Neuromorphic deep spiking neural networks for seizure detection
Neuromorphic Computing and Engineering(2023)
摘要
The vast majority of studies that process and analyze neural signals are conducted on cloud computing resources, which is often necessary for the demanding requirements of deep neural network workloads. However, applications such as epileptic seizure detection stand to benefit from edge devices that can securely analyze sensitive medical data in a real-time and personalised manner. In this work, we propose a novel neuromorphic computing approach to seizure detection using a surrogate gradient-based deep spiking neural network (SNN), which consists of a novel spiking ConvLSTM unit. We have trained, validated, and rigorously tested the proposed SNN model across three publicly accessible datasets, including Boston Children's Hospital-MIT (CHB-MIT) dataset from the U.S., and the Freiburg (FB) and EPILEPSIAE intracranial electroencephalogram datasets from Germany. The average leave-one-out cross-validation area under the curve score for FB, CHB-MIT and EPILEPSIAE datasets can reach 92.7 % , 89.0 % , and 81.1 % , respectively, while the computational overhead and energy consumption are significantly reduced when compared to alternative state-of-the-art models, showing the potential for building an accurate hardware-friendly, low-power neuromorphic system. This is the first feasibility study using a deep SNN for seizure detection on several reliable public datasets.
更多查看译文
关键词
deep spiking neural network,seizure detection,neuromorphic computing
AI 理解论文
溯源树
样例
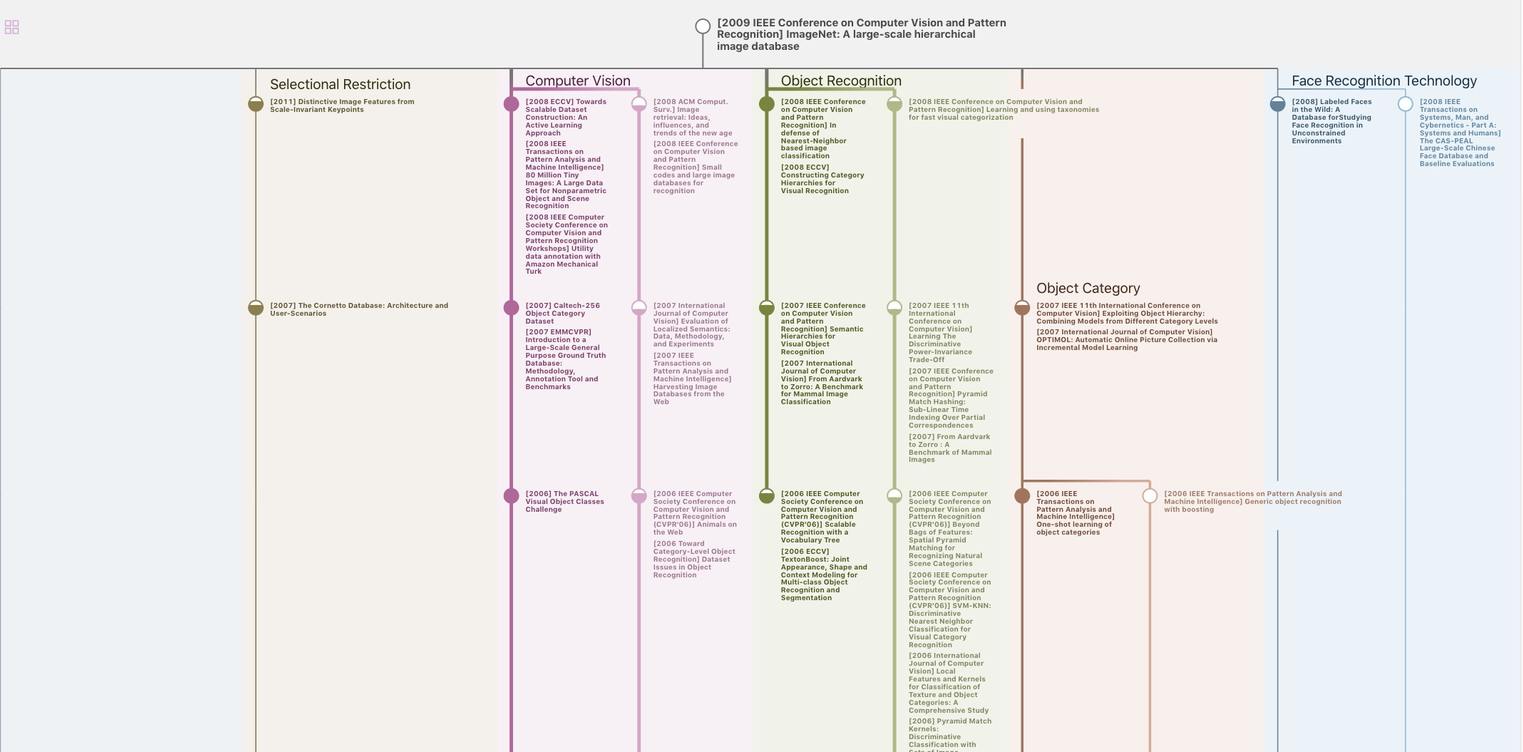
生成溯源树,研究论文发展脉络
Chat Paper
正在生成论文摘要