Network Pruning Spaces
CoRR(2023)
摘要
Network pruning techniques, including weight pruning and filter pruning, reveal that most state-of-the-art neural networks can be accelerated without a significant performance drop. This work focuses on filter pruning which enables accelerated inference with any off-the-shelf deep learning library and hardware. We propose the concept of \emph{network pruning spaces} that parametrize populations of subnetwork architectures. Based on this concept, we explore the structure aspect of subnetworks that result in minimal loss of accuracy in different pruning regimes and arrive at a series of observations by comparing subnetwork distributions. We conjecture through empirical studies that there exists an optimal FLOPs-to-parameter-bucket ratio related to the design of original network in a pruning regime. Statistically, the structure of a winning subnetwork guarantees an approximately optimal ratio in this regime. Upon our conjectures, we further refine the initial pruning space to reduce the cost of searching a good subnetwork architecture. Our experimental results on ImageNet show that the subnetwork we found is superior to those from the state-of-the-art pruning methods under comparable FLOPs.
更多查看译文
关键词
network,spaces
AI 理解论文
溯源树
样例
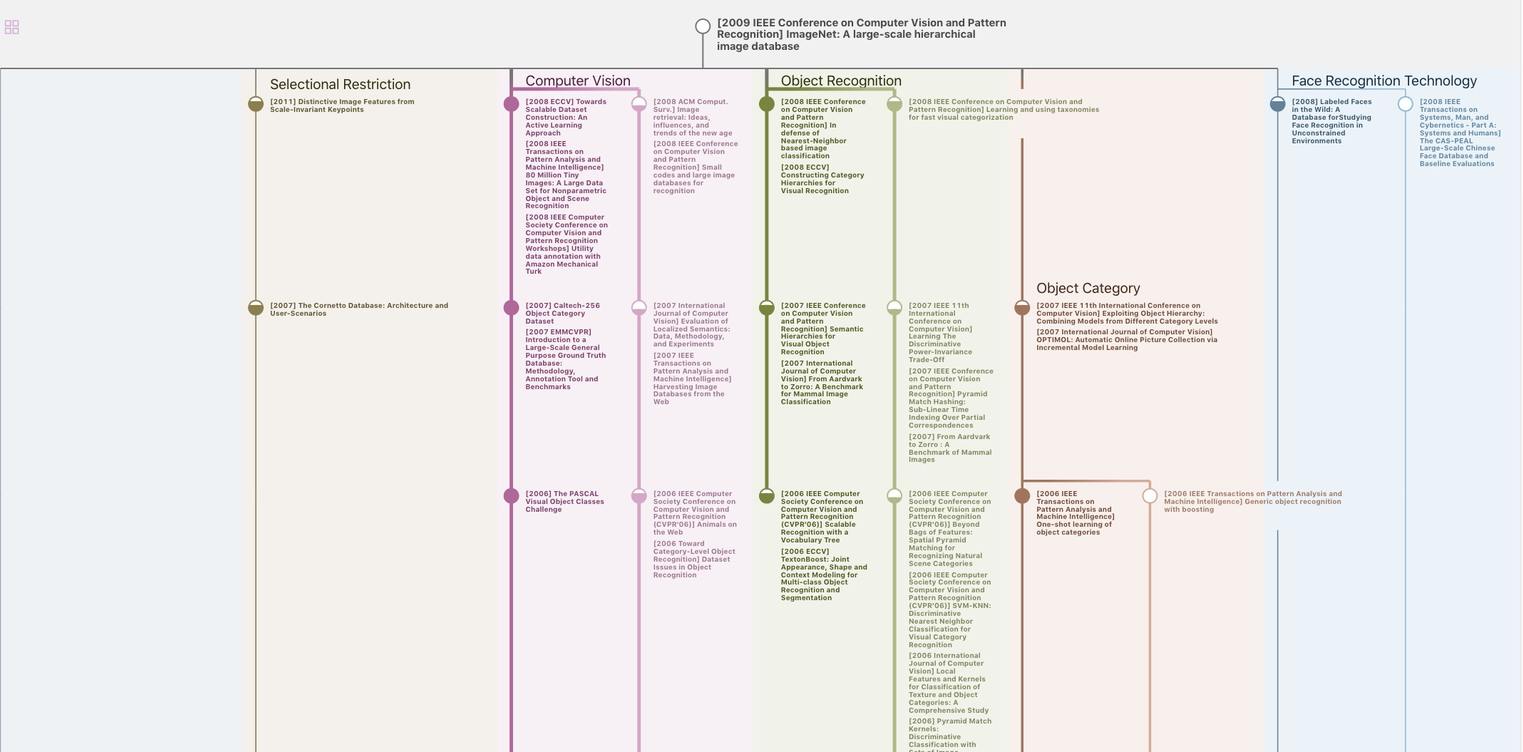
生成溯源树,研究论文发展脉络
Chat Paper
正在生成论文摘要