Quantum Kernel Alignment with Stochastic Gradient Descent
CoRR(2023)
摘要
Quantum support vector machines have the potential to achieve a quantum speedup for solving certain machine learning problems. The key challenge for doing so is finding good quantum kernels for a given data set -- a task called kernel alignment. In this paper we study this problem using the Pegasos algorithm, which is an algorithm that uses stochastic gradient descent to solve the support vector machine optimization problem. We extend Pegasos to the quantum case and and demonstrate its effectiveness for kernel alignment. Unlike previous work which performs kernel alignment by training a QSVM within an outer optimization loop, we show that using Pegasos it is possible to simultaneously train the support vector machine and align the kernel. Our experiments show that this approach is capable of aligning quantum feature maps with high accuracy, and outperforms existing quantum kernel alignment techniques. Specifically, we demonstrate that Pegasos is particularly effective for non-stationary data, which is an important challenge in real-world applications.
更多查看译文
关键词
QSVM,Pegasos,Kernel Alignment,Quantum Kernel,Quantum Kernel Training,Quantum Support Vector Machine
AI 理解论文
溯源树
样例
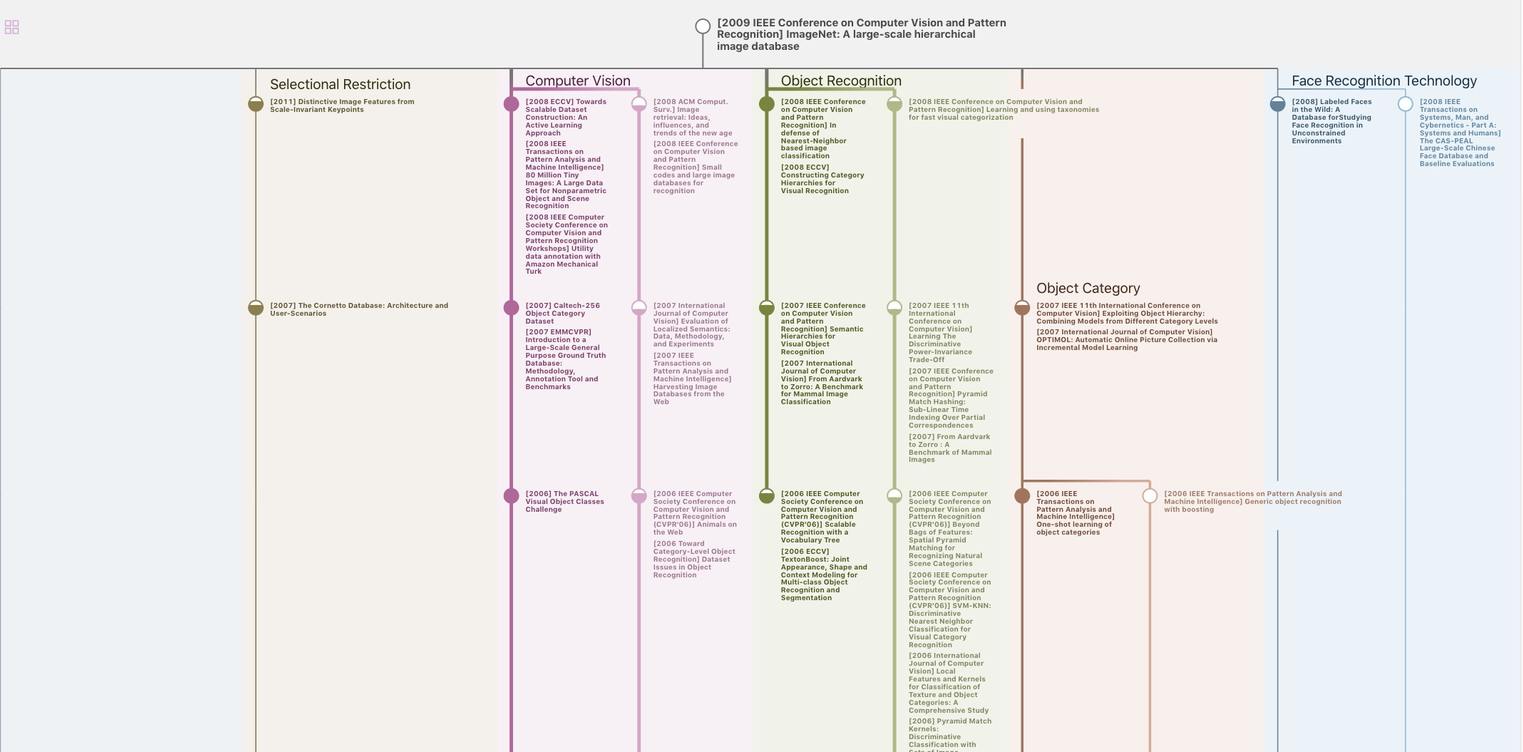
生成溯源树,研究论文发展脉络
Chat Paper
正在生成论文摘要