Learnable Earth Parser: Discovering 3D Prototypes in Aerial Scans
arXiv (Cornell University)(2023)
摘要
We propose an unsupervised method for parsing large 3D scans of real-world scenes into interpretable parts. Our goal is to provide a practical tool for analyzing 3D scenes with unique characteristics in the context of aerial surveying and mapping, without relying on application-specific user annotations. Our approach is based on a probabilistic reconstruction model that decomposes an input 3D point cloud into a small set of learned prototypical shapes. Our model provides an interpretable reconstruction of complex scenes and leads to relevant instance and semantic segmentations. To demonstrate the usefulness of our results, we introduce a novel dataset of seven diverse aerial LiDAR scans. We show that our method outperforms state-of-the-art unsupervised methods in terms of decomposition accuracy while remaining visually interpretable. Our method offers significant advantage over existing approaches, as it does not require any manual annotations, making it a practical and efficient tool for 3D scene analysis. Our code and dataset are available at https://imagine.enpc.fr/~loiseaur/learnable-earth-parser
更多查看译文
关键词
learnable earth parser,3d prototypes
AI 理解论文
溯源树
样例
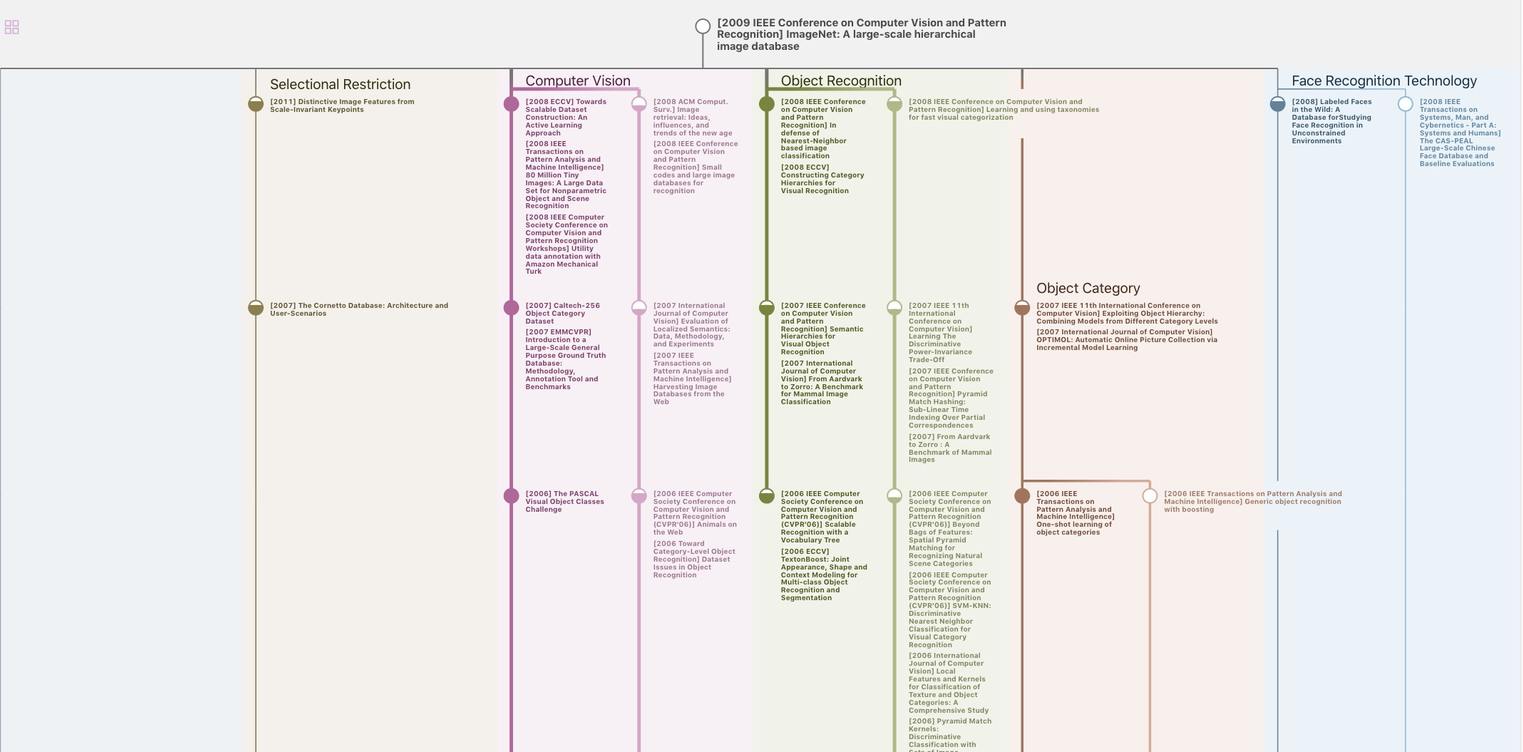
生成溯源树,研究论文发展脉络
Chat Paper
正在生成论文摘要