Decadal Temperature Prediction via Chaotic Behavior Tracking
CoRR(2023)
摘要
Decadal temperature prediction provides crucial information for quantifying the expected effects of future climate changes and thus informs strategic planning and decision-making in various domains. However, such long-term predictions are extremely challenging, due to the chaotic nature of temperature variations. Moreover, the usefulness of existing simulation-based and machine learning-based methods for this task is limited because initial simulation or prediction errors increase exponentially over time. To address this challenging task, we devise a novel prediction method involving an information tracking mechanism that aims to track and adapt to changes in temperature dynamics during the prediction phase by providing probabilistic feedback on the prediction error of the next step based on the current prediction. We integrate this information tracking mechanism, which can be considered as a model calibrator, into the objective function of our method to obtain the corrections needed to avoid error accumulation. Our results show the ability of our method to accurately predict global land-surface temperatures over a decadal range. Furthermore, we demonstrate that our results are meaningful in a real-world context: the temperatures predicted using our method are consistent with and can be used to explain the well-known teleconnections within and between different continents.
更多查看译文
关键词
decadal temperature prediction
AI 理解论文
溯源树
样例
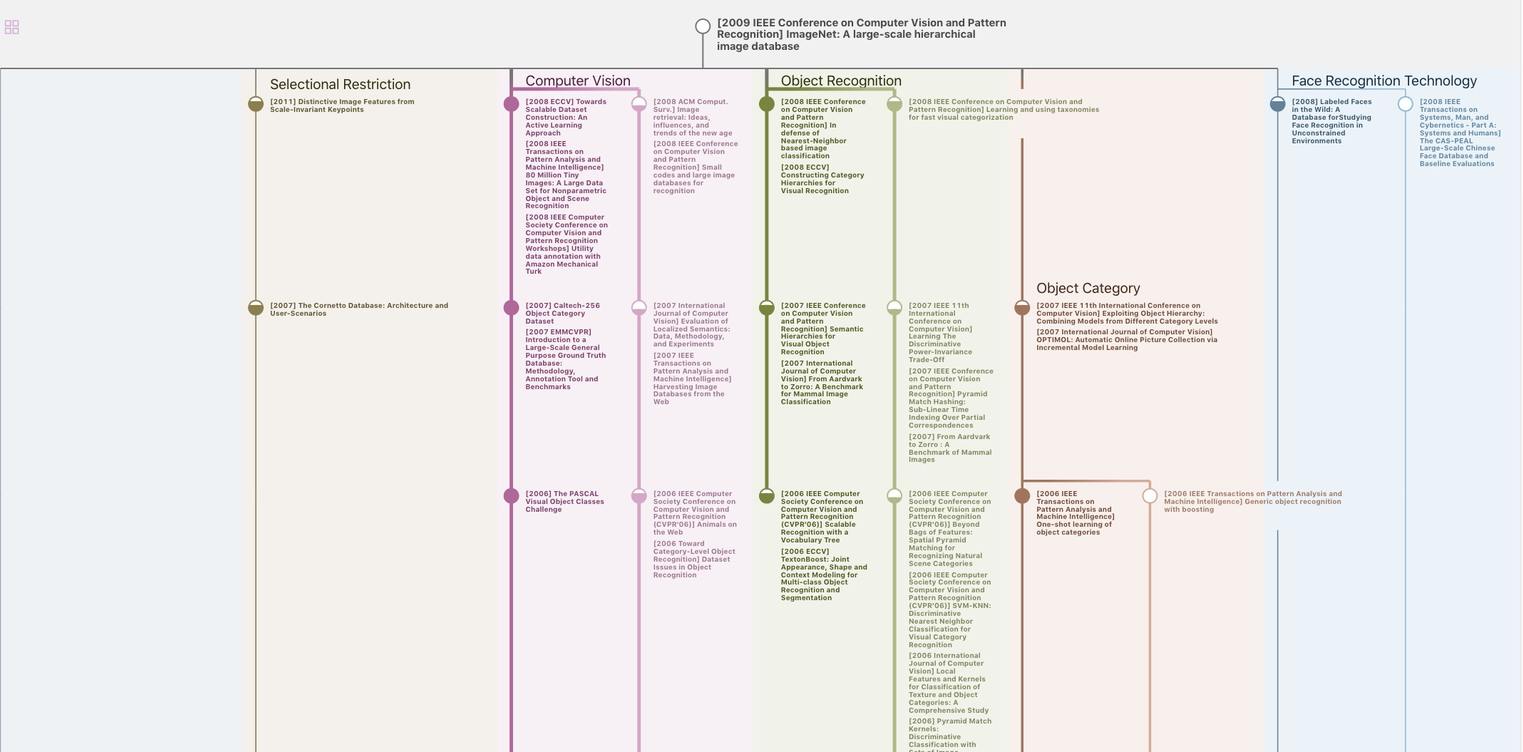
生成溯源树,研究论文发展脉络
Chat Paper
正在生成论文摘要