New Subset Selection Algorithms for Low Rank Approximation: Offline and Online
PROCEEDINGS OF THE 55TH ANNUAL ACM SYMPOSIUM ON THEORY OF COMPUTING, STOC 2023(2023)
摘要
Subset selection for the rank : approximation of an n x d matrix A offlers improvements in the interpretability of matrices, as well as a variety of computational savings. This problem is well-understood when the error measure is the Frobenius norm, with various tight algorithms known even in challenging models such as the online model, where an algorithm must select the column subset irrevocably when the columns arrive one by one. In sharp contrast, when the error measure is replaced by other matrix losses, optimal tradeoffls between the subset size and approximation quality have not been settled, even in the standard offline setting. We give a number of results towards closing these gaps. In the offline setting, we achieve nearly optimal bicriteria algorithms in two settings. First, we remove a v : factor from a prior result of Song-Woodruffl-Zhong when the loss function is any entrywise loss with an approximate triangle inequality and at least linear growth, which includes, e.g., the Huber loss. Our result is tight when applied to the l(p) loss. We give a similar improvement for the entrywise l(p) loss for root k> 2, improving a previous distortion of (O) over tilde (k(1-1/p)) to O (k(1/2-1/p)). We show this is tight for p = infinity, while for 2 < ? < 8, we give the fflrst bicriteria algorithms for ( 1 + Y)-approximate entrywise ffl? low rank approximation. Our results come from a general technique which improves distortions by replacing the use of a well-conditioned basis with a slightly larger spanning set for which any vector can be expressed as a linear combination with small Euclidean norm. This idea may be of independent interest and we show, for example, that it also gives the fflrst oblivious lp subspace embeddings for 1 = l < 2 with lp (31/?) distortion, which is nearly optimal and improves the previously best known lp (3) and closes a long line of work. In the online setting, we give the fflrst online subset selection algorithm for lp subspace approximation and entrywise ffl? low rank approximation by showing how to implement the classical sensitivity sampling algorithm online, which is challenging due to the sequential nature of sensitivity sampling. Our main technique is an online algorithm for detecting when an approximately optimal subspace changes substantially. We also give new related results for the online setting, including online coresets for Euclidean (k,p) clustering as well as an online active regression algorithm making Theta (d(p/2)/epsilon(p-1)) queries, answering open questions of Musco-MuscoWoodruffl-Yasuda and Chen-Li-Sun.
更多查看译文
关键词
low rank approximation,subset selection,oblivious subspace embeddings
AI 理解论文
溯源树
样例
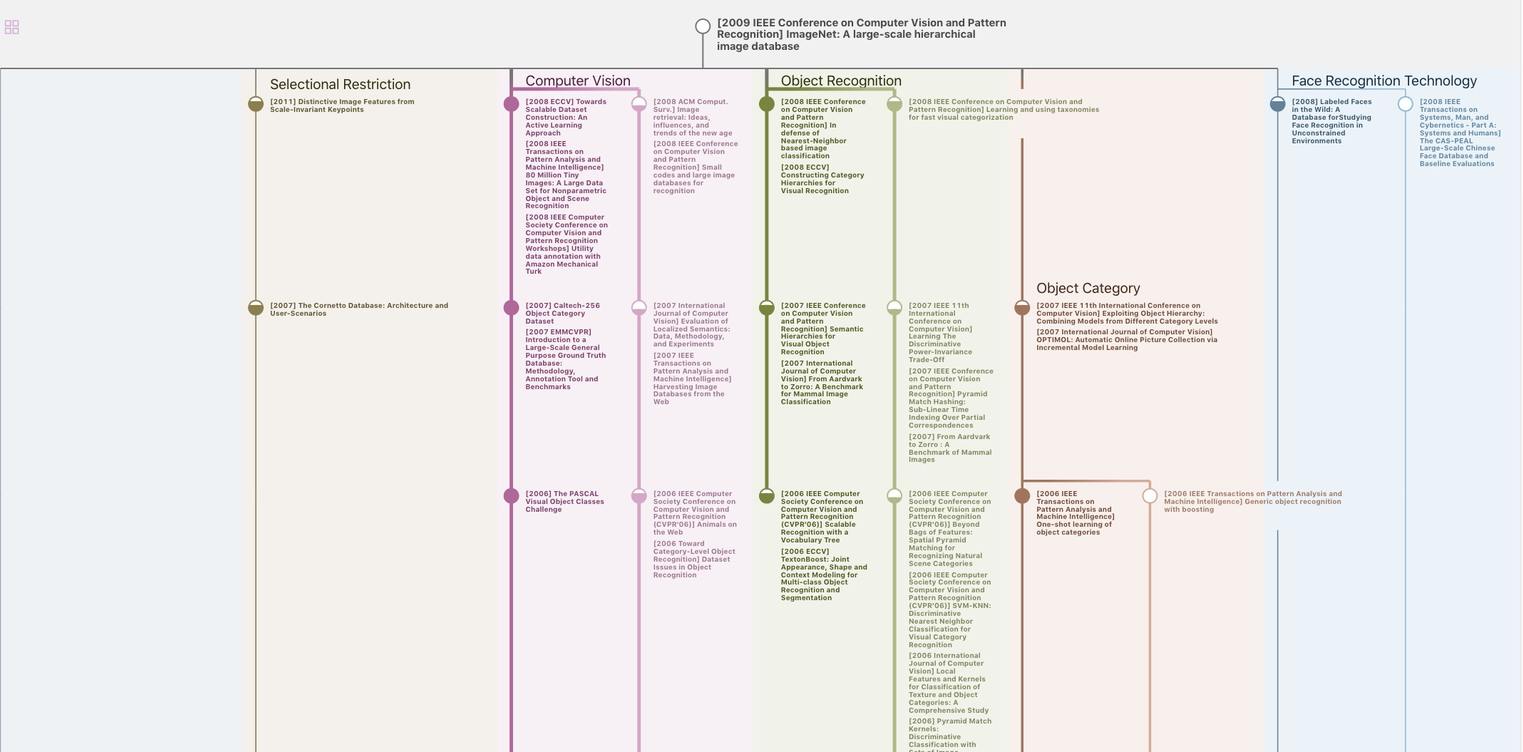
生成溯源树,研究论文发展脉络
Chat Paper
正在生成论文摘要