Handling Heavy Occlusion in Dense Crowd Tracking by Focusing on the Heads
ADVANCES IN ARTIFICIAL INTELLIGENCE, AI 2023, PT I(2024)
摘要
With the rapid development of deep learning, object detection and tracking play a vital role in today's society. Being able to identify and track all the pedestrians in the dense crowd scene with computer vision approaches is a typical challenge in this field, also known as the Multiple Object Tracking (MOT) challenge. Modern trackers are required to operate on more and more complicated scenes. According to the MOT20 challenge result, the pedestrian is 4 times denser than the MOT17 challenge. Hence, improving the ability to detect and track in extremely crowded scenes is the aim of this work. In light of the occlusion issue with the human body, the heads are usually easier to identify. In this work, we have designed a joint head and body detector in an anchor-free style to boost the detection recall and precision performance of pedestrians in both small and medium sizes. Innovatively, our model does not require information on the statistical head-body ratio for common pedestrians detection for training. Instead, the proposed model learns the ratio dynamically. To verify the effectiveness of the proposed model, we evaluate the model with extensive experiments on different datasets, including MOT20, Crowdhuman, and HT21 datasets. As a result, our proposed method significantly improves both the recall and precision rate on small and medium sized pedestrians, and achieves state-of-the-art results in these challenging datasets.
更多查看译文
关键词
Crowd,Detection,Tracking
AI 理解论文
溯源树
样例
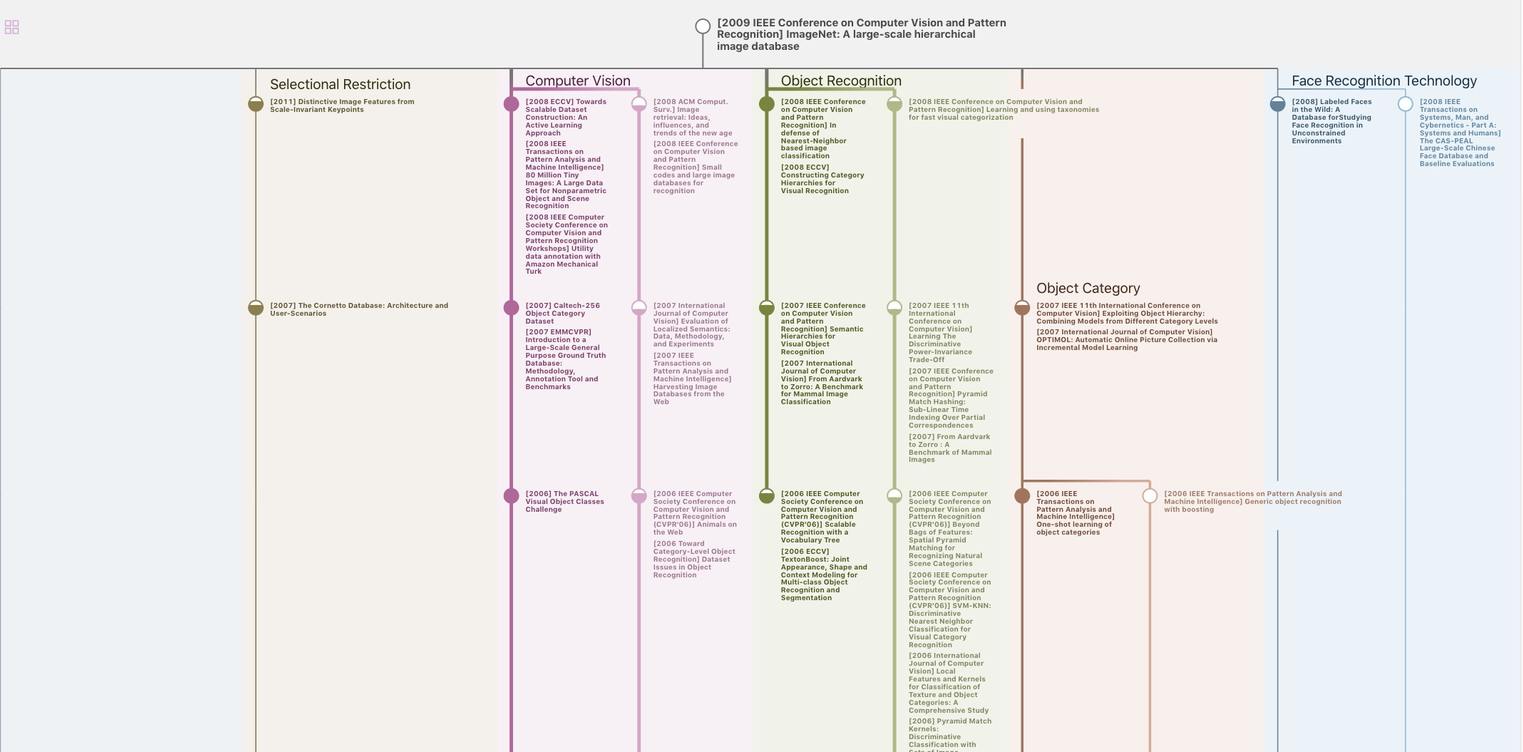
生成溯源树,研究论文发展脉络
Chat Paper
正在生成论文摘要