InstructUIE: Multi-task Instruction Tuning for Unified Information Extraction
CoRR(2023)
摘要
Large language models have unlocked strong multi-task capabilities from reading instructive prompts. However, recent studies have shown that existing large models still have difficulty with information extraction tasks. For example, gpt-3.5-turbo achieved an F1 score of 18.22 on the Ontonotes dataset, which is significantly lower than the state-of-the-art performance. In this paper, we propose InstructUIE, a unified information extraction framework based on instruction tuning, which can uniformly model various information extraction tasks and capture the inter-task dependency. To validate the proposed method, we introduce IE INSTRUCTIONS, a benchmark of 32 diverse information extraction datasets in a unified text-to-text format with expert-written instructions. Experimental results demonstrate that our method achieves comparable performance to Bert in supervised settings and significantly outperforms the state-of-the-art and gpt3.5 in zero-shot settings.
更多查看译文
关键词
unified information
AI 理解论文
溯源树
样例
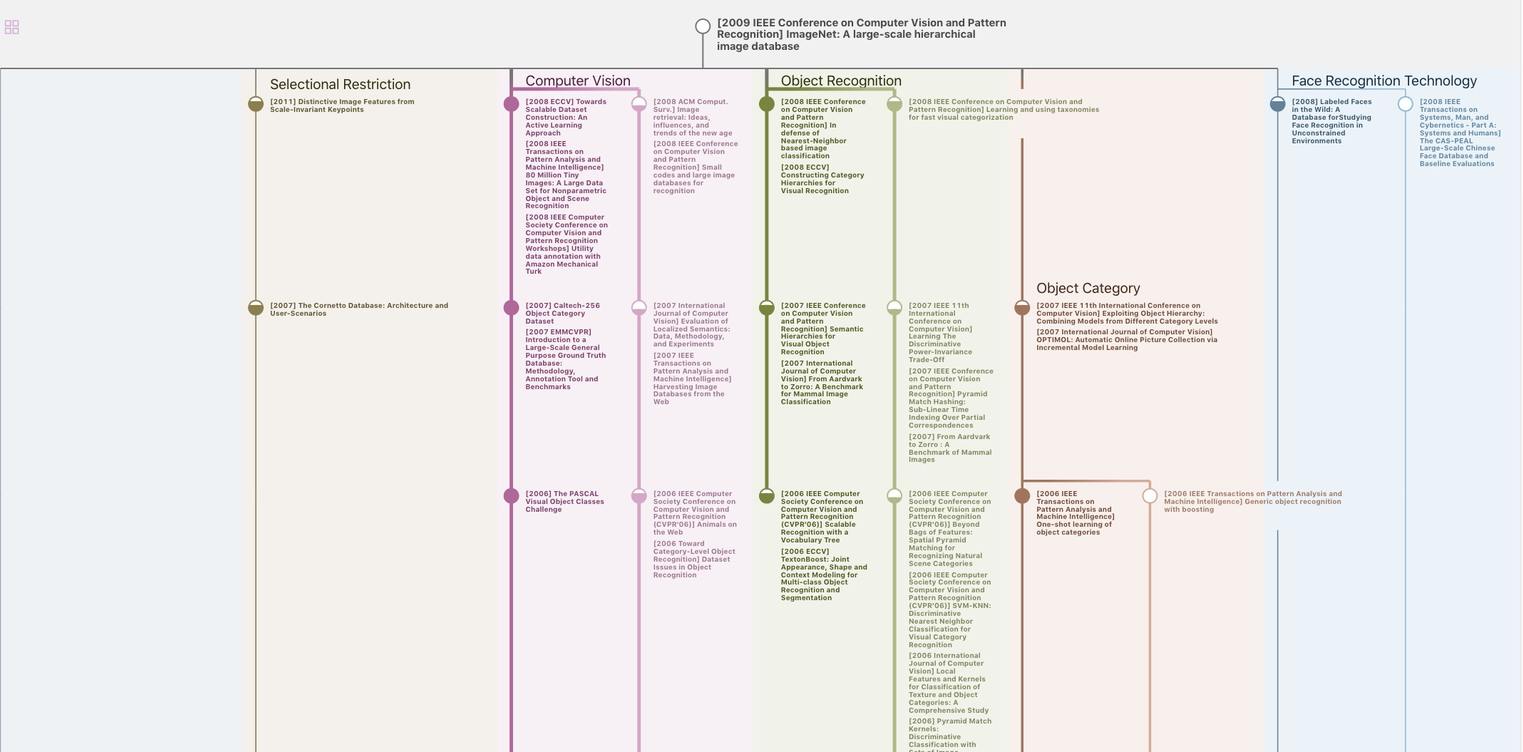
生成溯源树,研究论文发展脉络
Chat Paper
正在生成论文摘要