Quantifying the stochasticity of policy parameters in reinforcement learning problems.
Physical review. E(2023)
摘要
The stochastic dynamics of reinforcement learning is studied using a master equation formalism. We consider two different problems-Q learning for a two-agent game and the multiarmed bandit problem with policy gradient as the learning method. The master equation is constructed by introducing a probability distribution over continuous policy parameters or over both continuous policy parameters and discrete state variables (a more advanced case). We use a version of the moment closure approximation to solve for the stochastic dynamics of the models. Our method gives accurate estimates for the mean and the (co)variance of policy variables. For the case of the two-agent game, we find that the variance terms are finite at steady state and derive a system of algebraic equations for computing them directly.
更多查看译文
关键词
reinforcement learning,policy parameters,stochasticity
AI 理解论文
溯源树
样例
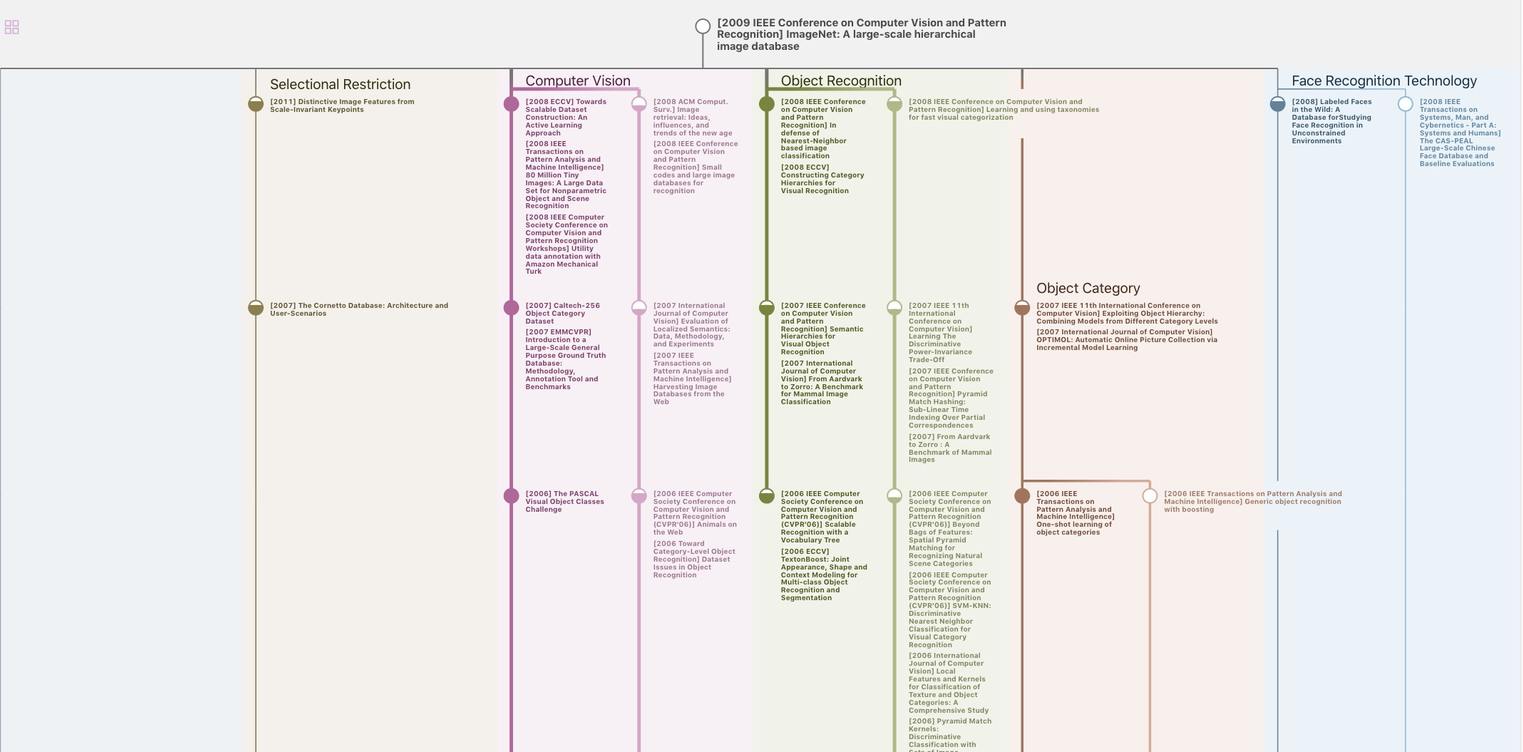
生成溯源树,研究论文发展脉络
Chat Paper
正在生成论文摘要