Automatic detection of actual water depth of urban floods from social media images
Measurement(2023)
摘要
Urban flooding disasters are the most frequent natural disasters in cities, which broadly affects people's livelihood. Prompt emergency response is the key to alleviating such impact, however, that would rely on the timely mapping of flooding according to waterlogging severity. Compared with traditional approaches, crowdsourcing images from social media has emerged as a more efficient way to obtain this type of information. Therefore, this paper aims to explore the approach based on computer vision technology to automatically extract water depth from social media images, which can be utilized to construct flooding maps during urban flooding. Firstly, the images related to urban flooding were retrieved from social media and then filtered, leaving only images containing people. Secondly, a specific objective detection model based on YOLO was trained to detect the human body parts which are divided into crus, thigh, shoulder, and head from bottom to top. The experiment shows that the mAP of the trained human body parts detection model in the test dataset is 0.967. Afterward, an algorithm for extracting water depth was proposed based on the ratio of bounding boxes of detected body parts in images to the actual length of human body parts. Next, these models were verified by comparing them with manually estimated depth range and manually measured depth. The experiment shows that the accuracy of water depth range is 0.959 and the mean absolute error of the actual depth detection of water is 10.22 cm in the measurement dataset. Finally, the proposed models were applied to map the 2016 flooding that occurred in Wuhan as an illustrative case. This approach is helpful to broaden the source of flooding severity information and improve the efficiency of flood mapping in urban flood emergency management.
更多查看译文
关键词
Automatic detection,Actual water depth,Urban flood,Social media,Object detection,YOLO
AI 理解论文
溯源树
样例
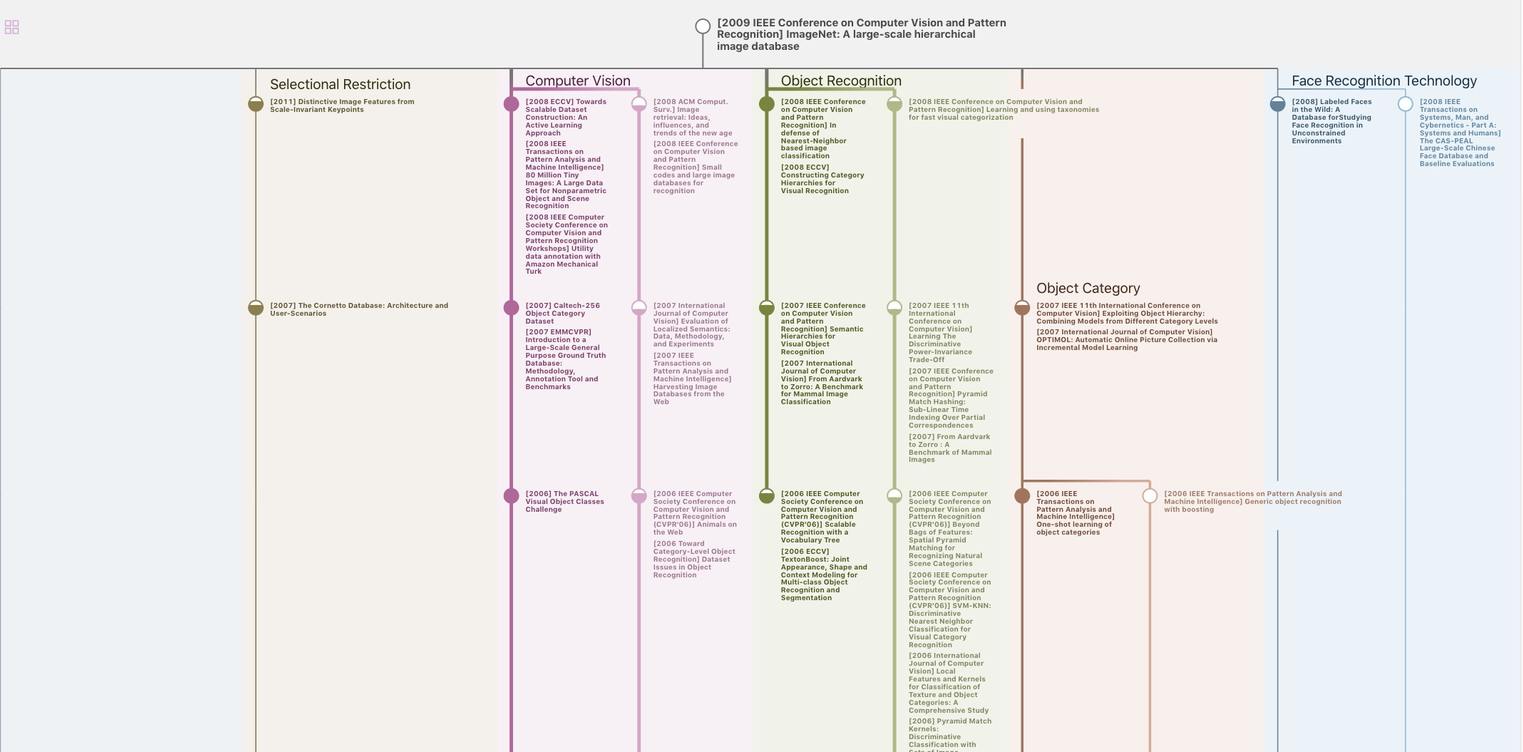
生成溯源树,研究论文发展脉络
Chat Paper
正在生成论文摘要