FedBlockHealth: A Synergistic Approach to Privacy and Security in IoT-Enabled Healthcare through Federated Learning and Blockchain
arXiv (Cornell University)(2023)
摘要
The rapid adoption of Internet of Things (IoT) devices in healthcare has introduced new challenges in preserving data privacy, security and patient safety. Traditional approaches need to ensure security and privacy while maintaining computational efficiency, particularly for resource-constrained IoT devices. This paper proposes a novel hybrid approach combining federated learning and blockchain technology to provide a secure and privacy-preserved solution for IoT-enabled healthcare applications. Our approach leverages a public-key cryptosystem that provides semantic security for local model updates, while blockchain technology ensures the integrity of these updates and enforces access control and accountability. The federated learning process enables a secure model aggregation without sharing sensitive patient data. We implement and evaluate our proposed framework using EMNIST datasets, demonstrating its effectiveness in preserving data privacy and security while maintaining computational efficiency. The results suggest that our hybrid approach can significantly enhance the development of secure and privacy-preserved IoT-enabled healthcare applications, offering a promising direction for future research in this field.
更多查看译文
关键词
federated learning,blockchain,privacy,iot-enabled
AI 理解论文
溯源树
样例
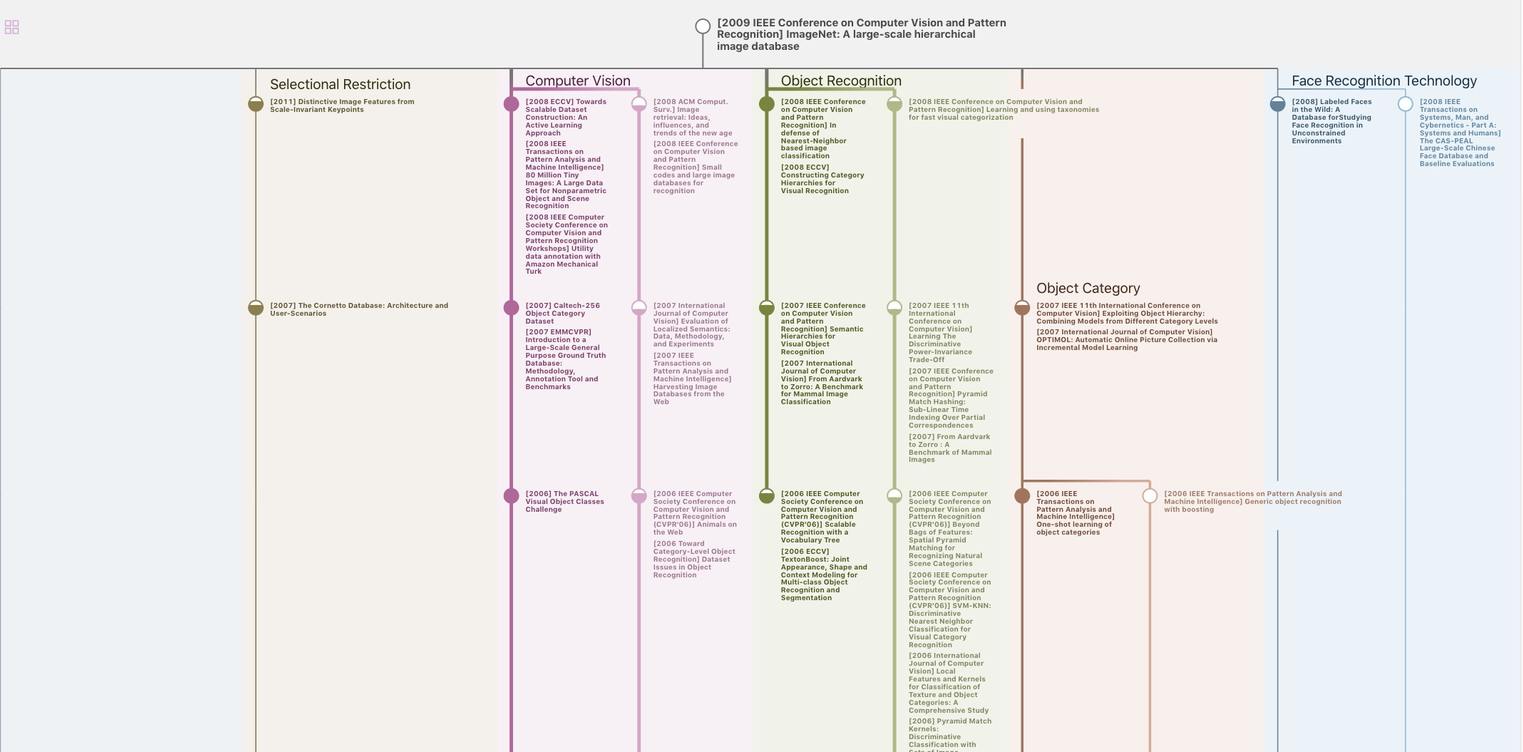
生成溯源树,研究论文发展脉络
Chat Paper
正在生成论文摘要