Constructing a Simulation Surrogate with Partially Observed Output
TECHNOMETRICS(2024)
摘要
Gaussian process surrogates are a popular alternative to directly using computationally expensive simulation models. When the simulation output consists of many responses, dimension-reduction techniques are often employed to construct these surrogates. However, surrogate methods with dimension reduction generally rely on complete output training data. This article proposes a new Gaussian process surrogate method that permits the use of partially observed output while remaining computationally efficient. The new method involves the imputation of missing values and the adjustment of the covariance matrix used for Gaussian process inference. The resulting surrogate represents the available responses, disregards the missing responses, and provides meaningful uncertainty quantification. The proposed approach is shown to offer sharper inference than alternatives in a simulation study and a case study where an energy density functional model that frequently returns incomplete output is calibrated.
更多查看译文
关键词
Calibration,Gaussian process,High-dimensional output,Missing data,Statistical emulation
AI 理解论文
溯源树
样例
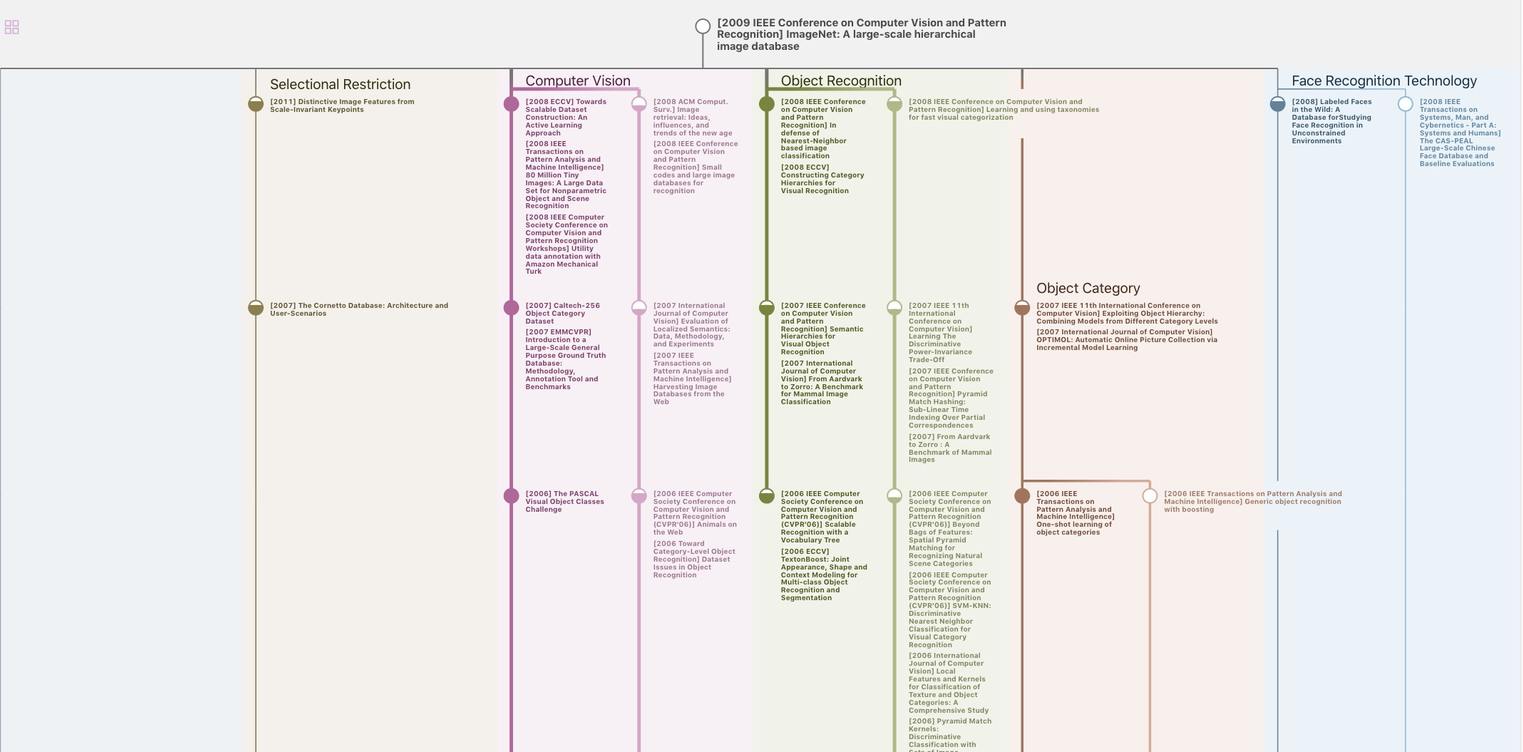
生成溯源树,研究论文发展脉络
Chat Paper
正在生成论文摘要