Dual Autoencoder Enhanced Subgraph Pattern Mining for Cognitive Diagnosis
2022 IEEE 34th International Conference on Tools with Artificial Intelligence (ICTAI)(2022)
摘要
In adaptive learning, Cognitive diagnosis aims to discover students' knowledge state on different knowledge con-cepts and predict their future performance. Most previous methods consider more on students' own answering history and rarely model the the impact brought by students with similar answering behaviors explicitly. This collaborative information among students is helpful for students who lack sufficient historical logs. In this paper, we propose a new cognitive diagnosis method called Dual Autoencoder Enhanced Subgraph Pattern Mining(DASPM) for Cognitive Diagnosis, which incorporates collaborative information among students into the cognitive di-agnosis process to obtain more accurate predictions. Specifically, we use a graph neural network to capture collaborative pattern on the student-exercise bipartite graph. In order to filter out the interference of irrelevant information, we design a sub graph extraction algorithm that separates local parts around the target student-exercise pair from global graph based on the correlation between exercises. In addition, we utilize a dual autoencoder module to encode students and exercises to enhance the initial representation of nodes in the sub graph. Extensive experiments on multiple datasets show the effectiveness of our proposed method.
更多查看译文
关键词
Cognitive Diagnosis,Adaptive Learning System,Student Performance Prediction,Autoencoder,Graph Neural Network
AI 理解论文
溯源树
样例
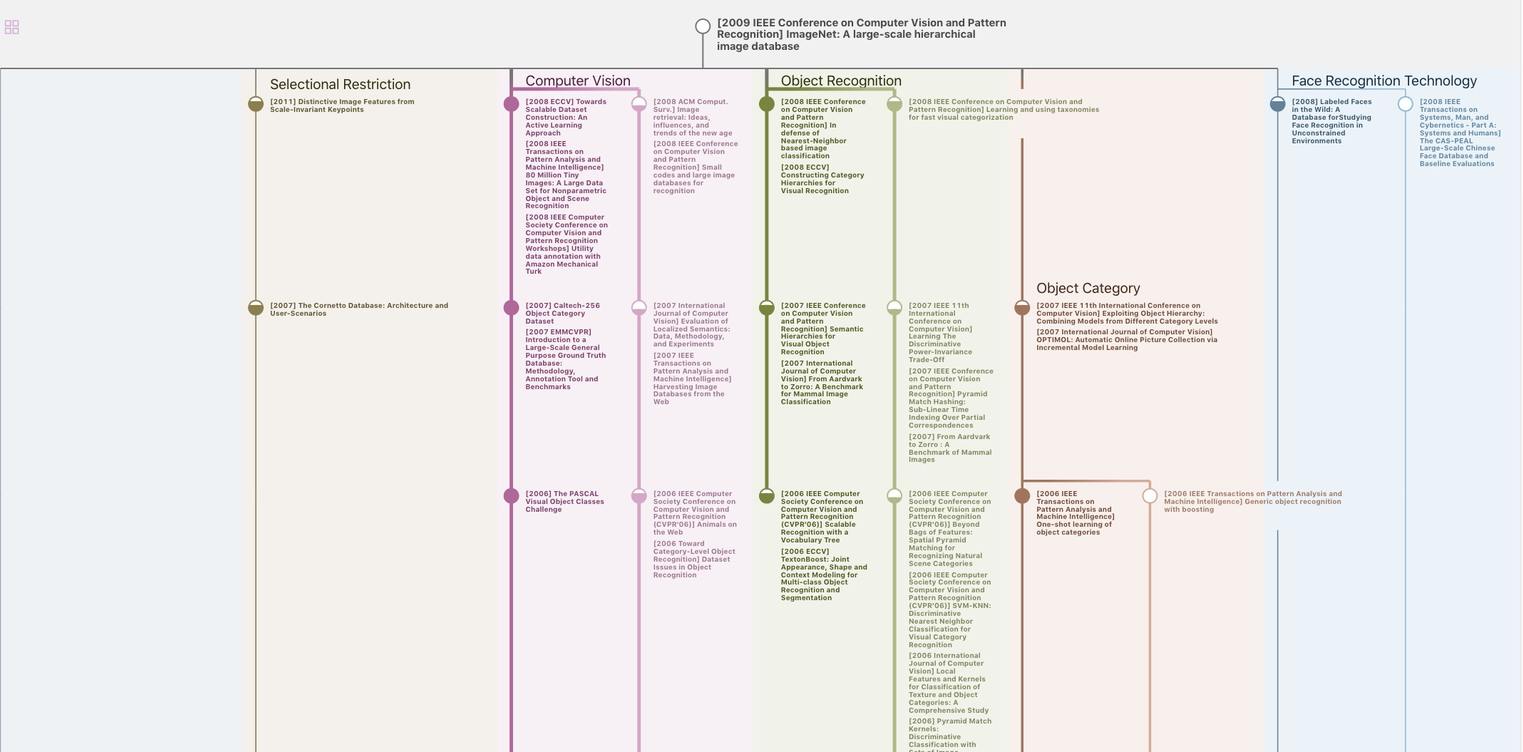
生成溯源树,研究论文发展脉络
Chat Paper
正在生成论文摘要