LASER: Neuro-Symbolic Learning of Semantic Video Representations
CoRR(2023)
摘要
Modern AI applications involving video, such as video-text alignment, video search, and video captioning, benefit from a fine-grained understanding of video semantics. Existing approaches for video understanding are either data-hungry and need low-level annotation, or are based on general embeddings that are uninterpretable and can miss important details. We propose LASER, a neuro-symbolic approach that learns semantic video representations by leveraging logic specifications that can capture rich spatial and temporal properties in video data. In particular, we formulate the problem in terms of alignment between raw videos and specifications. The alignment process efficiently trains low-level perception models to extract a fine-grained video representation that conforms to the desired high-level specification. Our pipeline can be trained end-to-end and can incorporate contrastive and semantic loss functions derived from specifications. We evaluate our method on two datasets with rich spatial and temporal specifications: 20BN-Something-Something and MUGEN. We demonstrate that our method not only learns fine-grained video semantics but also outperforms existing baselines on downstream tasks such as video retrieval.
更多查看译文
关键词
semantic video representations,learning,neuro-symbolic
AI 理解论文
溯源树
样例
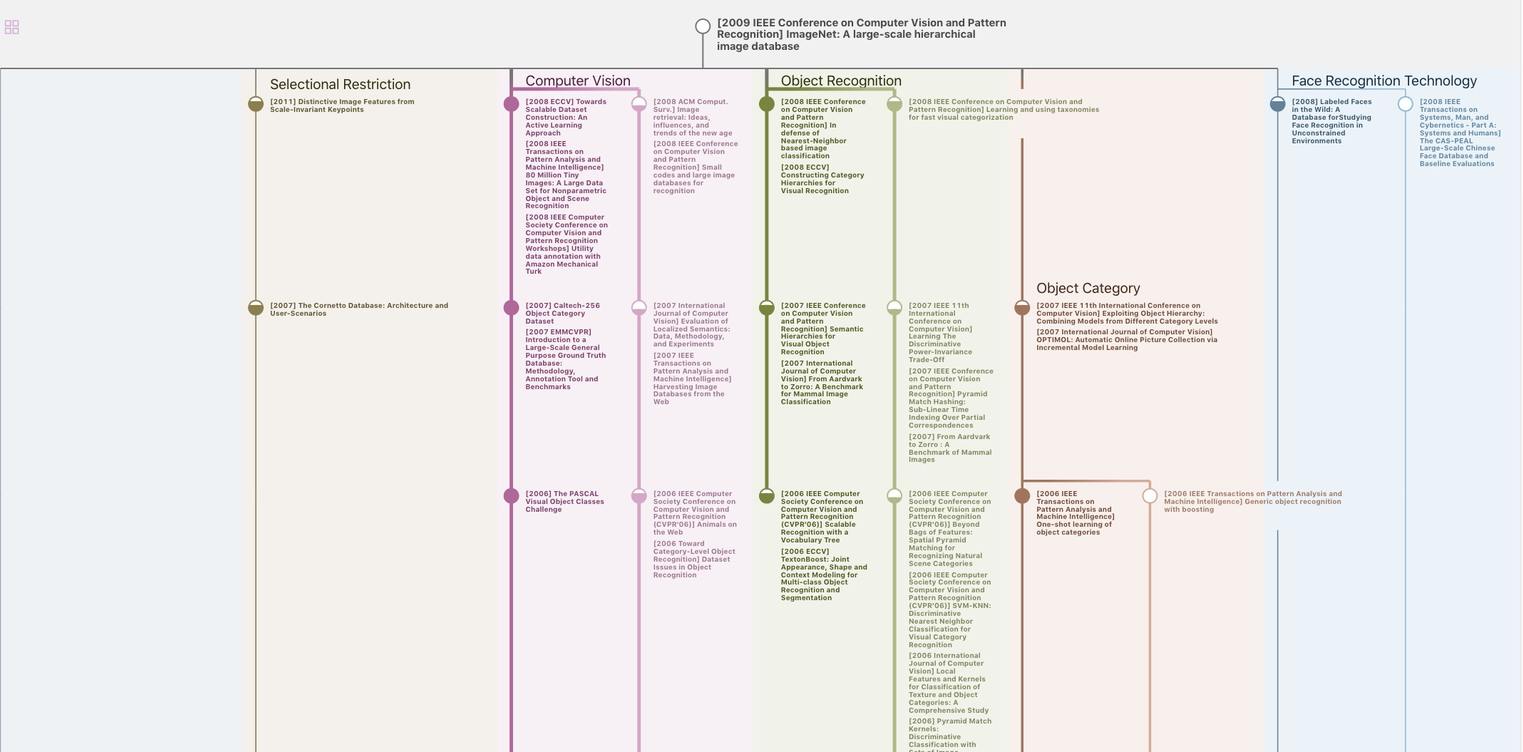
生成溯源树,研究论文发展脉络
Chat Paper
正在生成论文摘要