STen: Productive and Efficient Sparsity in PyTorch
CoRR(2023)
摘要
As deep learning models grow, sparsity is becoming an increasingly critical component of deep neural networks, enabling improved performance and reduced storage. However, existing frameworks offer poor support for sparsity. Specialized sparsity engines focus exclusively on sparse inference, while general frameworks primarily focus on sparse tensors in classical formats and neglect the broader sparsification pipeline necessary for using sparse models, especially during training. Further, existing frameworks are not easily extensible: adding a new sparse tensor format or operator is challenging and time-consuming. To address this, we propose STen, a sparsity programming model and interface for PyTorch, which incorporates sparsity layouts, operators, and sparsifiers, in an efficient, customizable, and extensible framework that supports virtually all sparsification methods. We demonstrate this by developing a high-performance grouped n:m sparsity layout for CPU inference at moderate sparsity. STen brings high performance and ease of use to the ML community, making sparsity easily accessible.
更多查看译文
关键词
efficient sparsity
AI 理解论文
溯源树
样例
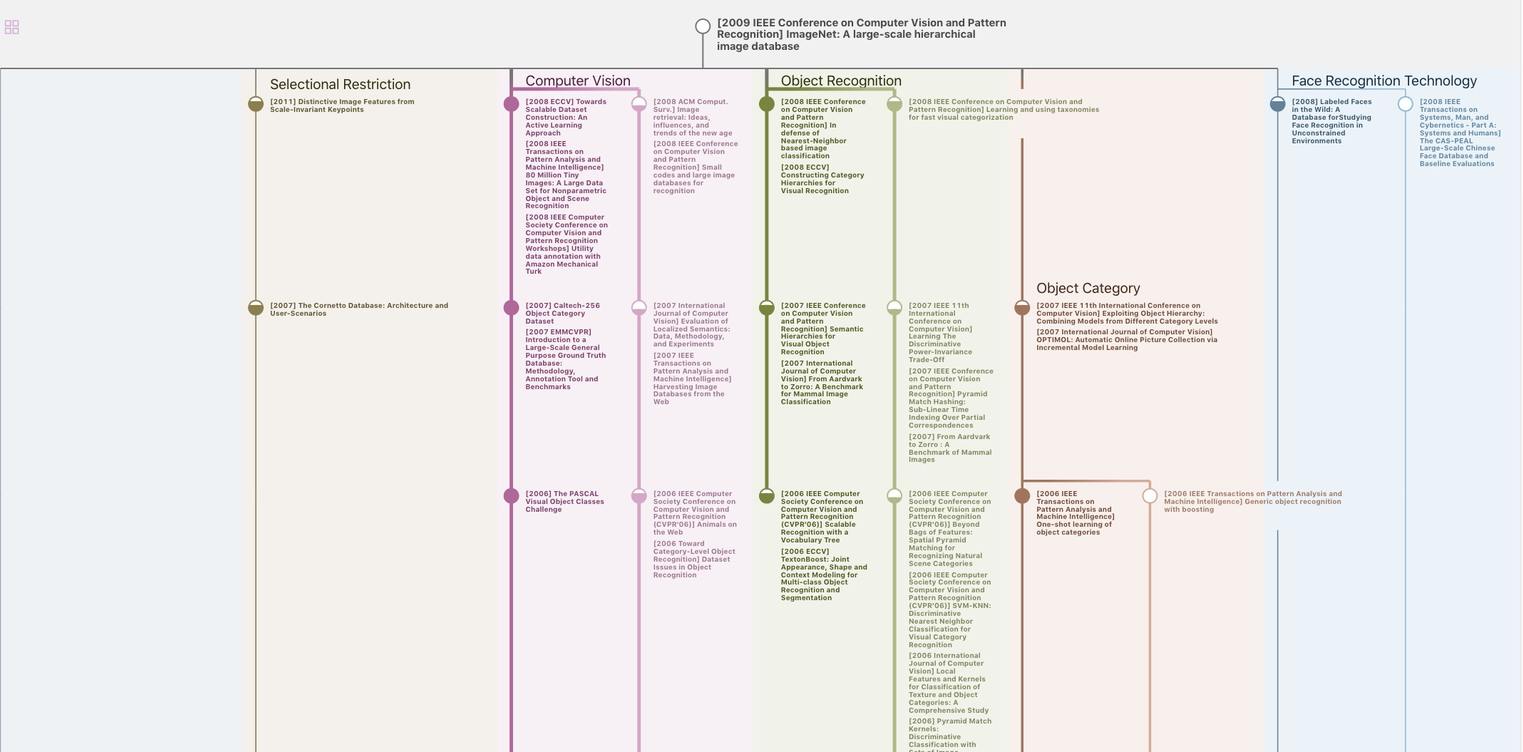
生成溯源树,研究论文发展脉络
Chat Paper
正在生成论文摘要