A novel human learning optimization algorithm with Bayesian inference learning
Knowledge-Based Systems(2023)
摘要
Humans perform Bayesian inference in a wide variety of tasks, which can help people make selection decisions effectively and therefore enhances learning efficiency and accuracy. Inspired by this fact, this paper presents a novel human learning optimization algorithm with Bayesian inference learning (HLOBIL), in which a Bayesian inference learning operator (BILO) is developed to utilize the inference strategy for enhancing learning efficiency. The in-depth analysis shows that the proposed BILO can efficiently improve the exploitation ability of the algorithm as it can achieve the optimal values and retrieve the optimal information with the accumulated search information. Besides, the exploration ability of HLOBIL is also strengthened by the inborn characteristics of Bayesian inference. The experimental results demonstrate that the developed HLOBIL is superior to previous HLO variants and other state-of-art algorithms with its improved exploitation and exploration abilities.
更多查看译文
关键词
Human learning optimization, Meta-heuristic, Bayesian inference, Bayesian inference learning, Individual learning, Social learning
AI 理解论文
溯源树
样例
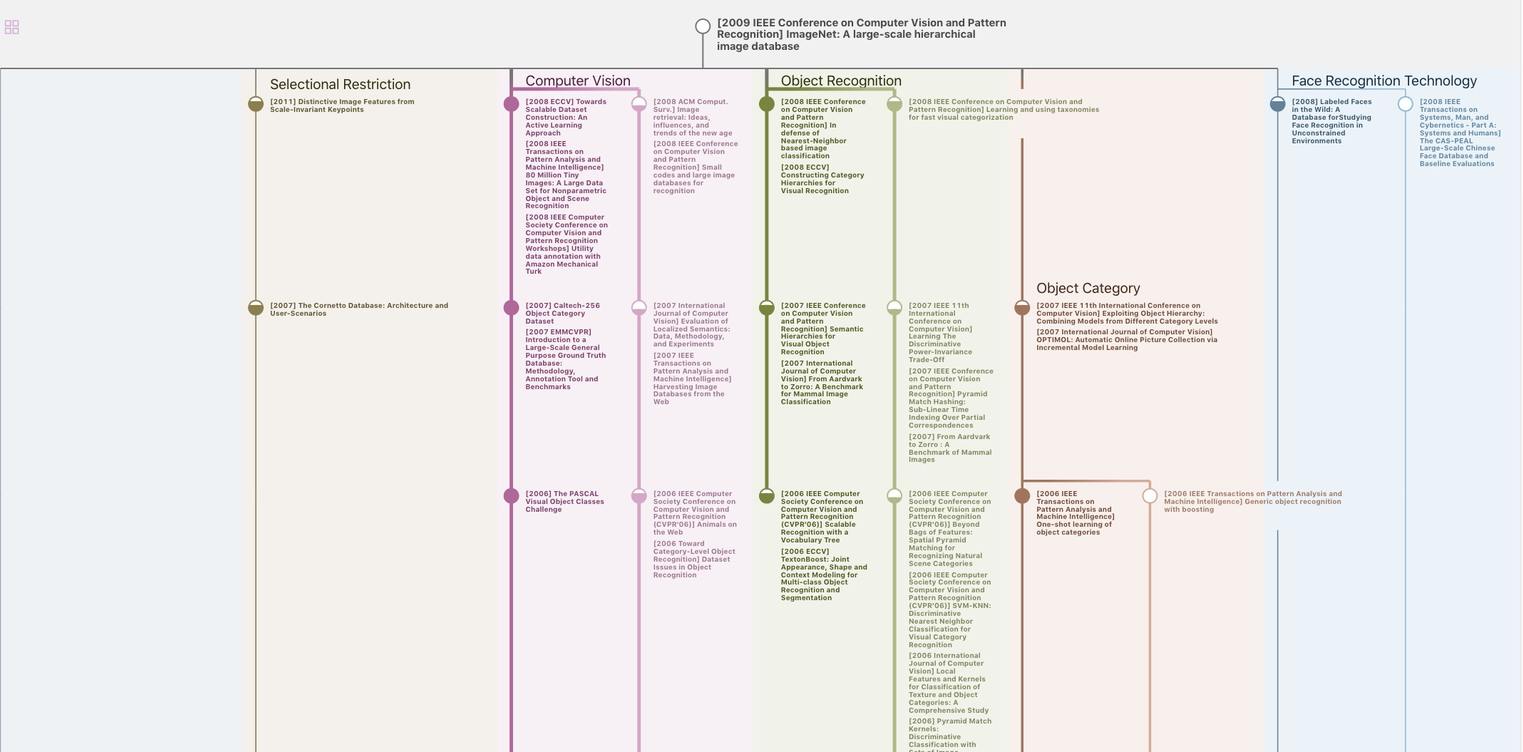
生成溯源树,研究论文发展脉络
Chat Paper
正在生成论文摘要