Bayesian inference of spike-time dependent learning rules from single neuron recordings in humans
crossref(2023)
摘要
The spike-timing dependent plasticity (STDP) learning rules are popular in both neuroscience and computer algorithms due to their ability to capture the change in neural connections arising from the correlated activity of neurons. Recent advances in recording technology have made large neural recordings common, but to date, there is no established method for inferring unobserved neural connections and learning rules from them. We use a Bayesian framework and assume neural spike recordings follow a binary data model to infer the connections and their evolution over time from data using STDP rules. We test the resulting method on one simulated and one real case study, where the real case study consists of human electrophysiological recordings. The simulated case study allows validation of the model, and the real case study shows that we are able to infer learning rules from awake human data that align with experimental results in rats and other animals. The real case study also uncovered a trend in maximum synaptic modification and age, however, this will need testing on a larger scale to draw conclusions.
更多查看译文
AI 理解论文
溯源树
样例
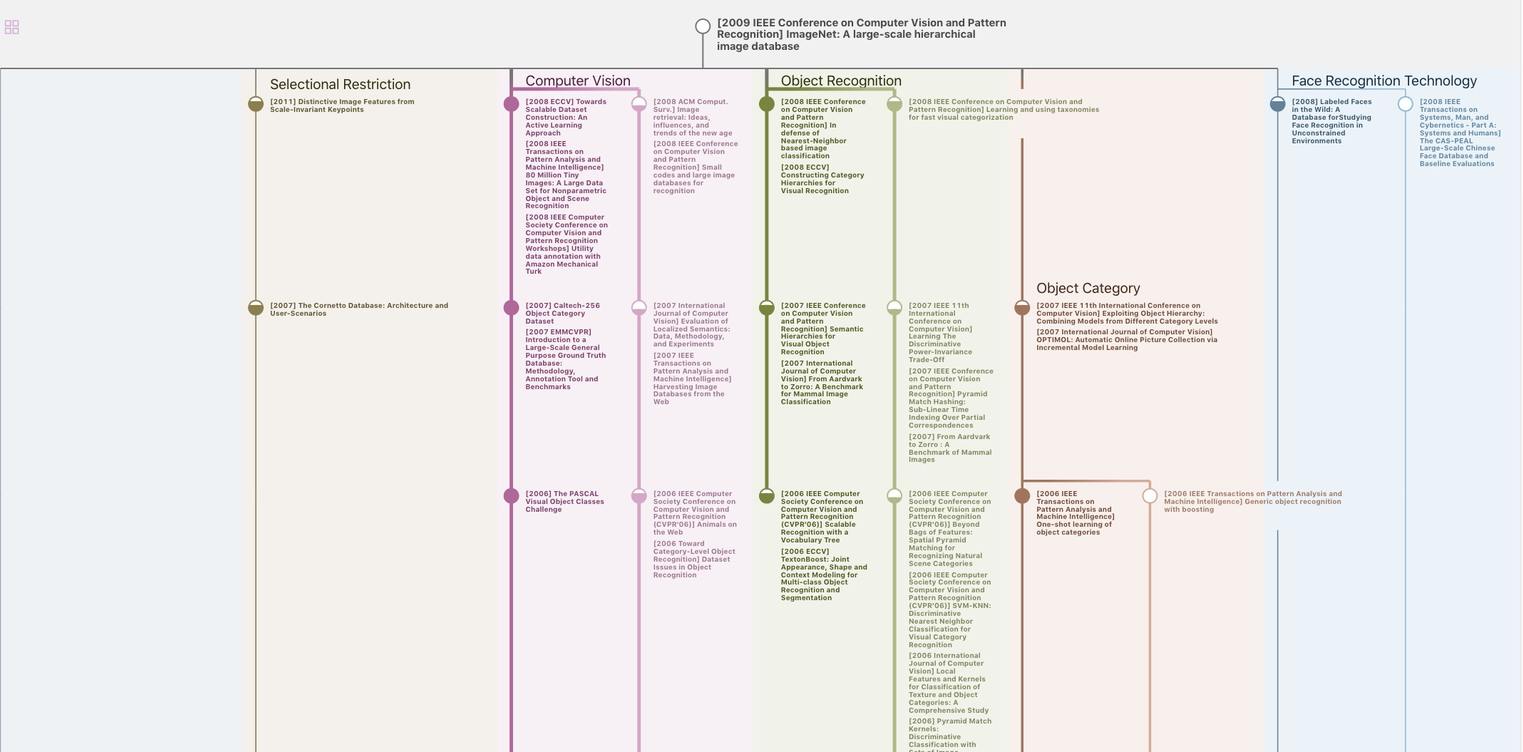
生成溯源树,研究论文发展脉络
Chat Paper
正在生成论文摘要