Do we need early exit networks in human activity recognition?
Engineering Applications of Artificial Intelligence(2023)
摘要
Deep learning is nowadays considered state-of-the-art technology in many applications thanks to huge perfor-mance capabilities. However, the accuracy levels that can be obtained with these models entail computationally demanding resources. This results in a challenging task when such systems have to be deployed on edge devices with tight computing, memory, and communication requirements and when energy expenditure and inference delays have to be kept under control. Early exit is a design methodology aimed at reducing the burden of neural networks on computational resources, trading off accuracy for latency.In this work, we aim at exploring the use of early exit for human activity recognition tasks. In particular, we propose an experimental assessment of the accuracy-latency trade-off on different deep network architectures across various publicly available datasets. We also evaluate the impact of early exiting in distributed environments by taking into account communication technologies.Experimental results provide evidence of the significant gain provided by early exits in terms of latency (up to 35x), without a reduction in accuracy (in most cases), confirming the viability of an adaptive approach. In a distributed environment, early exit results are not beneficial in all situations. In particular, it is not convenient for models that are very fast (with inference latency lower than, or as equal as, that of communication) and for models that are forced to make extensive use of far exit points to satisfy the accuracy requirements. Therefore, communication delays in a distributed environment shape performance in an architecture-dependent way.
更多查看译文
关键词
Deep learning,Early exit,Human activity recognition
AI 理解论文
溯源树
样例
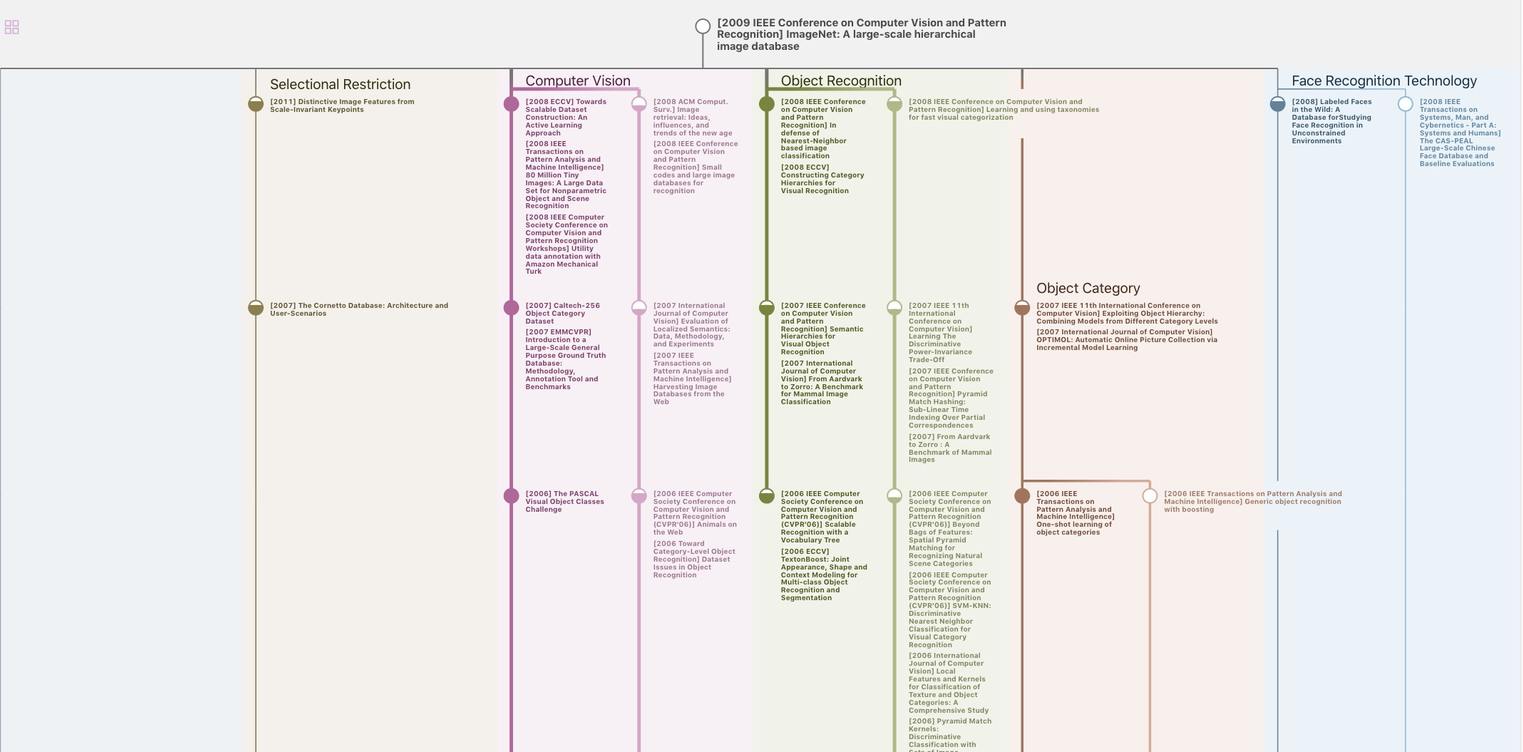
生成溯源树,研究论文发展脉络
Chat Paper
正在生成论文摘要