Graph deep learning enabled spatial domains identification for spatial transcriptomics
Briefings in Bioinformatics(2023)
摘要
Advancing spatially resolved transcriptomics (ST) technologies help biologists comprehensively understand organ function and tissue microenvironment. Accurate spatial domain identification is the foundation for delineating genome heterogeneity and cellular interaction. Motivated by this perspective, a graph deep learning (GDL) based spatial clustering approach is constructed in this paper. First, the deep graph infomax module embedded with residual gated graph convolutional neural network is leveraged to address the gene expression profiles and spatial positions in ST. Then, the Bayesian Gaussian mixture model is applied to handle the latent embeddings to generate spatial domains. Designed experiments certify that the presented method is superior to other state-of-the-art GDL-enabled techniques on multiple ST datasets. The codes and dataset used in this manuscript are summarized at https://github. com/narutoten520/SCGDL.
更多查看译文
关键词
spatial transcriptome,spatial clustering,graph deep learning,residual gated graph convolutional neural network,deep graph infomax,Bayesian Gaussian mixture models
AI 理解论文
溯源树
样例
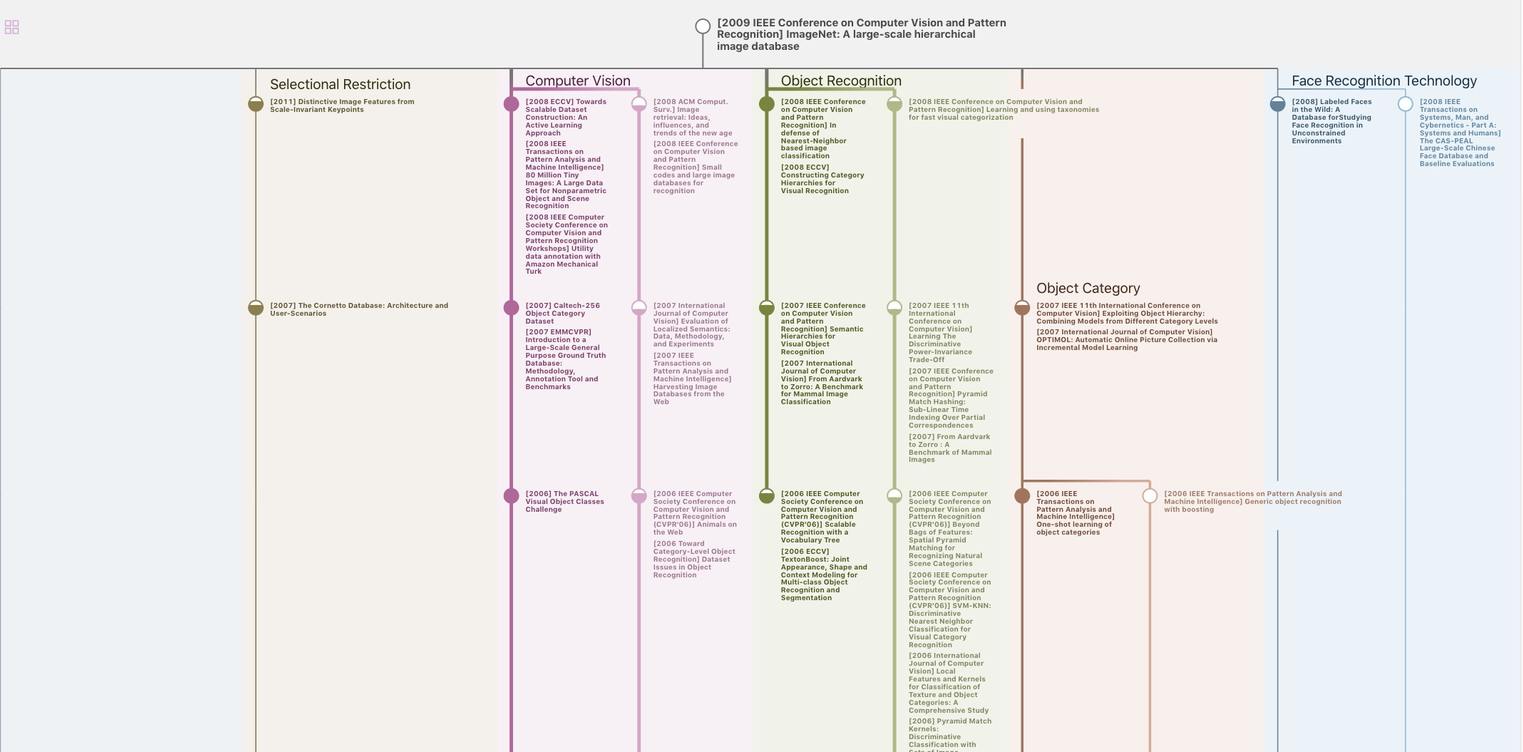
生成溯源树,研究论文发展脉络
Chat Paper
正在生成论文摘要