Welding seam detection and location: Deep learning network-based approach
International Journal of Pressure Vessels and Piping(2023)
摘要
There are many kinds of surface defects, large difference in size and strong randomness in the process of material welding, which makes it difficult for some conventional target detection algorithms to quickly and accurately locate and identify. This paper works out an improved Faster R–CNN network model. By adding FPN pyramid structure, variable convolution network and background suppression function to the traditional Faster R–CNN network, the path between the lower layer and the upper layer is greatly shortened, and the location information is better preserved. The simulation test shows that the model above improves the detection accuracy of the network, shortens the detection time, and reduces the loss value. The mAP is increased by 0.243, and the detection time of a single picture is shortened by 0.019s. The detection accuracy of five weld defects is significantly improved, and the accuracy of weld deviation is improved by 0.234, which is the one that improved the most significantly. It shows that the improved model is effective and has good convergence, and can meet the requirements of the detection of weld defects in the actual production process.
更多查看译文
关键词
Welding seam defect,Deep learning network,Detection,Faster R–CNN,FPN
AI 理解论文
溯源树
样例
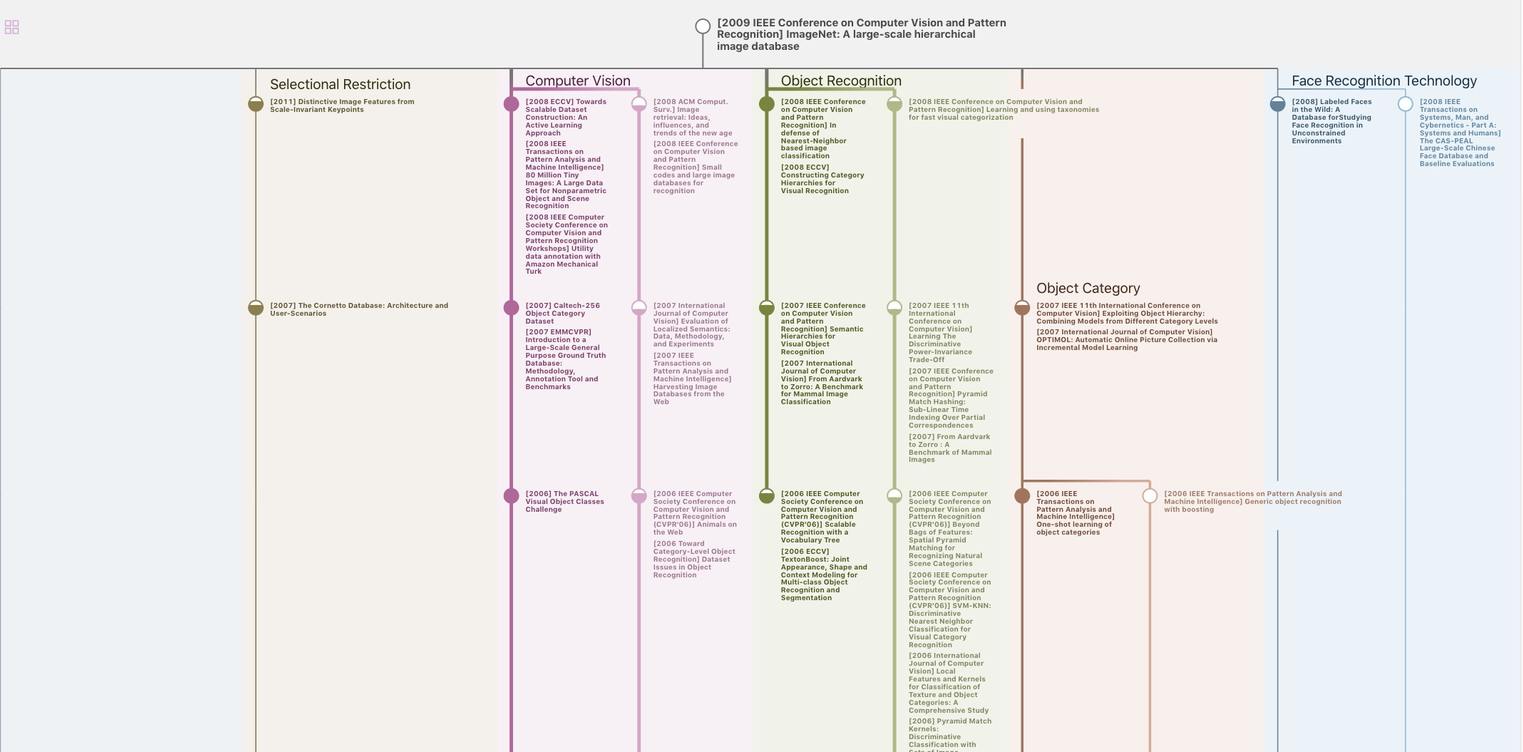
生成溯源树,研究论文发展脉络
Chat Paper
正在生成论文摘要