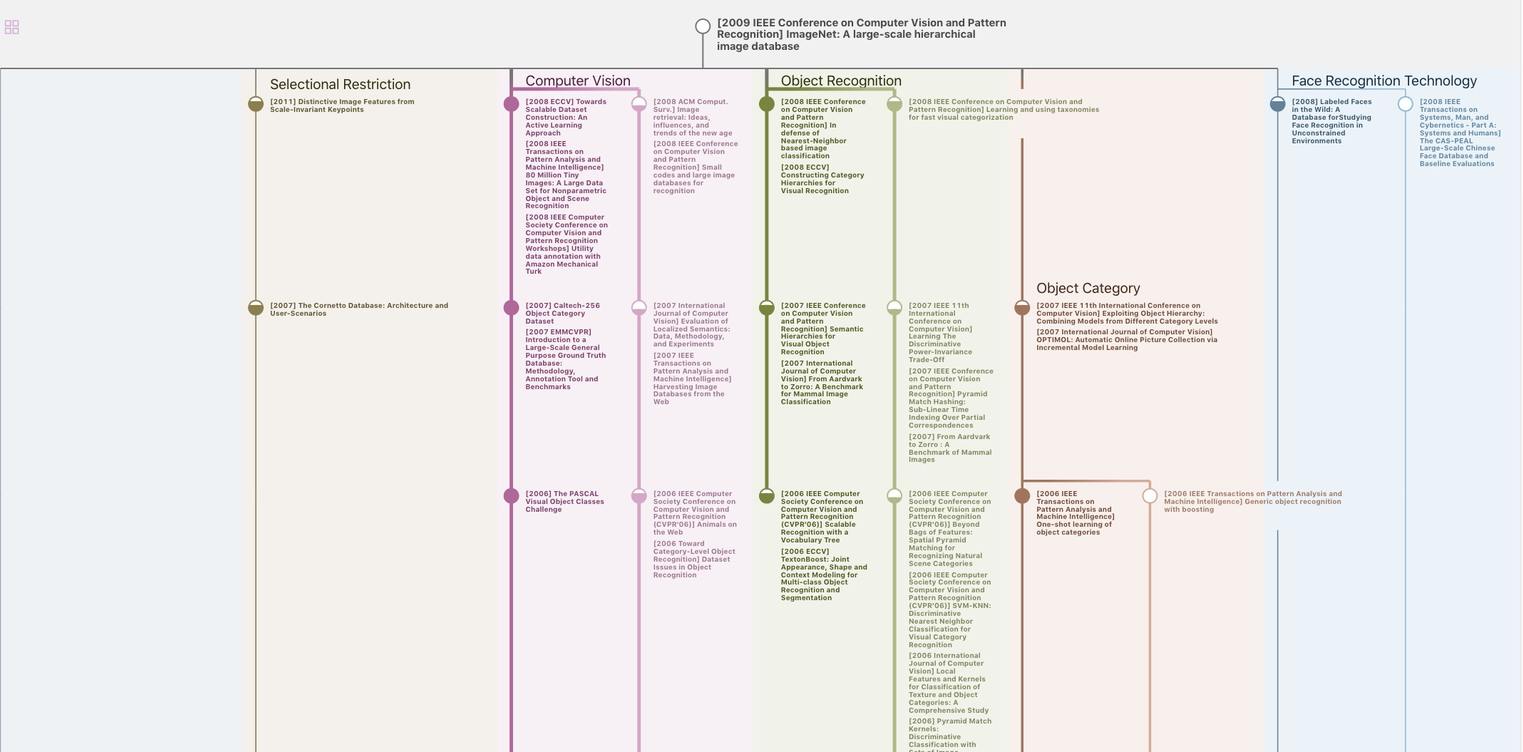
生成溯源树,研究论文发展脉络
Chat Paper
正在生成论文摘要