Overhead Reduction in UAV-Assisted Federated Learning with Fast-Varying Environment
WSA & SCC 2023; 26th International ITG Workshop on Smart Antennas and 13th Conference on Systems, Communications, and Coding(2023)
摘要
Thanks to its communication efficiency, low latency, and privacy-preserving nature, federated learning (FL) has emerged as a promising learning paradigm in the unmanned aerial vehicle (UAV)-enabled networks, where the UAVs support various applications. Despite its great potential, FL over a wireless network suffers from excessive communication overhead, particularly when the data distribution changes frequently. This drawback, which results from the necessity of frequent model updates, degrades the network performance severely. To address this challenge, we propose two methods based on offline and online Bayesian changepoint detection. In such approaches, the FL mechanism is triggered only upon observing abrupt changes in data distribution at a sufficiently large number of clients that can severely degrade the model accuracy. Our results show that both approaches yield near-optimal FL performance while significantly reducing the overhead.
更多查看译文
AI 理解论文
溯源树
样例
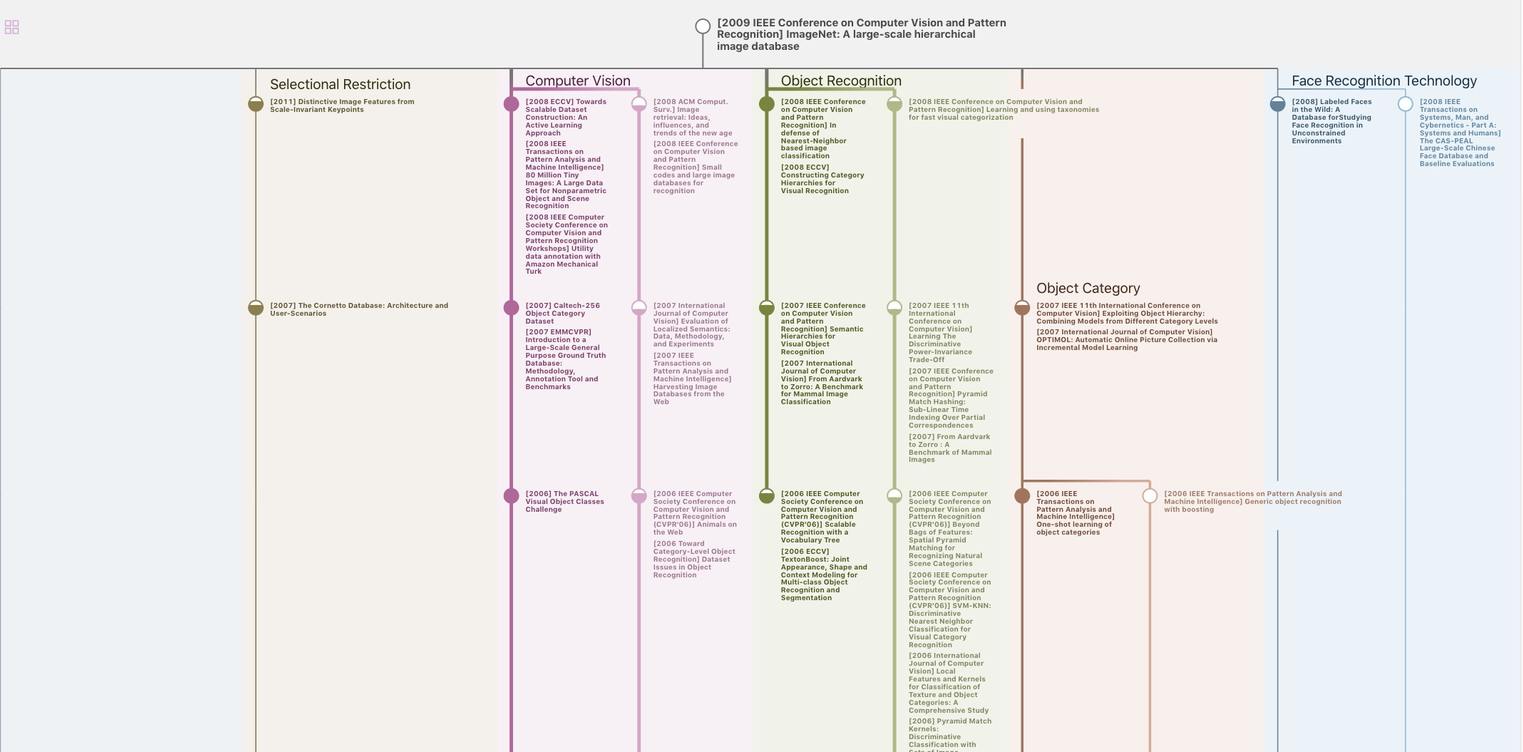
生成溯源树,研究论文发展脉络
Chat Paper
正在生成论文摘要