Hybrid Deep Learning for Channel Estimation and Power Assignment for MISO-NOMA System
2023 International Conference on IT Innovation and Knowledge Discovery (ITIKD)(2023)
摘要
In this paper, the influence of Deep Neural Network (DNN) in predicting both the channel parameters and the power factors for users in a Power Domain Multi-Input Single-Output Non-Orthogonal Multiple Access (MISO-NOMA) system is inspected. In channel prediction based Deep Learning (DL) approach, we integrate the Long Short Term Memory (LSTM) learning network into NOMA system in order that LSTM can be utilized to predict the channel coefficients. Additionally, in Deep Learning based power estimation method, we introduce an algorithm based on Convolutional Neural Network (CNN) to predict and allocate the power factor for each user in MISO-NOMA cell. DNN is trained online using channel statistics in order to approximate the channel coefficients and allocate the power factors for each user, so that these parameters can be utilized by the receiver to recover the desired data. Besides, this paper introduce a framework where channel prediction based on LSTM layer and power approximation based on CNN can be jointly employed for multiuser detection in MISO-NOMA. In this work, Power factors are optimized analytically based on maximizing the sum-rate of users to derive the optimum power factors. Simulation outcomes for distinct metrics have verified the dominance of the channel estimation and power predication based DNN over standard approaches.
更多查看译文
关键词
DL,LSTM,CNN,NOMA,KKT conditions
AI 理解论文
溯源树
样例
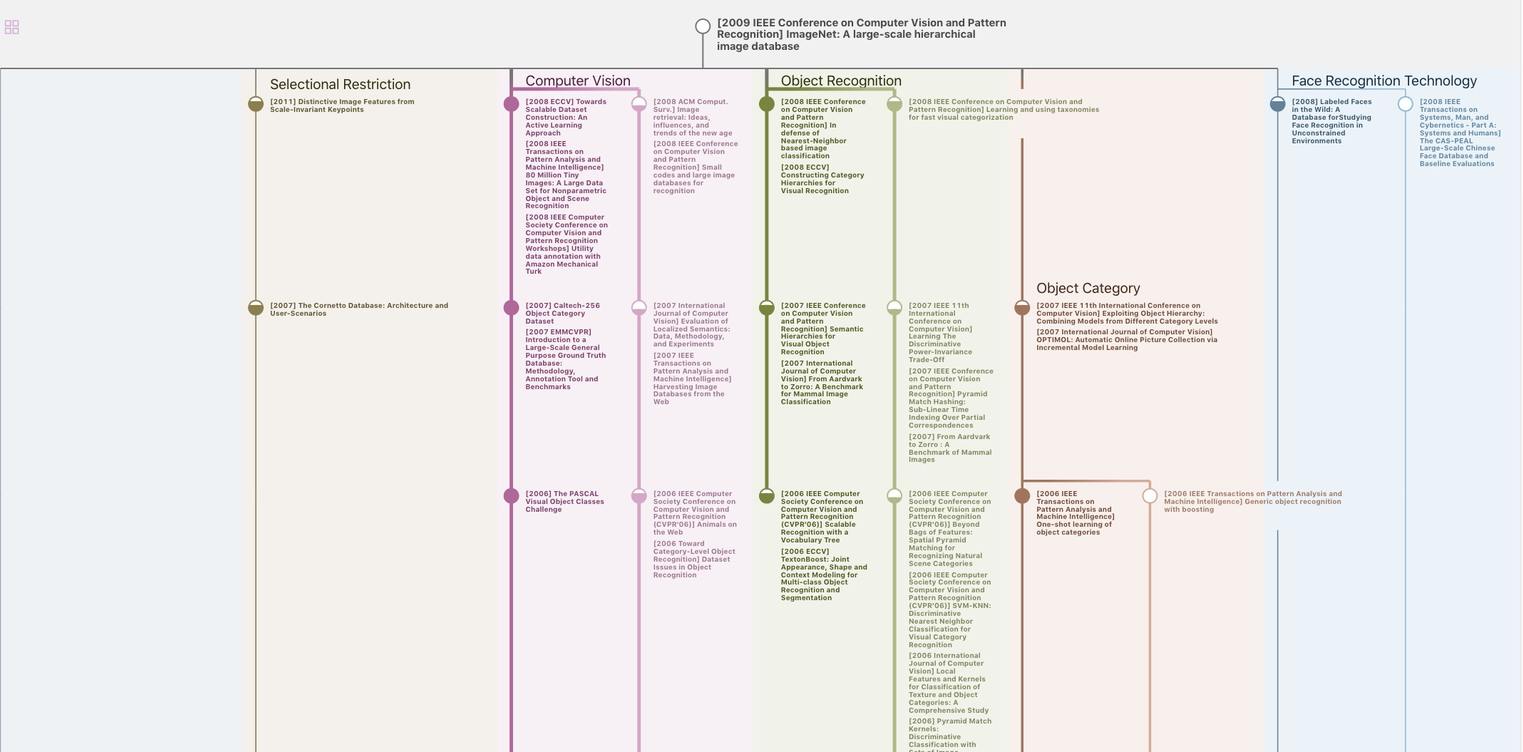
生成溯源树,研究论文发展脉络
Chat Paper
正在生成论文摘要