Predictive performance of random forest on the identification of mangrove species in arid environments
Ecological Informatics(2023)
摘要
Machine learning methods are the most popular approaches for carrying out classification in remote sensing studies. Of the methods available, random forest (RF) is the one most often used due to its high predictive performance. The objective of this study was to assess the predictive performance of RF in identifying (classifying) mangrove species in an arid environment using two cameras: one conventional (visible part of the light, RGB), the other specialized (Green, Red, Near-infrared, GRN). The RGB and GRN bands were used with derived vegetation indexes (for each camera), the canopy height model (derived from photogrammetry), and distance to water (derived from raster analysis) to classify the study area in eight classes (including three mangrove species) using RF. Results suggest only slight differences in predictive performance (validation) between the products derived from the GRN and RGB cameras, the accuracy values ranged from 0.58 to 0.77 and from 0.53 to 0.72 for RGB and GRN, respectively. The most important variables were the distance to water and canopy height model for both cameras, followed by specific bands and vegetation indices. The study concludes that conventional cameras mounted in commercial drones can be used efficiently to identify mangrove species in arid environments when the classification model uses physical variables of the species (tree height) and the system (distance to water). Results of this study can be applied to describe spatial distributions by species in small or large patches of mangroves in arid environments, thus improving our ecological knowledge of this ecosystem.
更多查看译文
关键词
Phantom 4,Red-green-blue,Near-infrared,Distance to water,Canopy height model
AI 理解论文
溯源树
样例
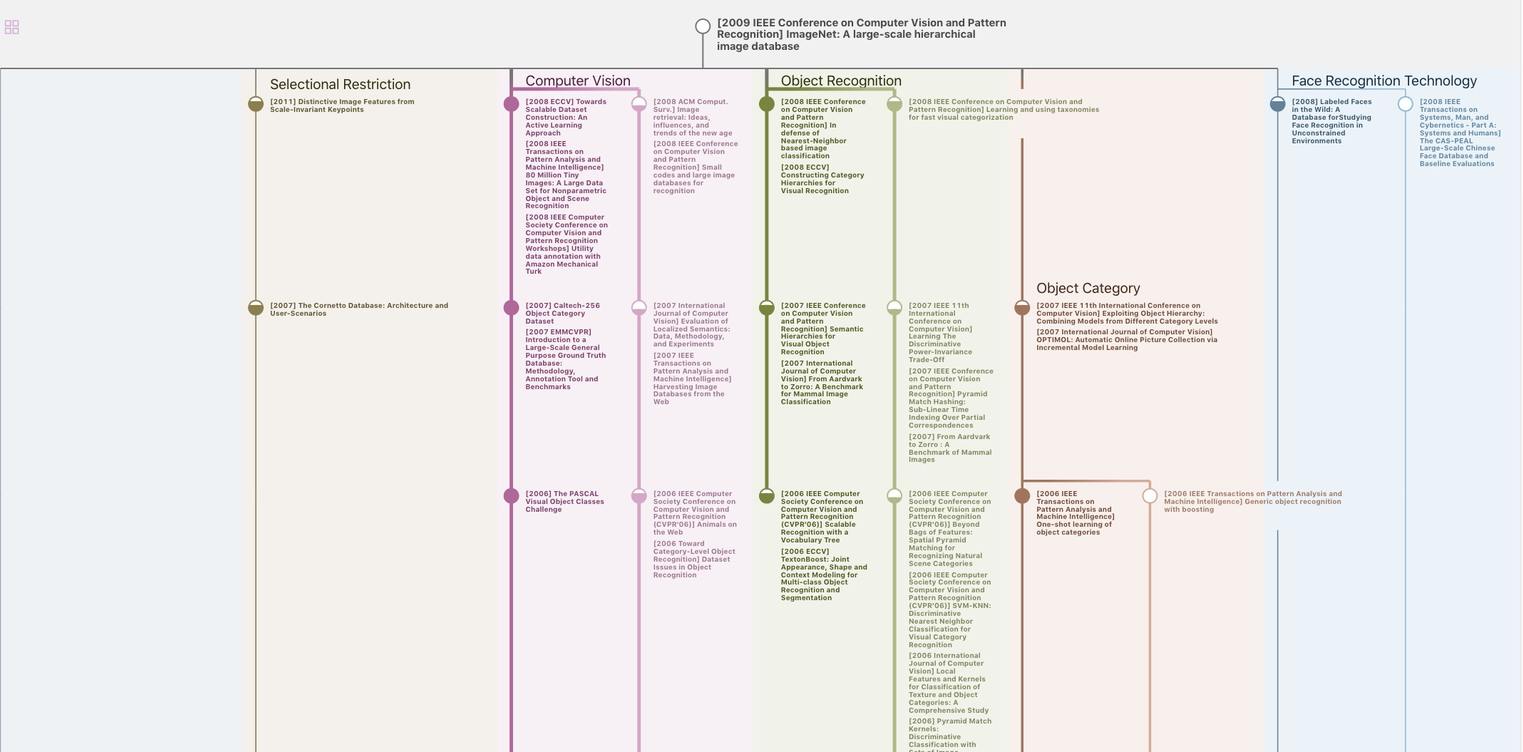
生成溯源树,研究论文发展脉络
Chat Paper
正在生成论文摘要