Knowledge Discovery from Qualitative Spatial and Temporal Data
2022 IEEE 34th International Conference on Tools with Artificial Intelligence (ICTAI)(2022)
摘要
Qualitative reasoning formalisms facilitate the representation and interpretation of information involving complex entities. We use in this paper qualitative spatial and temporal reasoning to introduce novel data mining tasks, which consist in extracting knowledge from quantitative databases that are trans-formed into collections of qualitative relation networks (QRNs). After describing our qualitative data mining framework, we first propose an Apriori-like algorithm that exploits monotonicity and QRN consistency for pruning the search space: the validity of a pattern candidate depends on the supports of the larger patterns that include it and on its consistency. We then introduce an encoding of our data mining tasks into the well-known problem of frequent itemset mining. We finally show the feasibility of our approach by providing preliminary experimental results using real-world datasets about the movements of football players during matches.
更多查看译文
关键词
qualitative reasoning,knowledge discovery,data mining
AI 理解论文
溯源树
样例
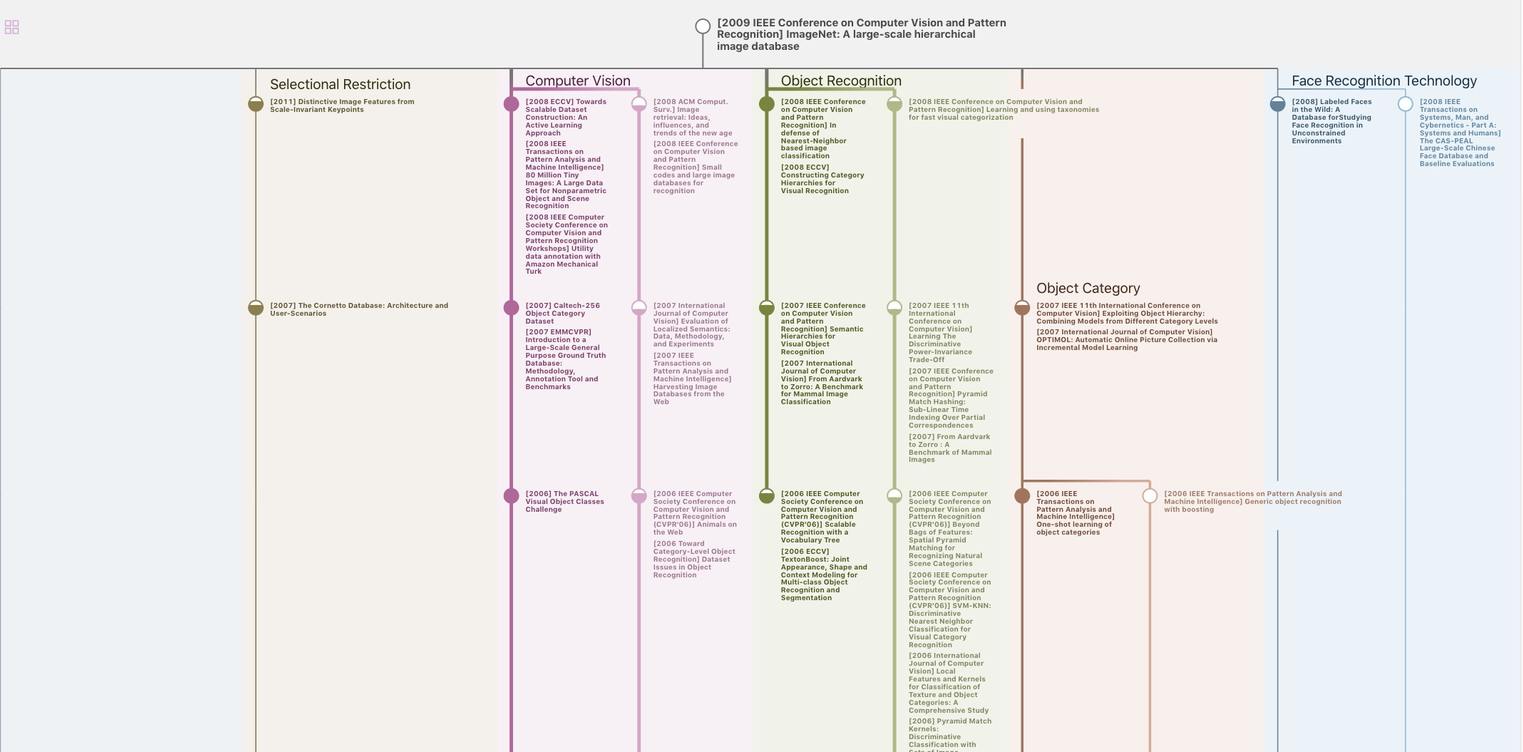
生成溯源树,研究论文发展脉络
Chat Paper
正在生成论文摘要