Grounding Classical Task Planners via Vision-Language Models
CoRR(2023)
摘要
Classical planning systems have shown great advances in utilizing rule-based human knowledge to compute accurate plans for service robots, but they face challenges due to the strong assumptions of perfect perception and action executions. To tackle these challenges, one solution is to connect the symbolic states and actions generated by classical planners to the robot's sensory observations, thus closing the perception-action loop. This research proposes a visually-grounded planning framework, named TPVQA, which leverages Vision-Language Models (VLMs) to detect action failures and verify action affordances towards enabling successful plan execution. Results from quantitative experiments show that TPVQA surpasses competitive baselines from previous studies in task completion rate.
更多查看译文
关键词
classical task planners,vision-language
AI 理解论文
溯源树
样例
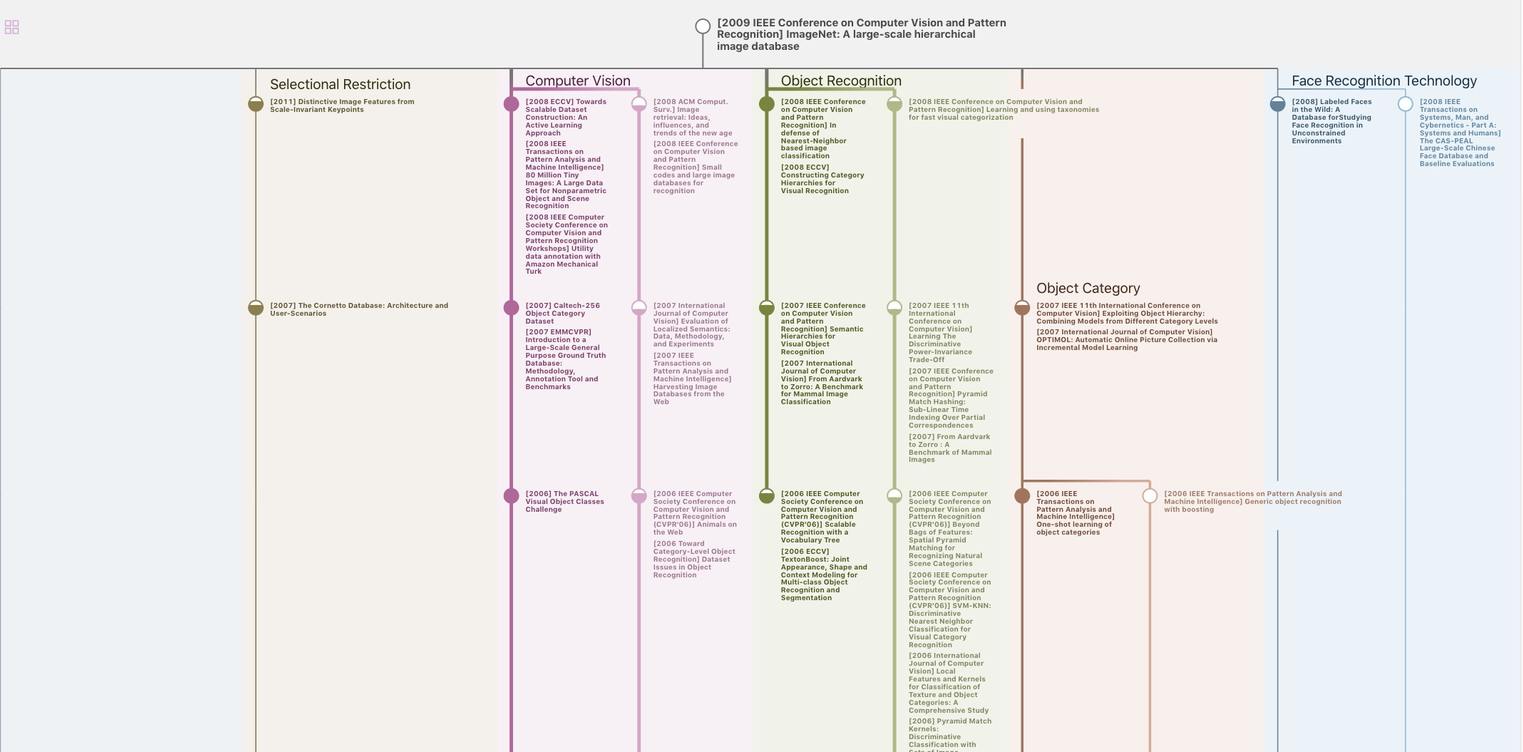
生成溯源树,研究论文发展脉络
Chat Paper
正在生成论文摘要